Multilayer Models Of Random Sequences: Representability And Inference Via Nonlinear Population Monte Carlo
CAMSAP(2019)
摘要
We investigate a class of dynamical models with a multilayer structure for the representation of discrete-time sequences. Each layer is a first-order, discrete-time Markov process, either on a discrete state space (i.e., a Markov chain) or on a general state space. The transition kernel for each layer is different (hence it yields different stochastic dynamics) and at each time there is a single active layer. The active layers are selected over time according to a first-order Markov chain. This simple description includes many types of interacting multiple models, of interest in target tracking applications. It also fits many real-world systems that display a variety of dynamical patterns over time without any observable switching mechanism (examples abound in financial time series). In this paper we show that the family of multilayer models described above can represent a broad class of random sequences, including Markov chains of order M > 1 on discrete spaces or auto-regressive process (again, of order M > 1) on general state spaces. We also propose a general nonlinear population Monte Carlo scheme that can be employed for model selection and model inference. Numerical examples are given for the case of multilayer models with discrete observations.
更多查看译文
关键词
Multilayer networks, random sequences, population Monte Carlo, Markov chains
AI 理解论文
溯源树
样例
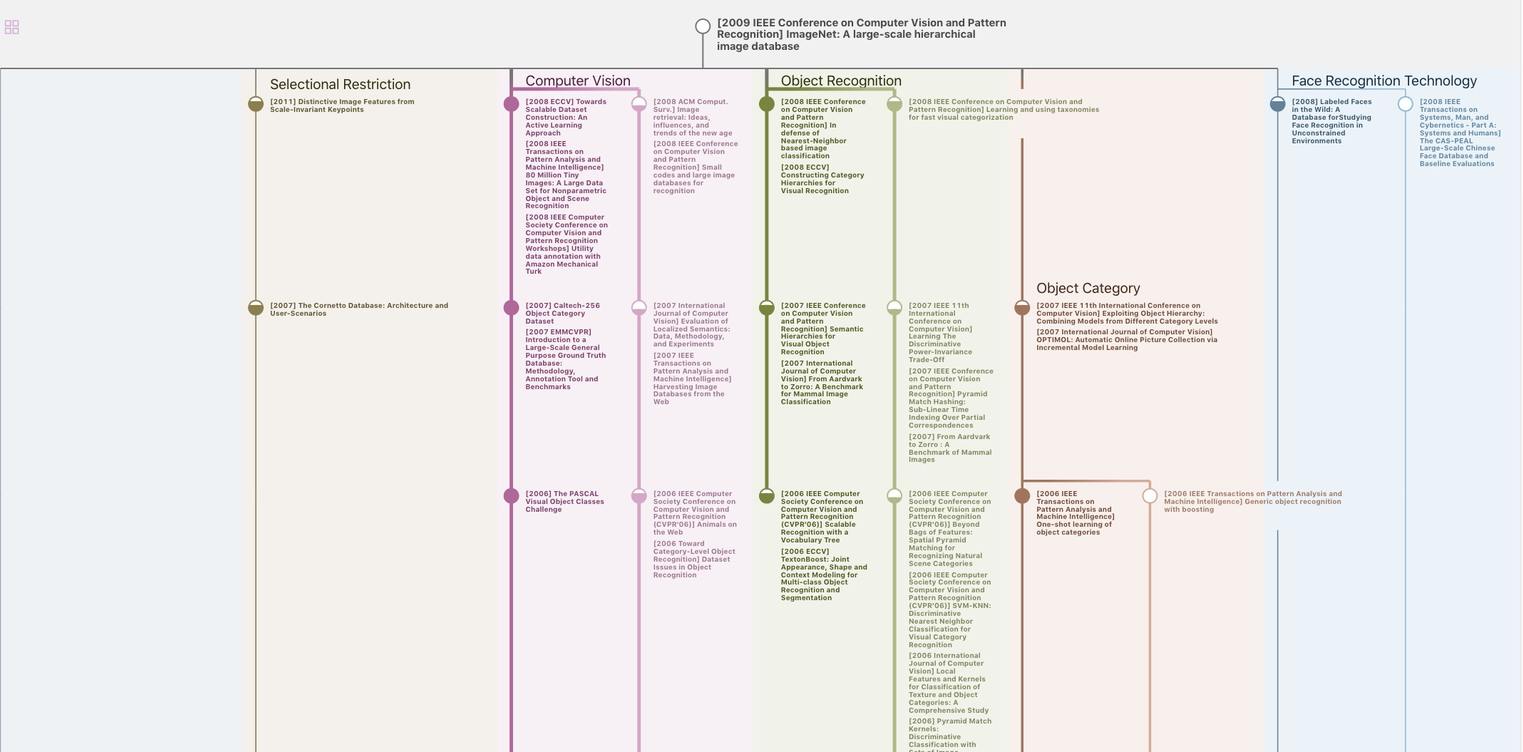
生成溯源树,研究论文发展脉络
Chat Paper
正在生成论文摘要