Phase Transitions in the Dynamic Mode Decomposition Algorithm
2019 IEEE 8th International Workshop on Computational Advances in Multi-Sensor Adaptive Processing (CAMSAP)(2019)
摘要
We analyze the Dynamic Mode Decomposition (DMD) algorithm in the noisy data setting. Previous work has shown that DMD is a source separation algorithm in disguise, i.e., that it is capable of unmixing linearly mixed time series. In this work, we analyze the performance of DMD when the mixed time series are corrupted by noise. We demonstrate that a preprocessing step of the truncated SVD before applying DMD yields significant benefits, and quantify the performance of the truncated-SVD-plus-DMD (tSVD-DMD) algorithm using tools from random matrix theory. We validate our findings with numerical simulations.
更多查看译文
关键词
Dynamic Mode Decomposition,Singular Value Decomposition,Random Matrix Theory,Source Separation,Time Series
AI 理解论文
溯源树
样例
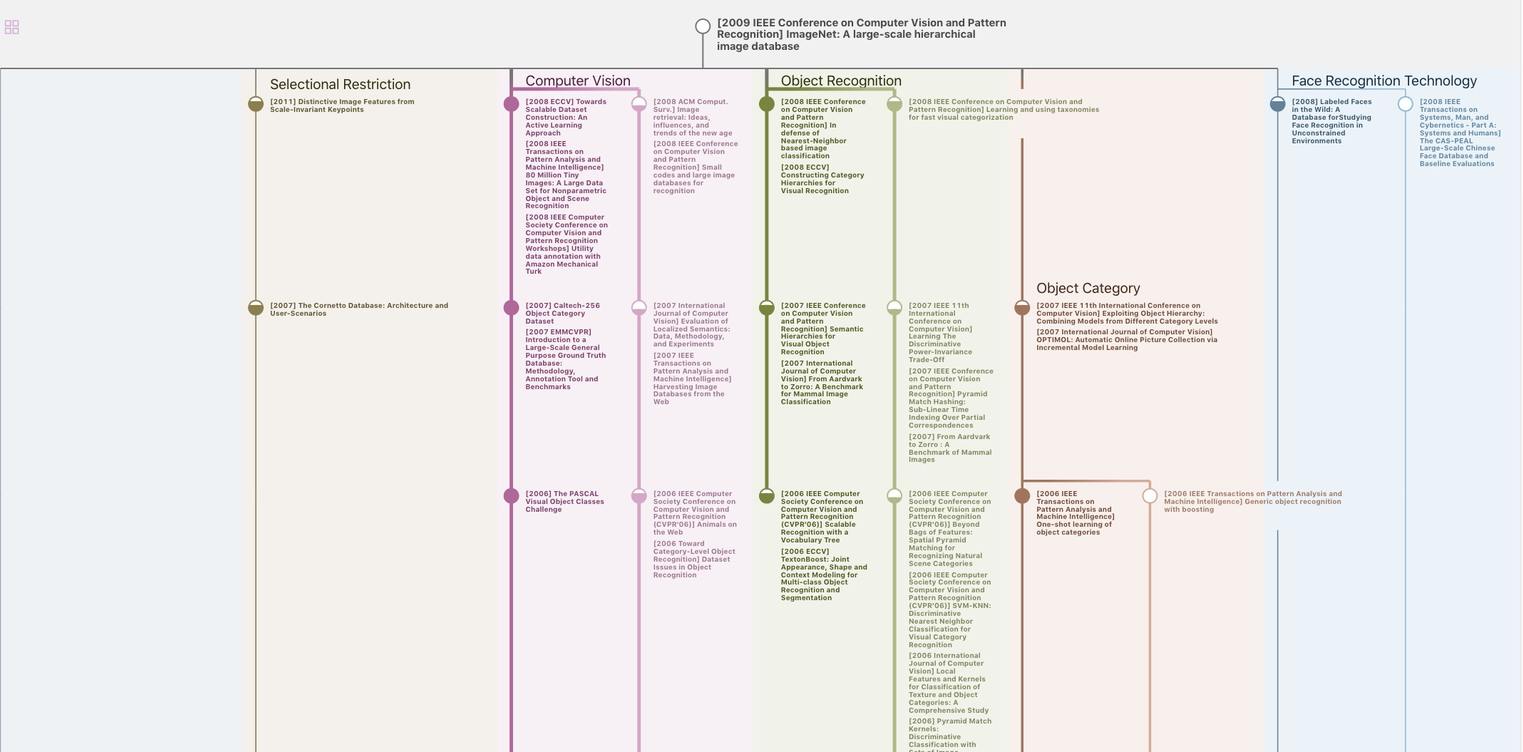
生成溯源树,研究论文发展脉络
Chat Paper
正在生成论文摘要