Multivariate Anomaly Detection in Mixed Telemetry Time-Series Using A Sparse Decomposition.
IEEE International Workshop on Computational Advances in Multi-Sensor Adaptive Processing(2019)
摘要
Spacecraft health monitoring from housekeeping telemetry data represents one of the main issues in space operations. Motivated by the success of machine learning or data driven-based methods in many signal and image processing applications, some of these methods have been applied to anomaly detection in housekeeping telemetry via a semi-supervised learning. This paper studies a new multivariate anomaly detection algorithm based on a sparse decomposition on a dictionary of nominal patterns. One originality of the proposed method is a multivariate framework allowing us to take into account possible relationships between different telemetry parameters, in particular through a joint processing of time-series described by mixed continuous and discrete parameters. The proposed method is tested with real satellite telemetry and evaluated on a representative anomaly dataset composed of actual anomalies that occurred on several operated satellites. The first results confirm the interest of the proposed method and demonstrate its competitiveness with respect to the state-of-the-art.
更多查看译文
关键词
spacecraft health monitoring,housekeeping telemetry data,space operations,machine learning,image processing applications,semisupervised learning,multivariate anomaly detection algorithm,sparse decomposition,nominal patterns,multivariate framework,mixed continuous parameters,discrete parameters,satellite telemetry,representative anomaly dataset,operated satellites,mixed telemetry time-series
AI 理解论文
溯源树
样例
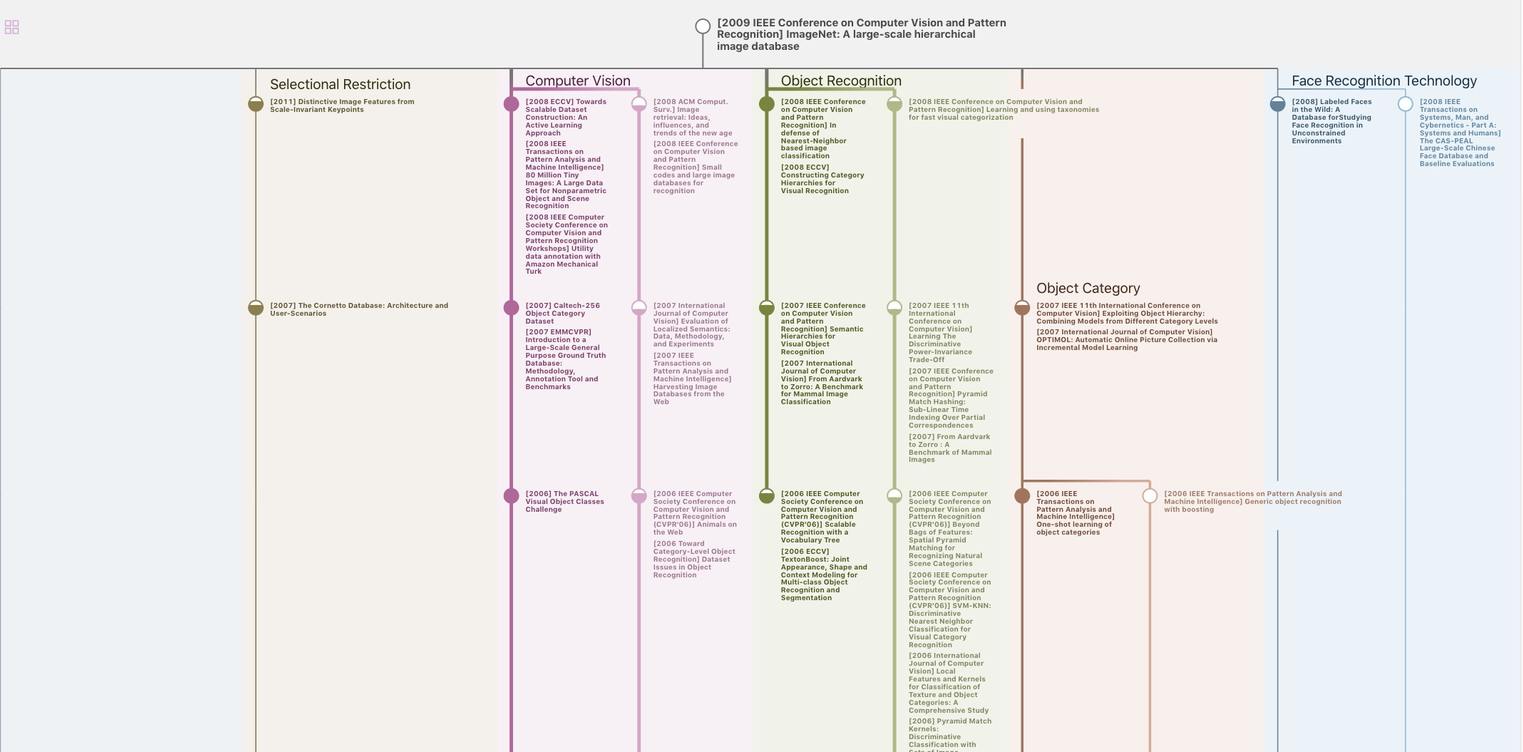
生成溯源树,研究论文发展脉络
Chat Paper
正在生成论文摘要