General principles of machine learning for brain-computer interfacing.
Handbook of clinical neurology(2020)
摘要
Brain-computer interfaces (BCIs) are systems that translate brain activity patterns into commands that can be executed by an artificial device. This enables the possibility of controlling devices such as a prosthetic arm or exoskeleton, a wheelchair, typewriting applications, or games directly by modulating our brain activity. For this purpose, BCI systems rely on signal processing and machine learning algorithms to decode the brain activity. This chapter provides an overview of the main steps required to do such a process, including signal preprocessing, feature extraction and selection, and decoding. Given the large amount of possible methods that can be used for these processes, a comprehensive review of them is beyond the scope of this chapter, and it is focused instead on the general principles that should be taken into account, as well as discussing good practices on how these methods should be applied and evaluated for proper design of reliable BCI systems.
更多查看译文
关键词
Artifacts,Brain-computer interface,Brain–machine interface,Classification,Cross-validation,Features,Filtering,Information transfer rate,Machine learning,Performance evaluation,Regression
AI 理解论文
溯源树
样例
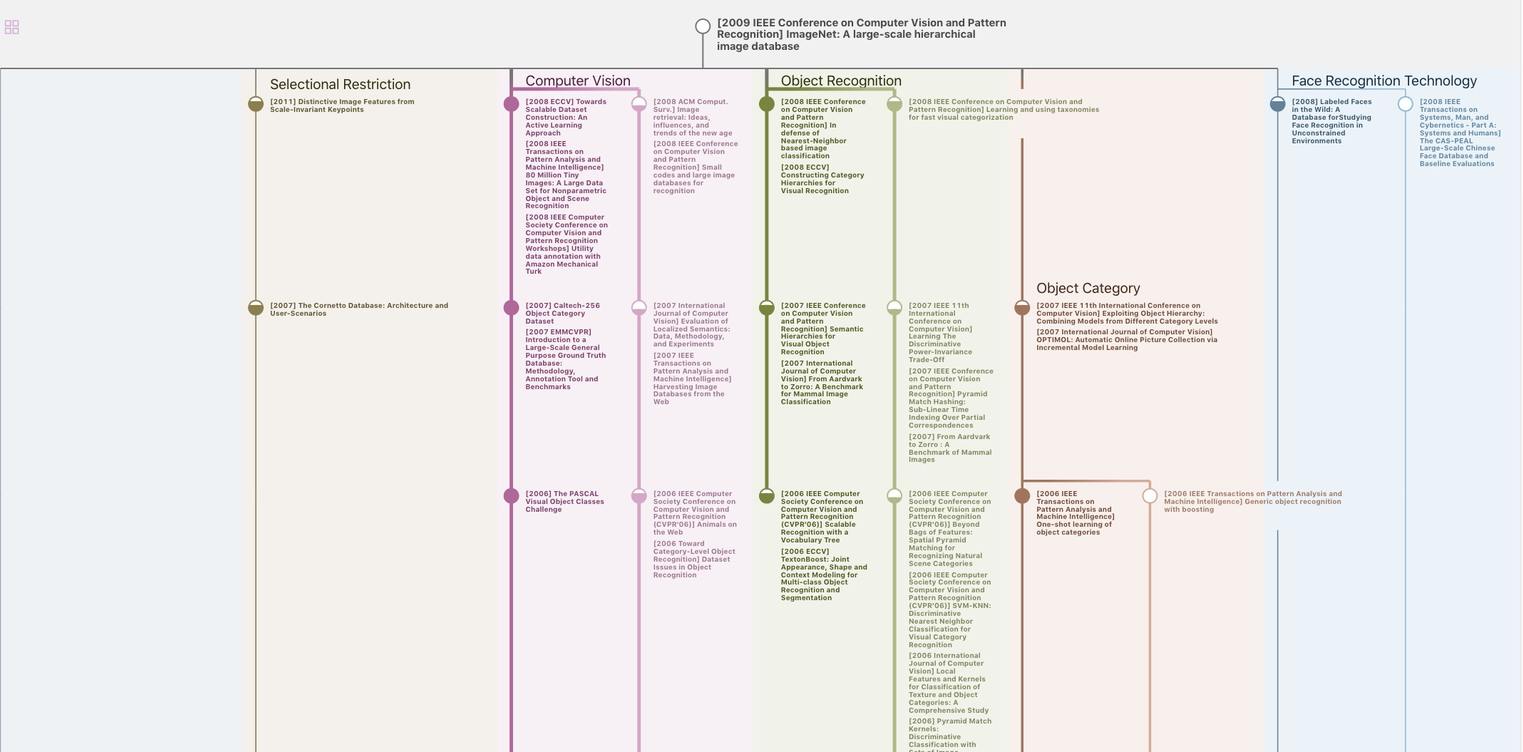
生成溯源树,研究论文发展脉络
Chat Paper
正在生成论文摘要