Asr Error Correction And Domain Adaptation Using Machine Translation
2020 IEEE INTERNATIONAL CONFERENCE ON ACOUSTICS, SPEECH, AND SIGNAL PROCESSING(2020)
摘要
Off-the-shelf pre-trained Automatic Speech Recognition (ASR) systems are an increasingly viable service for companies of any size building speech-based products. While these ASR systems are trained on large amounts of data, domain mismatch is still an issue for many such parties that want to use this service as-is leading to not so optimal results for their task. We propose a simple technique to perform domain adaptation for ASR error correction via machine translation. The machine translation model is a strong candidate to learn a mapping from out-of-domain ASR errors to in-domain terms in the corresponding reference files. We use two off-the-shelf ASR systems in this work: Google ASR (commercial) and the ASPIRE model (open-source). We observe 7% absolute improvement in word error rate and 4 point absolute improvement in BLEU score in Google ASR output via our proposed method. We also evaluate ASR error correction via a downstream task of Speaker Diarization that captures speaker style, syntax, structure and semantic improvements we obtain via ASR correction.
更多查看译文
关键词
Domain adaptation, ASR error correction, machine translation, diarization, medical transcription
AI 理解论文
溯源树
样例
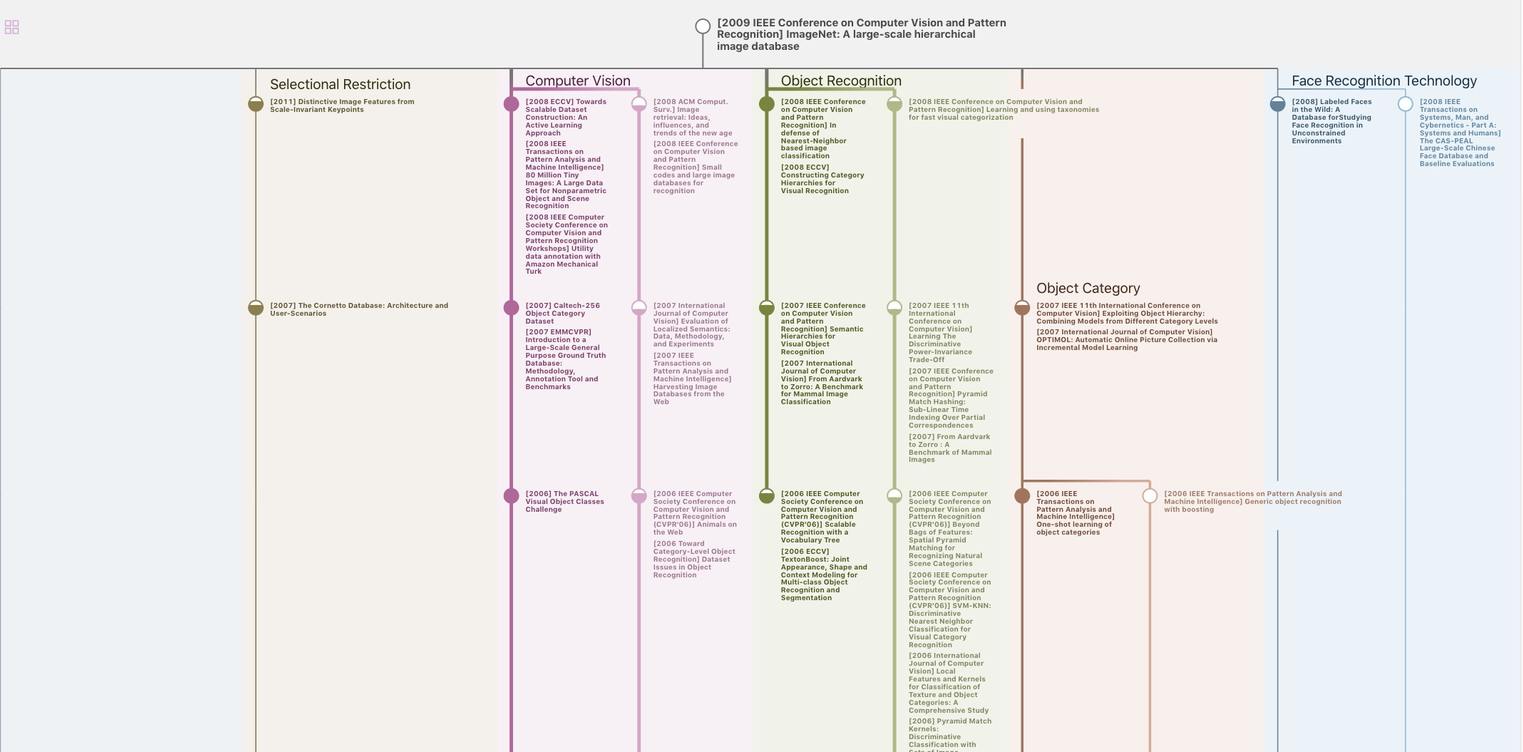
生成溯源树,研究论文发展脉络
Chat Paper
正在生成论文摘要