End-To-End Learning Local Multi-View Descriptors For 3d Point Clouds
2020 IEEE/CVF CONFERENCE ON COMPUTER VISION AND PATTERN RECOGNITION (CVPR)(2020)
摘要
In this work, we propose an end-to-end framework to learn local multi-view descriptors for 3D point clouds. To adopt a similar multi-view representation, existing studies use hand-crafted viewpoints for rendering in a preprocessing stage, which is detached from the subsequent descriptor learning stage. In our framework, we integrate the multiview rendering into neural networks by using a differentiable renderer, which allows the viewpoints to be optimizable parameters for capturing more informative local context of interest points. To obtain discriminative descriptors, we also design a soft-view pooling module to attentively fuse convolutional features across views. Extensive experiments on existing 3D registration benchmarks show that our method outperforms existing local descriptors both quantitatively and qualitatively.
更多查看译文
关键词
3d,end-to-end,multi-view
AI 理解论文
溯源树
样例
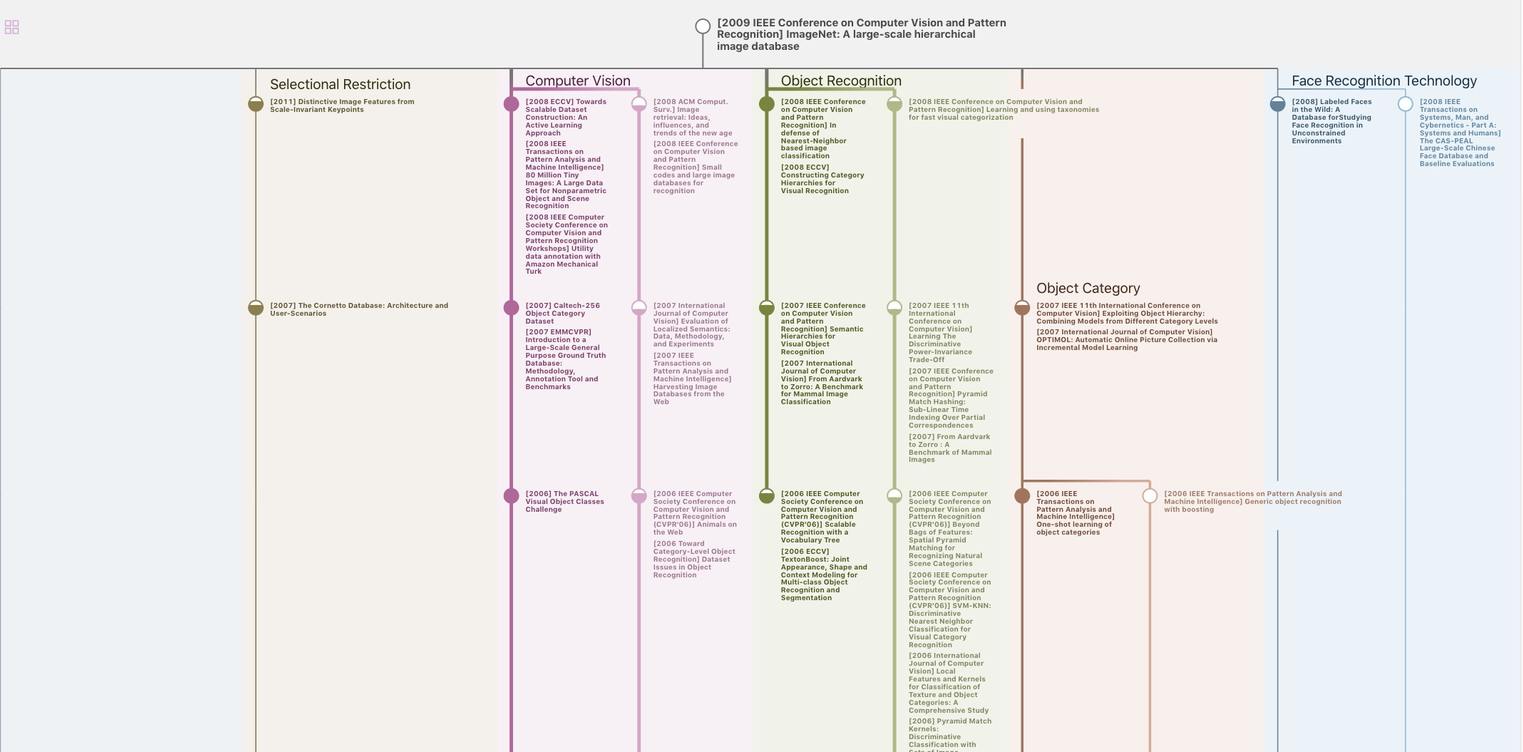
生成溯源树,研究论文发展脉络
Chat Paper
正在生成论文摘要