Sparse Non-Negative Multivariate Curve Resolution: L0, L1, or L2 Norms?
Chemometrics and intelligent laboratory systems(2020)
摘要
Several constraints are designed to further restrict bilinear decompositions to a unique solution. Constraints are physico-chemical restrictions on the curve resolution task. Sparsity, as a constraint, was introduced to create solutions with zero elements. As neither the number of zeros nor the places of zeros are not initially available, sparsity constraint should be implemented with caution. Regarding sparsity constraint, two important issues can be addressed. The first issue is the effect of sparsity constraint on the possible solutions of bilinear decompositions, i.e., set of sparse solutions. The second issue is the type of Lp-norm, {p=0,1,2}, for the sparsity implementation. Self-modeling curve resolution (SMCR) tools (say Borgen-Rajkó plot) draw a clear picture of the data micro-structure. Focusing on the geometry of bilinear data sets, outer-polygon as the non-negativity boundary of possible solutions contains all the sparse solutions.In this contribution, we shed light on all possible sparse solutions of a bilinear decomposition, and it was shown that outer-polygon is the set of sparse solutions. Finally, Lp-norms were calculated for the different feasible profiles, and it is revealed that L0-norm minimization and L2-norm maximization are the correct way toward the sparse/est solutions. Finally, this study targets LC/GC-MS, hyperspectral images, and all of the data sets which contain zero values in their profiles. Since omics researches use extensively, e.g., mass spectrometry, thus a wide community expected to be interested in our report on the limitations of sparsity.
更多查看译文
关键词
Sparsity constraint,L-p-norms,LC/GC-MS data,Hyperspectral image,Borgen-Rajko plot,Outer-polygon
AI 理解论文
溯源树
样例
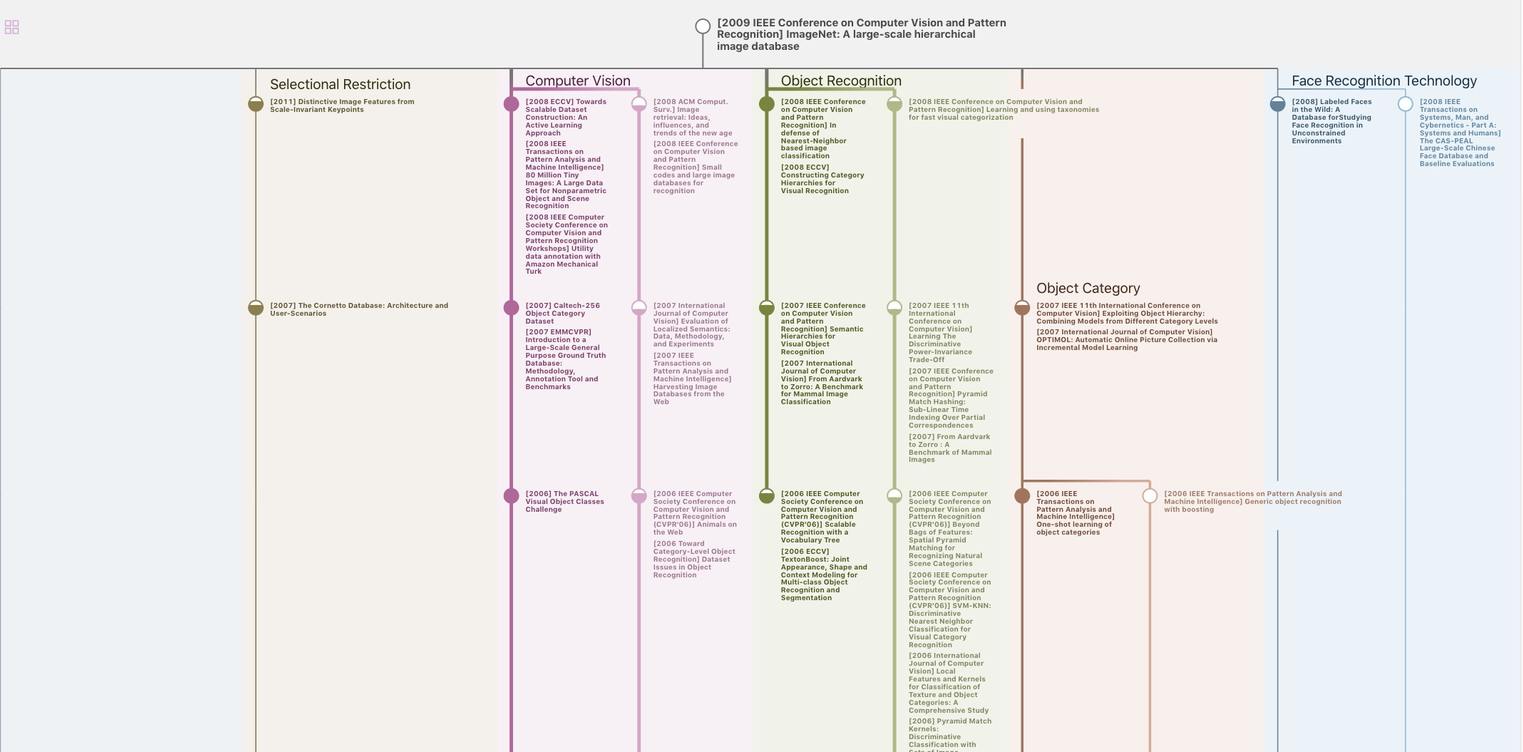
生成溯源树,研究论文发展脉络
Chat Paper
正在生成论文摘要