Combining analytic methods to unlock sequential and temporal patterns of self-regulated learning.
LAK '20: 10th International Conference on Learning Analytics and Knowledge Frankfurt Germany March, 2020(2020)
摘要
The temporal and sequential nature of learning is receiving increasing focus in Learning Analytics circles. The desire to embed studies in recognised theories of self-regulated learning (SRL) has led researchers to conceptualise learning as a process that unfolds and changes over time. To that end, a body of research knowledge is growing which states that traditional frequency-based correlational studies are limited in narrative impact. To further explore this, we analysed trace data collected from online activities of a sample of 239 computer engineering undergraduate students enrolled on a course that followed a flipped class-room pedagogy. We employed SRL categorisation of micro-level processes based on a recognised model of learning, and then analysed the data using: 1) simple frequency measures; 2) epistemic network analysis; 3) temporal process mining; and 4) stochastic process mining. We found that a combination of analyses provided us with a richer insight into SRL behaviours than any one single method. We found that better performing learners employed more optimal behaviours in their navigation through the course's learning management system.
更多查看译文
关键词
Learning Analytics,Self-regulated Learning,Micro-level Processes,Epistemic Network Analysis,Process Mining
AI 理解论文
溯源树
样例
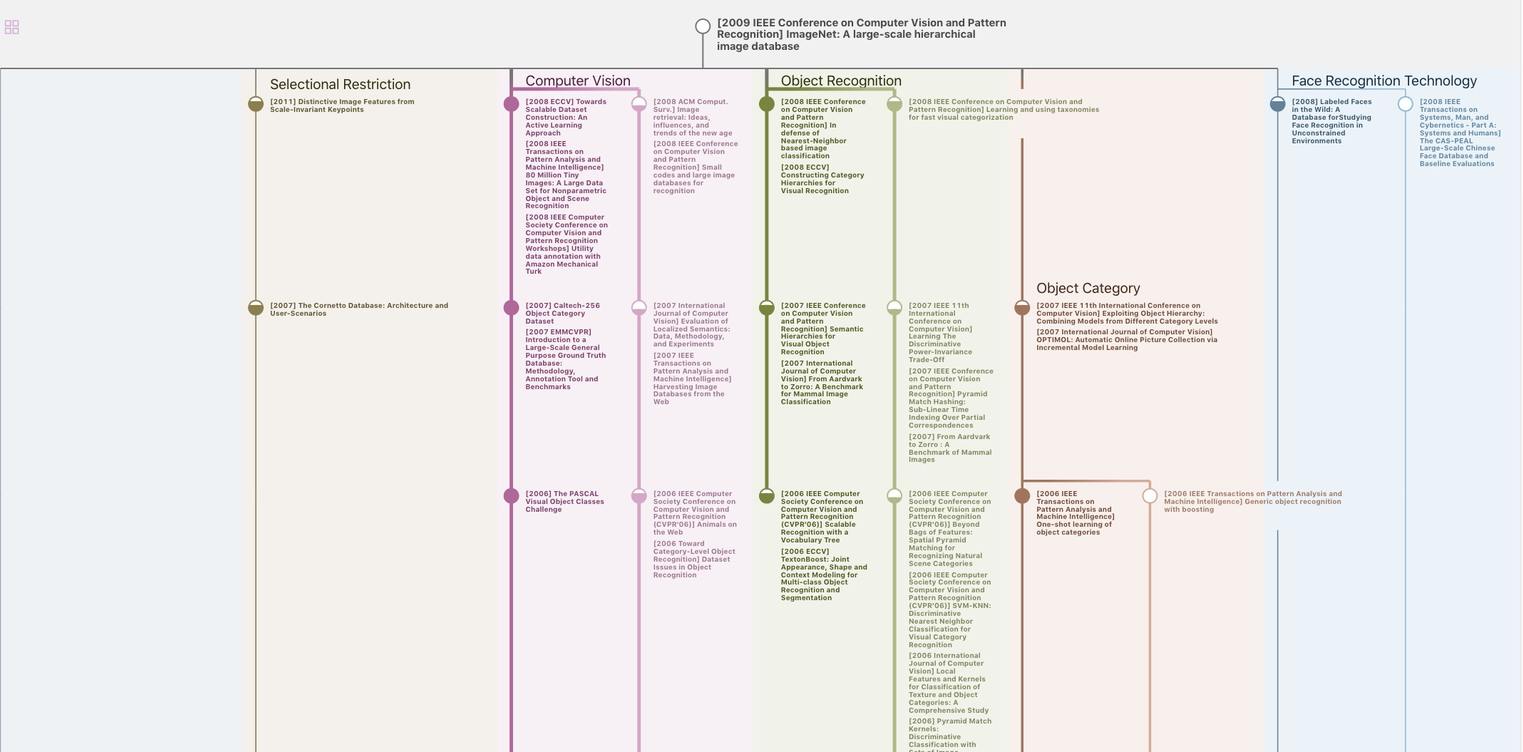
生成溯源树,研究论文发展脉络
Chat Paper
正在生成论文摘要