Multi-Agent Reinforcement Learning Based Energy Efficiency Optimization in NB-IoT Networks
2019 IEEE Globecom Workshops (GC Wkshps)(2019)
摘要
Based on the existing Evolved Packet System (EPS) architecture, Narrowband Internet of Things (NB-IoT) has been expected as a promising paradigm to support energy-aware massive Machine Type Communications (mMTC). However, with the tremendous increase of IoT devices, as well as their requirements of energy- saving and low-cost, current power ramping and preamble allocation mechanisms in legacy long term evolution (LTE) can hardly achieve high energy efficiency in machine-to-machine (M2M) communications, mainly resulting from the significant redundancy of control signals. Due to the strict restrictions of NB-IoT, up till the present moment, the standardized preamble allocation mechanism is still randomly picking. To satisfy these constrained conditions in NB-IoT, this work proposes a joint optimization framework of power ramping and preamble picking to improve the energy efficiency of NB-IoT systems. In this optimization problem, a comprehensive energy estimation model is established, which investigates the inadequacy of random access (RA) procedure and meanwhile reveals the effects of power ramping and preamble picking on energy efficiency. In addition, to search the optimal policies of the joint optimization formulated. A distributed Multi-Agent Reinforcement Learning (MARL) algorithm based on Win-or-Learn-Fast Policy Hill-Climbing (WoLF-PHC) is proposed, in which a "stateless" modification is introduced to reduce the algorithm complexity significantly. The performance of high energy efficiency is validated in simulations, which also reveal the applicability and convergence of the designed WoLF-PHC based optimization algorithm.
更多查看译文
关键词
Evolved Packet System architecture,distributed MultiAgent Reinforcement Learning,optimal policies,preamble picking,comprehensive energy estimation model,optimization problem,NB-IoT systems,joint optimization framework,standardized preamble allocation mechanism,machine-to-machine communications,high energy efficiency,legacy long term evolution,current power ramping,IoT devices,energy efficiency optimization,NB-IoT networks,energy-aware massive Machine Type Communications
AI 理解论文
溯源树
样例
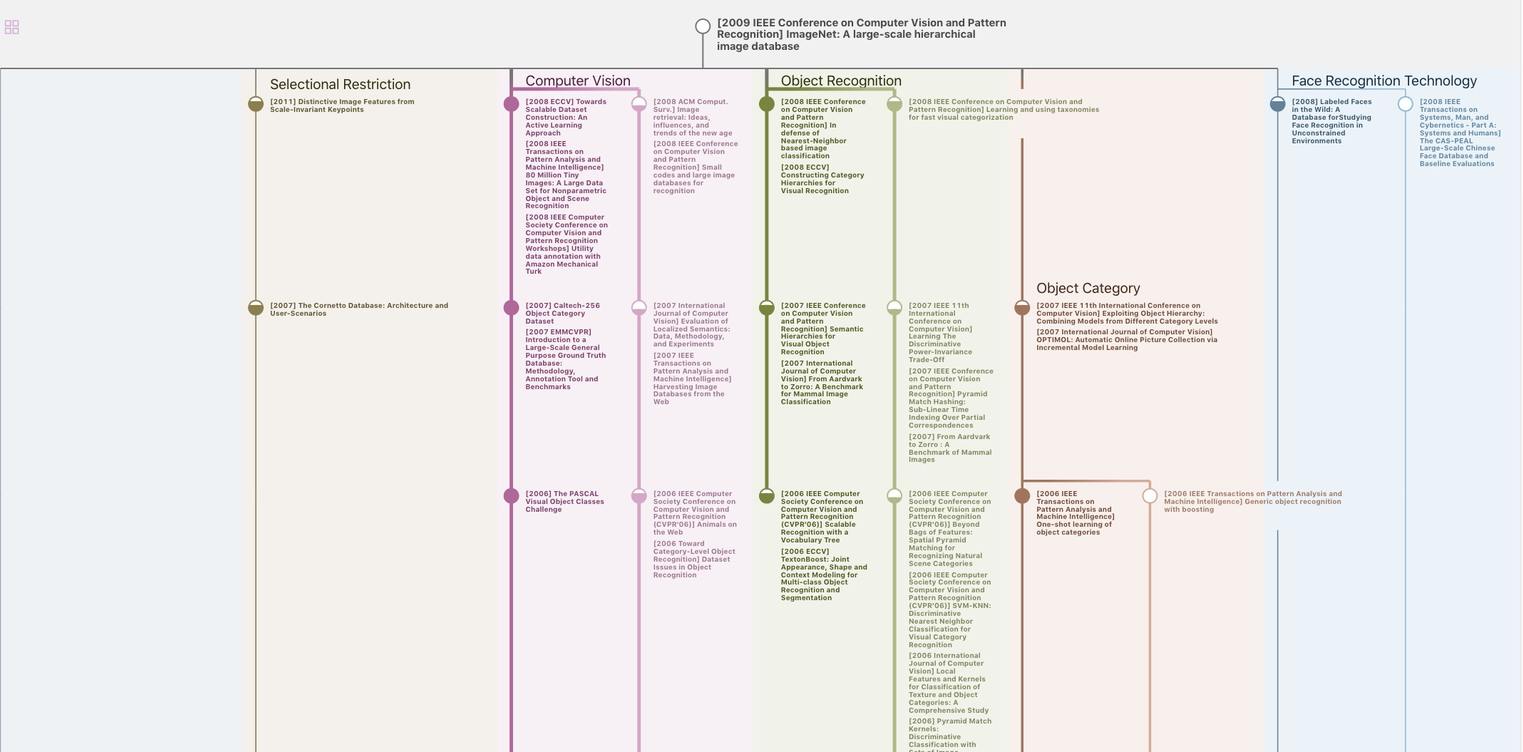
生成溯源树,研究论文发展脉络
Chat Paper
正在生成论文摘要