KQIs-Driven QoE Anomaly Detection and Root Cause Analysis in Cellular Networks.
GLOBECOM Workshops(2019)
摘要
With the dense deployment of cellular networks, the enrichment of service types and the explosive growth of data volume, there is an increasing need of automatically detecting and diagnosing degraded performance cells for operators. Previous studies mainly focus on QoS (Quality of Service) based anomaly detection and root cause analysis, but the cellular network operators pay more attention to the user’s QoE (Quality of Experience) degradation since QoE can better represent the user’s actual service experience. To achieve online QoE anomaly detection and root cause analysis, in this paper, we present a new integrated anomaly detection and diagnosis framework. Firstly, QoE is expressed by the KQIs (Key Quality Indicators) vector of the user’s three most common services with largest traffic. And then, QoE anomaly detection is processed by using two-step clustering method (SOM+K-mediods) to obtain QoE Anomaly Codebooks. Diagnosis is achieved by selecting KPIs (Key Performance Indicators) and learning their characteristic impact on KQIs to obtain Fault Cause Codebooks. The QoE Anomaly Codebooks are used to detect anomaly samples, and the Fault Cause Codebooks are applied to find the fault cause types. The designed framework has been evaluated with real 4G data. For comparison, three other two- step clustering algorithms are introduced, and results show that our framework can achieve the highest detection accuracy rate. Therefore, it can be directly used in real-world cellular networks for online fault detection and diagnosis.
更多查看译文
关键词
On-line Detection, Diagnosis, SOM plus K-mediods, Self-organizing networks (SONs), QoE
AI 理解论文
溯源树
样例
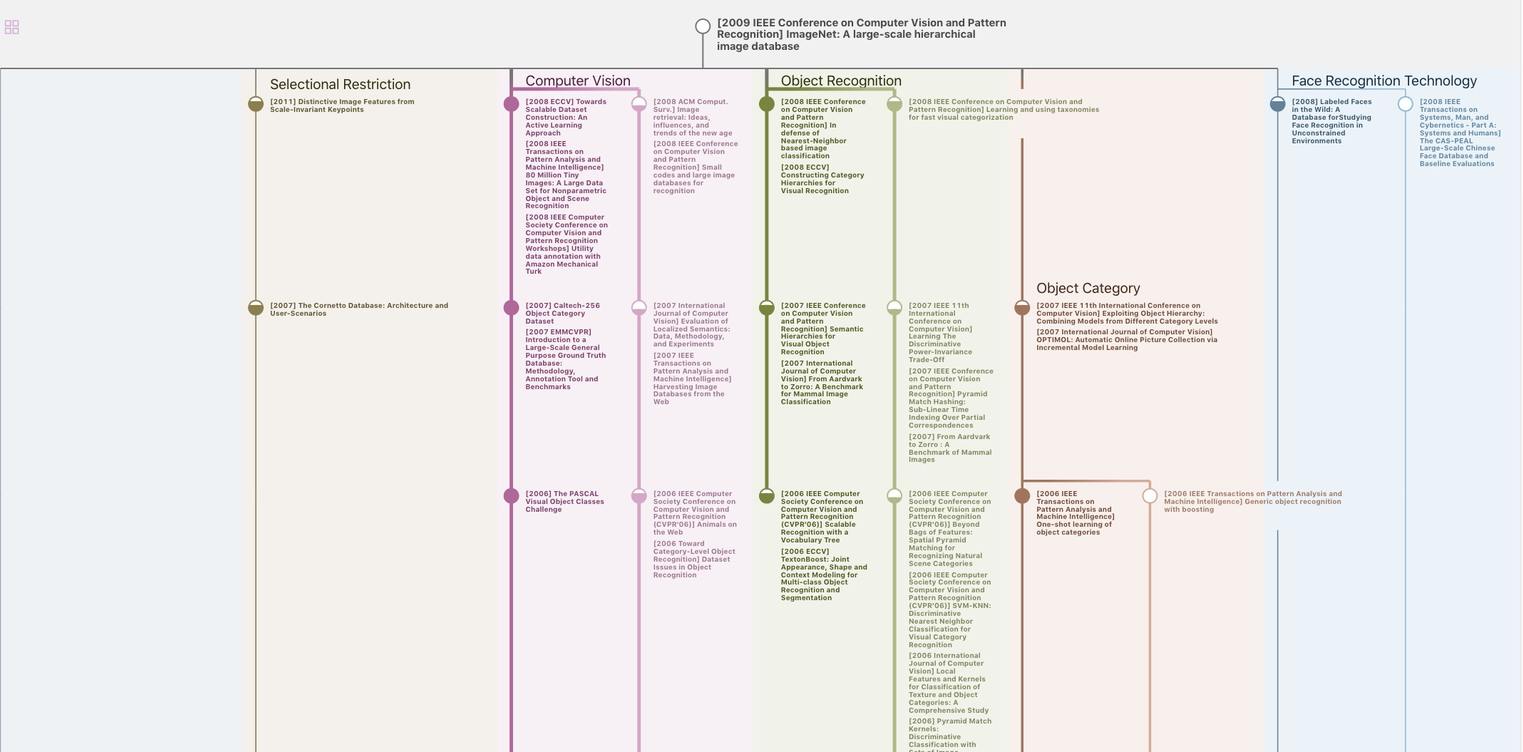
生成溯源树,研究论文发展脉络
Chat Paper
正在生成论文摘要