RegBoost: a gradient boosted multivariate regression algorithm
International Journal of Crowd Science(2020)
摘要
Purpose – Inspired by the basic idea of gradient boosting, this study aims to design a novel multivariate regression ensemble algorithm RegBoost by using multivariate linear regression as a weak predictor. Design/methodology/approach – To achieve nonlinearity after combining all linear regression predictors, the training data is divided into two branches according to the prediction results using the current weak predictor. The linear regression modeling is recursively executed in two branches. In the test phase, test data is distributed to a specific branch to continue with the next weak predictor. The final result is the sum of all weak predictors across the entire path. Findings – Through comparison experiments, it is found that the algorithm RegBoost can achieve similar performance to the gradient boosted decision tree (GBDT). The algorithm is very effective compared to linear regression. Originality/value – This paper attempts to design a novel regression algorithm RegBoost with reference to GBDT. To the best of the knowledge, for the first time, RegBoost uses linear regression as a weak predictor, and combine with gradient boosting to build an ensemble algorithm.
更多查看译文
关键词
Linear regression,Ensemble learning,Gradient boosting,RMSE
AI 理解论文
溯源树
样例
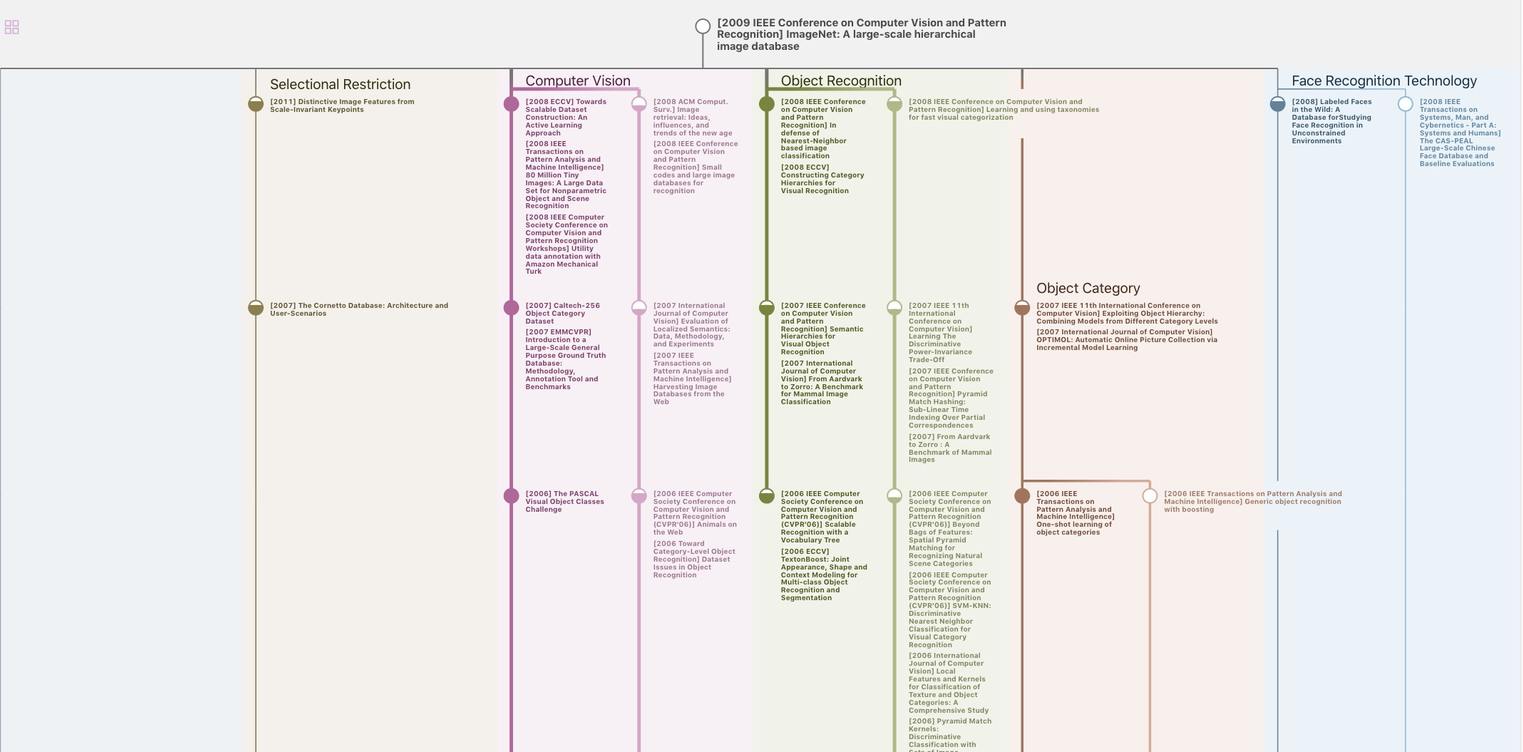
生成溯源树,研究论文发展脉络
Chat Paper
正在生成论文摘要