Well-posedness of the Deterministic Transport Equation with Singular Velocity Field Perturbed along Fractional Brownian Paths
Journal of Differential Equations(2023)SCI 2区
Abstract
In this article we prove path-by-path uniqueness in the sense of Davie \cite{Davie07} and Shaposhnikov \cite{Shaposhnikov16} for SDE's driven by a fractional Brownian motion with a Hurst parameter $H\in(0,\frac{1}{2})$, uniformly in the initial conditions, where the drift vector field is allowed to be merely bounded and measurable.\par Using this result, we construct weak unique regular solutions in $W_{loc}^{k,p}\left([0,1]\times\mathbb{R}^d\right)$, $p>d$ of the classical transport and continuity equations with singular velocity fields perturbed along fractional Brownian paths.\par The latter results provide a systematic way of producing examples of singular velocity fields, which cannot be treated by the regularity theory of DiPerna-Lyons \cite{DiPernaLions89}, Ambrosio \cite{Ambrosio04} or Crippa-De Lellis \cite{CrippaDeLellis08}.\par Our approach is based on a priori estimates at the level of flows generated by a sequence of mollified vector fields, converging to the original vector field, and which are uniform with respect to the mollification parameter. In addition, we use a compactness criterion based on Malliavin calculus from \cite{DMN92} as well as supremum concentration inequalities. \emph{keywords}: Transport equation, Compactness criterion, Singular vector fields, Regularization by noise.
MoreTranslated text
Key words
Transport equation,Compactness criterion,Singular vector fields,Regularization by noise
PDF
View via Publisher
AI Read Science
AI Summary
AI Summary is the key point extracted automatically understanding the full text of the paper, including the background, methods, results, conclusions, icons and other key content, so that you can get the outline of the paper at a glance.
Example
Background
Key content
Introduction
Methods
Results
Related work
Fund
Key content
- Pretraining has recently greatly promoted the development of natural language processing (NLP)
- We show that M6 outperforms the baselines in multimodal downstream tasks, and the large M6 with 10 parameters can reach a better performance
- We propose a method called M6 that is able to process information of multiple modalities and perform both single-modal and cross-modal understanding and generation
- The model is scaled to large model with 10 billion parameters with sophisticated deployment, and the 10 -parameter M6-large is the largest pretrained model in Chinese
- Experimental results show that our proposed M6 outperforms the baseline in a number of downstream tasks concerning both single modality and multiple modalities We will continue the pretraining of extremely large models by increasing data to explore the limit of its performance
Try using models to generate summary,it takes about 60s
Must-Reading Tree
Example
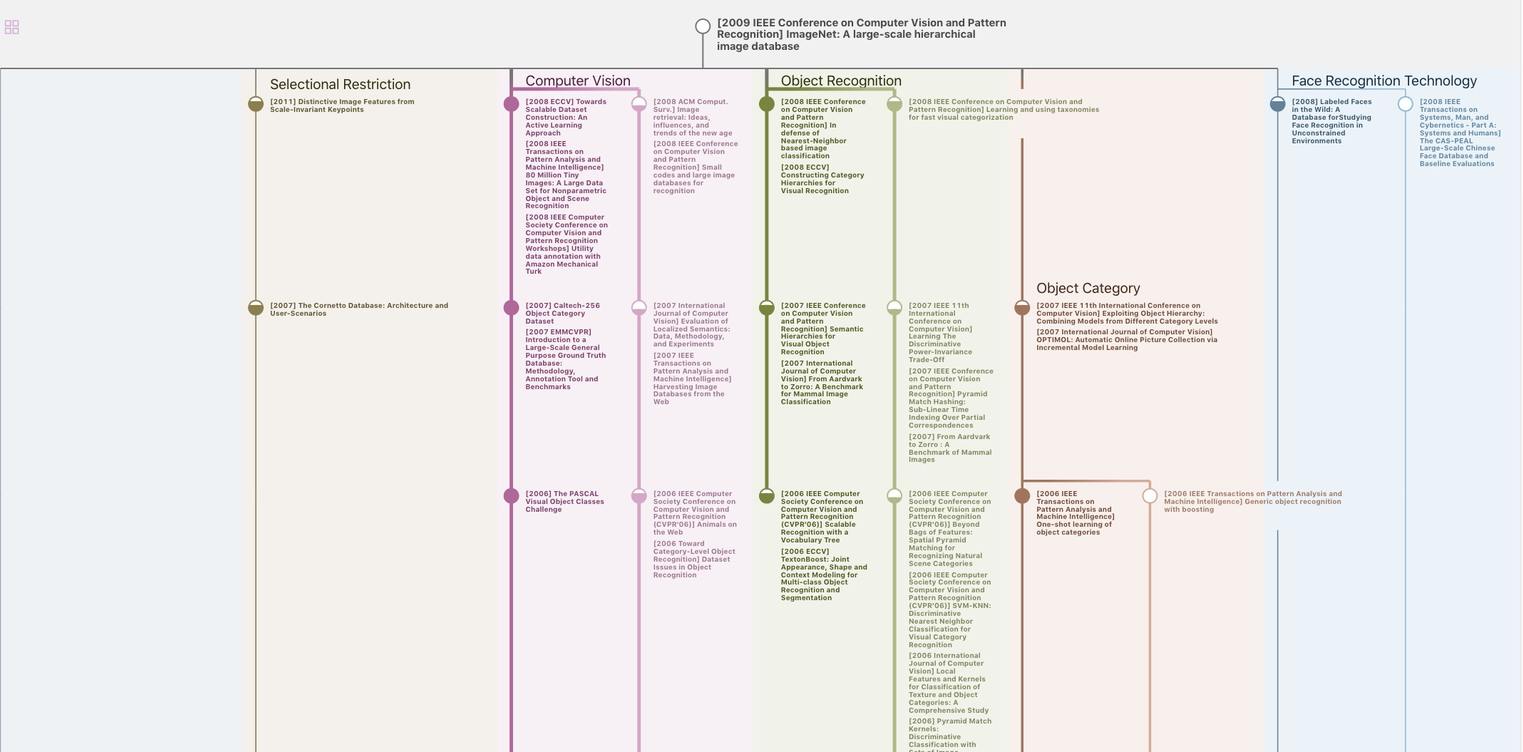
Generate MRT to find the research sequence of this paper
Related Papers
Path-by-path Uniqueness of Multidimensional SDE’s on the Plane with Nondecreasing Coefficients
Electronic Journal of Probability 2022
被引用3
ALEA-LATIN AMERICAN JOURNAL OF PROBABILITY AND MATHEMATICAL STATISTICS 2023
被引用2
arXiv (Cornell University) 2022
被引用4
Data Disclaimer
The page data are from open Internet sources, cooperative publishers and automatic analysis results through AI technology. We do not make any commitments and guarantees for the validity, accuracy, correctness, reliability, completeness and timeliness of the page data. If you have any questions, please contact us by email: report@aminer.cn
Chat Paper
GPU is busy, summary generation fails
Rerequest