When are Non-Parametric Methods Robust?
ICML(2020)
摘要
A growing body of research has shown that many classifiers are susceptible to {\em{adversarial examples}} -- small strategic modifications to test inputs that lead to misclassification. In this work, we study general non-parametric methods, with a view towards understanding when they are robust to these modifications. We establish general conditions under which non-parametric methods are r-consistent -- in the sense that they converge to optimally robust and accurate classifiers in the large sample limit. Concretely, our results show that when data is well-separated, nearest neighbors and kernel classifiers are r-consistent, while histograms are not. For general data distributions, we prove that preprocessing by Adversarial Pruning (Yang et. al., 2019) -- that makes data well-separated -- followed by nearest neighbors or kernel classifiers also leads to r-consistency.
更多查看译文
关键词
robust,methods,non-parametric
AI 理解论文
溯源树
样例
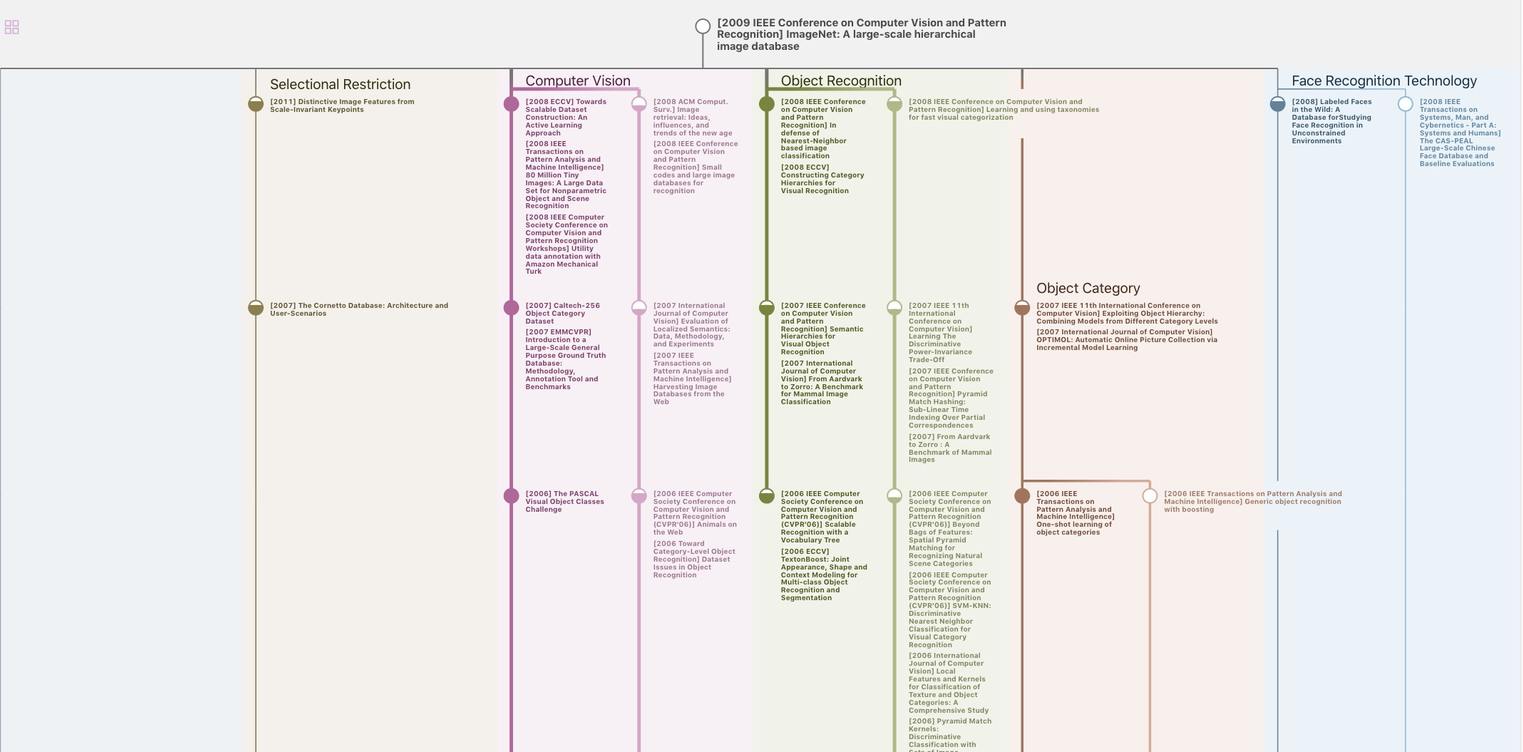
生成溯源树,研究论文发展脉络
Chat Paper
正在生成论文摘要