Toward Interpretable Machine Learning: Transparent Deep Neural Networks and Beyond
arxiv(2020)
摘要
With the broader and highly successful usage of machine learning in industry and the sciences, there has been a growing demand for explainable AI. Interpretability and explanation methods for gaining a better understanding about the problem solving abilities and strategies of nonlinear Machine Learning such as Deep Learning (DL), LSTMs, and kernel methods are therefore receiving increased attention. In this work we aim to (1) provide a timely overview of this active emerging field and explain its theoretical foundations, (2) put interpretability algorithms to a test both from a theory and comparative evaluation perspective using extensive simulations, (3) outline best practice aspects i.e. how to best include interpretation methods into the standard usage of machine learning and (4) demonstrate successful usage of explainable AI in a representative selection of application scenarios. Finally, we discuss challenges and possible future directions of this exciting foundational field of machine learning.
更多查看译文
关键词
interpretable machine learning,transparent deep neural networks,deep neural networks,neural networks,machine learning
AI 理解论文
溯源树
样例
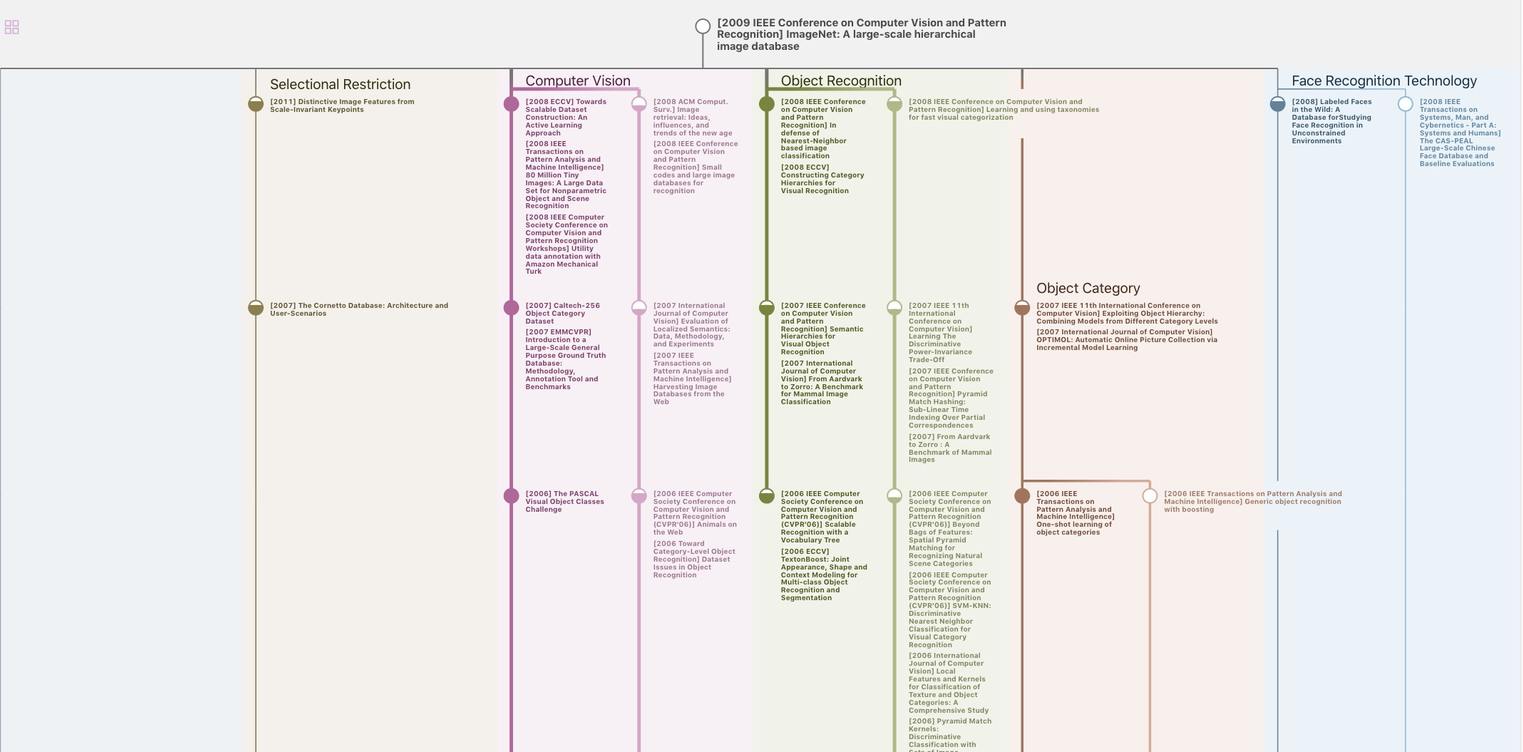
生成溯源树,研究论文发展脉络
Chat Paper
正在生成论文摘要