Robust Drift Characterization from Event Streams of Business Processes
ACM Transactions on Knowledge Discovery from Data(2020)
摘要
Process workers may vary the normal execution of a business process to adjust to changes in their operational environment, e.g., changes in workload, season, or regulations. Changes may be simple, such as skipping an individual activity, or complex, such as replacing an entire procedure with another. Over time, these changes may negatively affect process performance; hence, it is important to identify and understand them early on. As such, a number of techniques have been developed to detect process drifts, i.e., statistically significant changes in process behavior, from process event logs (offline) or event streams (online). However, detecting a drift without characterizing it, i.e., without providing explanations on its nature, is not enough to help analysts understand and rectify root causes for process performance issues. Existing approaches for drift characterization are limited to simple changes that affect individual activities. This article contributes an efficient, accurate, and noise-tolerant automated method for characterizing complex drifts affecting entire process fragments. The method, which works both offline and online, relies on two cornerstone techniques, one to automatically discover process trees from event streams (logs) and the other to transform process trees using a minimum number of change operations. The operations identified are then translated into natural language statements to explain the change behind a drift. The method has been extensively evaluated on artificial and real-life datasets, and against a state-of-the-art baseline method. The results from one of the real-life datasets have also been validated with a process stakeholder.
更多查看译文
关键词
Concept drift,business process,business process management,drift characterization,process drift,process mining
AI 理解论文
溯源树
样例
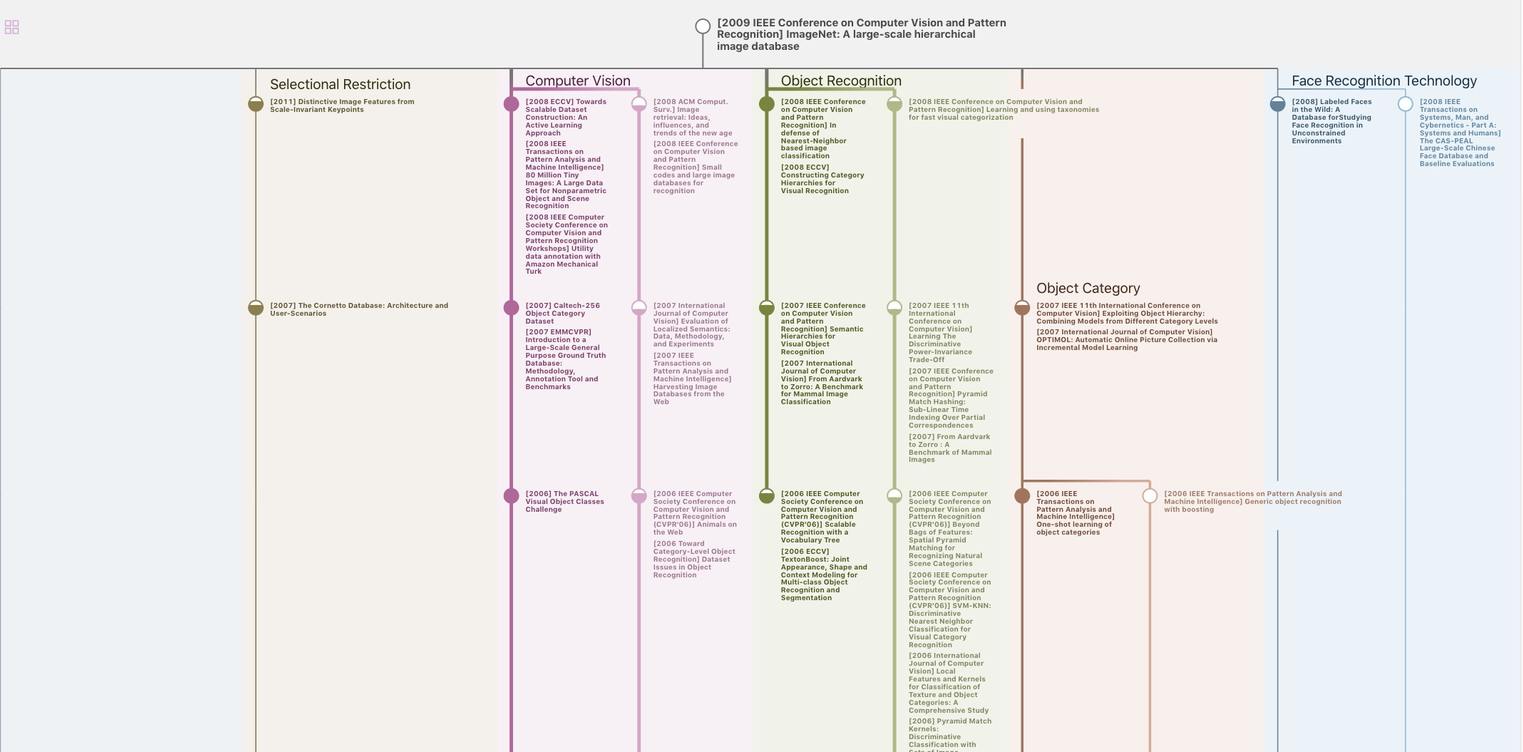
生成溯源树,研究论文发展脉络
Chat Paper
正在生成论文摘要