SNOW: Subscribing to Knowledge via Channel Pooling for Transfer & Lifelong Learning of Convolutional Neural Networks
ICLR(2020)
摘要
SNOW is an efficient learning method to improve training/serving throughput as well as accuracy for transfer and lifelong learning of convolutional neural networks based on knowledge subscription. SNOW selects the top-K useful intermediate
feature maps for a target task from a pre-trained and frozen source model through a novel channel pooling scheme, and utilizes them in the task-specific delta model. The source model is responsible for generating a large number of generic feature maps. Meanwhile, the delta model selectively subscribes to those feature maps and fuses them with its local ones to deliver high accuracy for the target task. Since a source model takes part in both training and serving of all target tasks
in an inference-only mode, one source model can serve multiple delta models, enabling significant computation sharing. The sizes of such delta models are fractional of the source model, thus SNOW also provides model-size efficiency.
Our experimental results show that SNOW offers a superior balance between accuracy and training/inference speed for various image classification tasks to the existing transfer and lifelong learning practices.
更多查看译文
关键词
channel pooling, efficient training and inferencing, lifelong learning, transfer learning, multi task
AI 理解论文
溯源树
样例
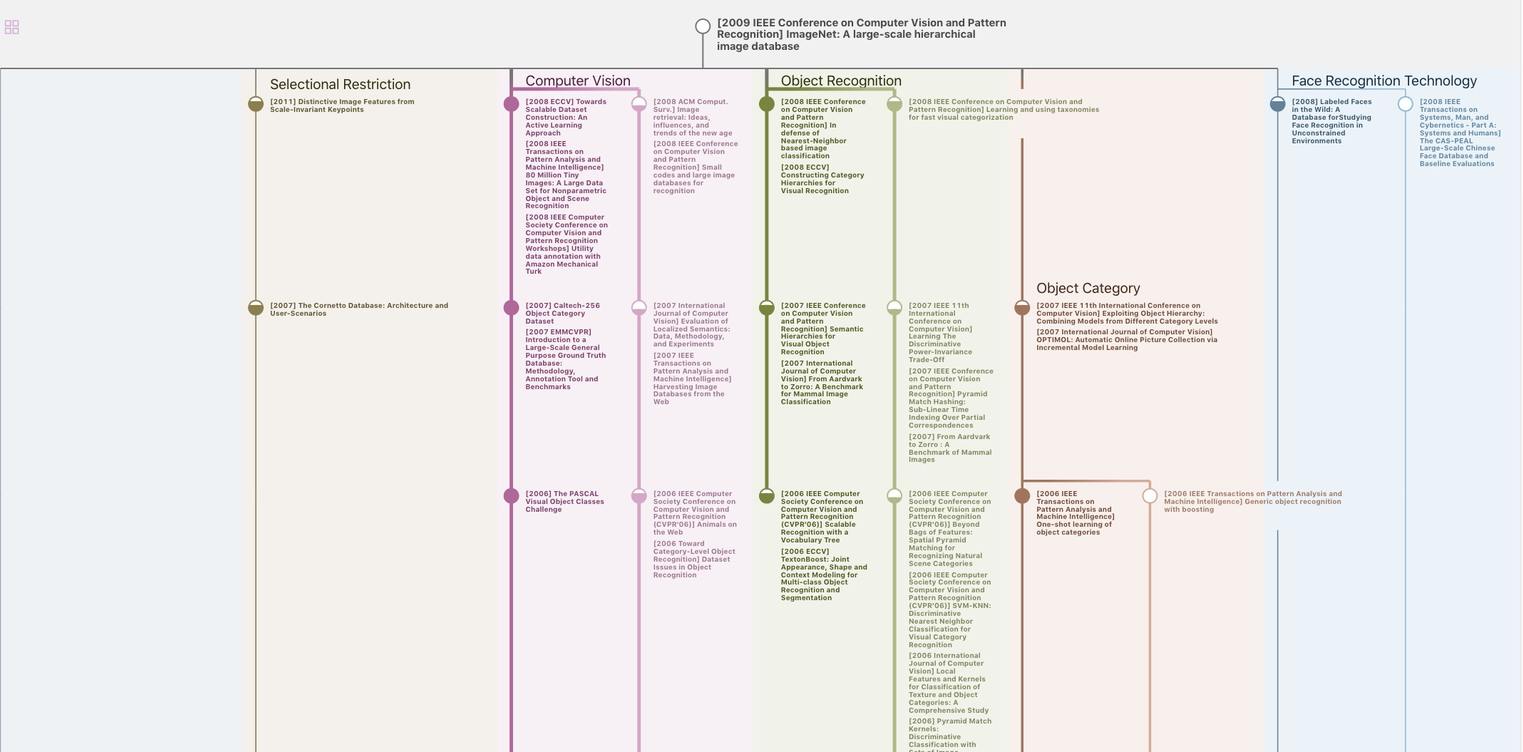
生成溯源树,研究论文发展脉络
Chat Paper
正在生成论文摘要