A Study on Acoustic Parameter Selection Strategies to Improve Deep Learning-Based Speech Synthesis
2019 Asia-Pacific Signal and Information Processing Association Annual Summit and Conference (APSIPA ASC)(2019)
摘要
In this paper, we investigate the variation in the performance of a deep learning-based speech synthesis (DLSS) system based on the configuration of output acoustic parameters. Our method is mainly applicable for vocoding-based statistical parametric speech synthesis (SPSS), which has advantages in low-resource scenarios. Given the independence assumption of the source-filter model for the spectral and fundamental frequency F0 parameters, we propose a reliable network architecture for training acoustic parameters. Particularly, the F0 parameter suffers from high fluctuation and an extremely low number of dimensions. To relieve these problems, we introduce a context-window approach. Furthermore, we apply data augmentation to the proposed structure to overcome a lack of training data, which is a frequent issue with multi-speaker TTS systems. Experimental results confirm the superiority of the proposed algorithm over conventional ones in both single-speaker and multi-speaker TTS setups.
更多查看译文
关键词
vocoding-based statistical parametric speech synthesis,source-filter model,spectral frequency parameters,fundamental frequency parameters,reliable network architecture,F0 parameter suffers,multispeaker TTS systems,acoustic parameter selection strategies,deep learning-based speech synthesis system
AI 理解论文
溯源树
样例
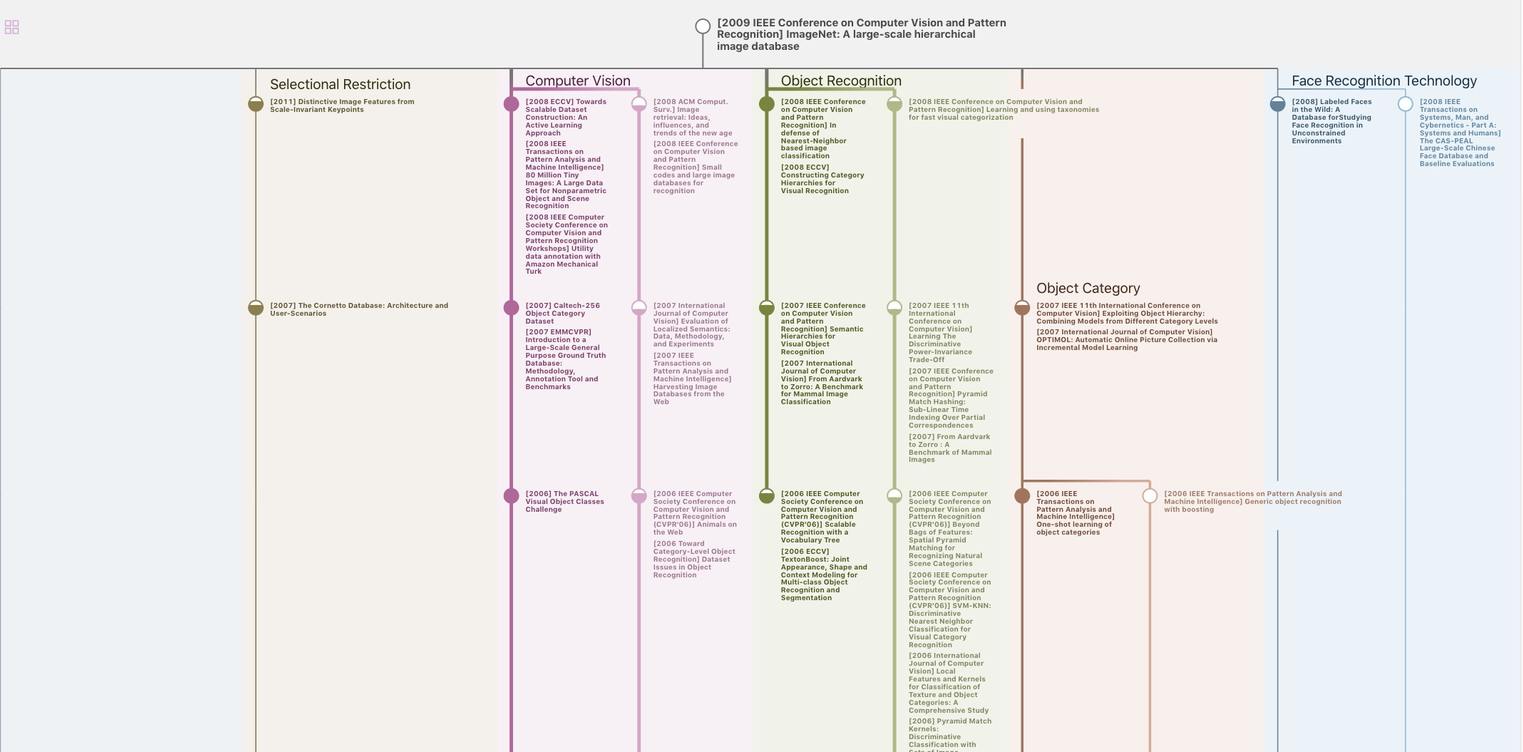
生成溯源树,研究论文发展脉络
Chat Paper
正在生成论文摘要