Machine Learning-Enabled Adaptation of Information Fusion Software Systems.
FUSION(2019)
摘要
Real-time control systems must fuse information from multiple sensors to perform mission tasks in dynamic environments. The volatility of these environments can cause sensor degradation or failure, reducing the accuracy and reliability of the information fusion. The fusion system must automatically adapt to these environmental changes to minimize their effect while still completing its objectives. Further complicating the situation, many systems contain non-adaptive legacy software, so a generic adaptation framework must be able to adapt the systemu0027s software without any semantic knowledge. In this paper, we present an approach that uses semantic-agnostic program transformation to expand the range of behavior of an Unmanned Underwater Vehicle (UUV) information fusion system and optimize its behavior against mission objectives using machine-learning techniques. An analysis of our approach showed that adapting a UUV with our adaptation framework resulted in a 50% increase in its ability to search for an object under water and return safely while perturbed by battery failures.
更多查看译文
关键词
machine learning,sensor fusion,data aggregation,optimization,UUV,Path Planner,Kalman Filter
AI 理解论文
溯源树
样例
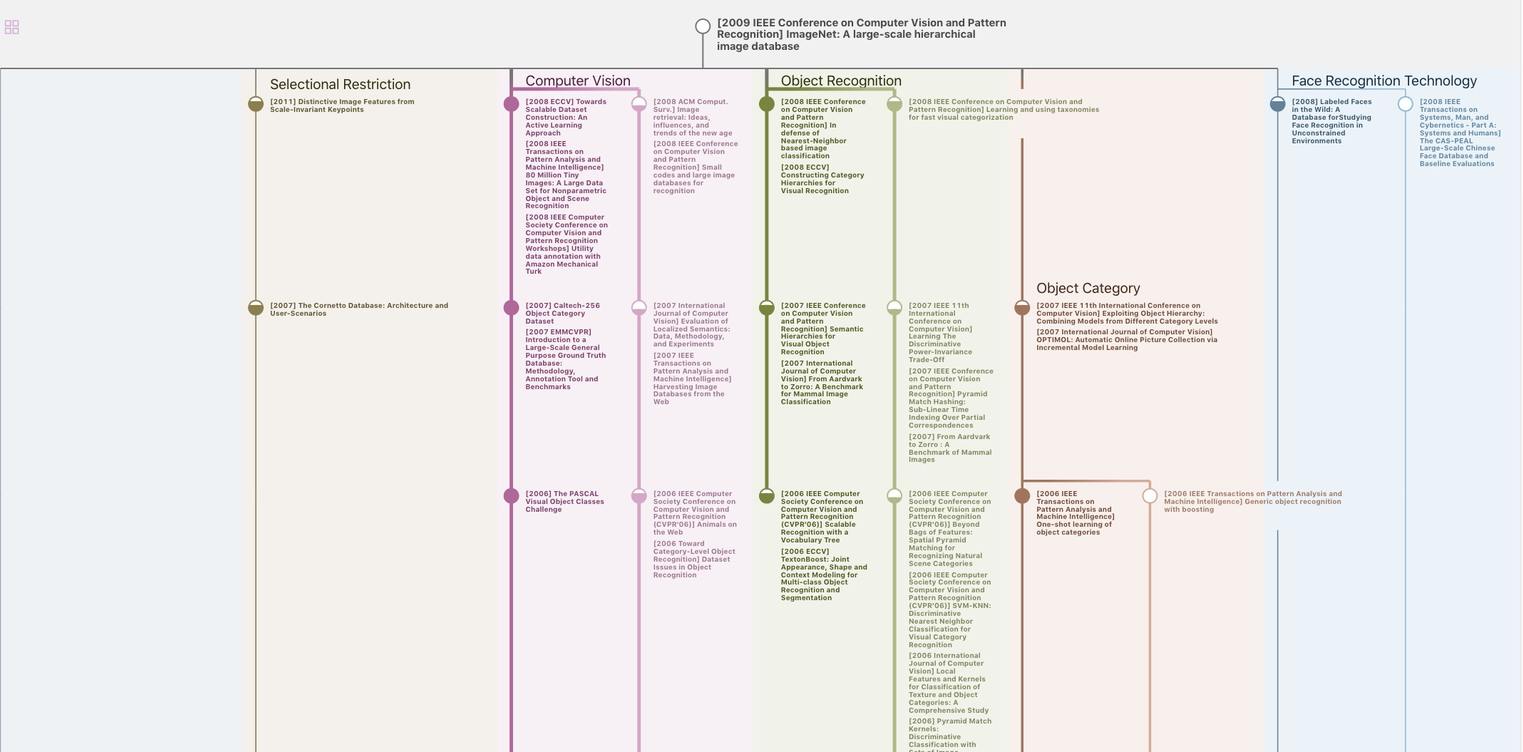
生成溯源树,研究论文发展脉络
Chat Paper
正在生成论文摘要