Notice of Violation of IEEE Publication Principles: Evaluating machine learning algorithms for fake news detection
2017 IEEE 15th Student Conference on Research and Development (SCOReD)(2017)
摘要
Notice of Violation of IEEE Publication Principles
"Evaluating Machine Learning Algorithms for Fake News Detection"
by Shlok Gilda
in the 2017 IEEE 15th Student Conference on Research and Development (SCOReD), December 2017, pp.110-115
After careful and considered review of the content and authorship of this paper by a duly constituted expert committee, this paper has been found to be in violation of IEEE's Publication Principles.
This paper is a near duplication of the original text from the paper cited below. The original text was copied without attribution (including appropriate references to the original author(s) and/or paper title) and without permission.
Due to the nature of this violation, reasonable effort should be made to remove all past references to this paper, and future references should be made to the following article:
"Fake News Detection Exploring the Application of NLP Methods to Machine Identification of Misleading News Sources"
by Lauren Dyson & Alden Golab
in Final Project for CAPP 30255 Advanced Machine Learning for Public Policy, University of Chicago
This work explores the application of natural language processing techniques for the detection of ‘fake news’, that is, misleading news stories that come from non-reputable sources. Using a dataset obtained from Signal Media and a list of sources from OpenSources.co, we apply term frequency-inverse document frequency (TF-IDF) of bi-grams and probabilistic context free grammar (PCFG) detection to a corpus of about 11,000 articles. We test our dataset on multiple classification algorithms — Support Vector Machines, Stochastic Gradient Descent, Gradient Boosting, Bounded Decision Trees, and Random Forests. We find that TF-IDF of bi-grams fed into a Stochastic Gradient Descent model identifies non-credible sources with an accuracy of 77.2%, with PCFGs having slight effects on recall.
更多"Evaluating Machine Learning Algorithms for Fake News Detection"
by Shlok Gilda
in the 2017 IEEE 15th Student Conference on Research and Development (SCOReD), December 2017, pp.110-115
After careful and considered review of the content and authorship of this paper by a duly constituted expert committee, this paper has been found to be in violation of IEEE's Publication Principles.
This paper is a near duplication of the original text from the paper cited below. The original text was copied without attribution (including appropriate references to the original author(s) and/or paper title) and without permission.
Due to the nature of this violation, reasonable effort should be made to remove all past references to this paper, and future references should be made to the following article:
"Fake News Detection Exploring the Application of NLP Methods to Machine Identification of Misleading News Sources"
by Lauren Dyson & Alden Golab
in Final Project for CAPP 30255 Advanced Machine Learning for Public Policy, University of Chicago
This work explores the application of natural language processing techniques for the detection of ‘fake news’, that is, misleading news stories that come from non-reputable sources. Using a dataset obtained from Signal Media and a list of sources from OpenSources.co, we apply term frequency-inverse document frequency (TF-IDF) of bi-grams and probabilistic context free grammar (PCFG) detection to a corpus of about 11,000 articles. We test our dataset on multiple classification algorithms — Support Vector Machines, Stochastic Gradient Descent, Gradient Boosting, Bounded Decision Trees, and Random Forests. We find that TF-IDF of bi-grams fed into a Stochastic Gradient Descent model identifies non-credible sources with an accuracy of 77.2%, with PCFGs having slight effects on recall.
查看译文
关键词
fake news,ieee publication principles,machine learning
AI 理解论文
溯源树
样例
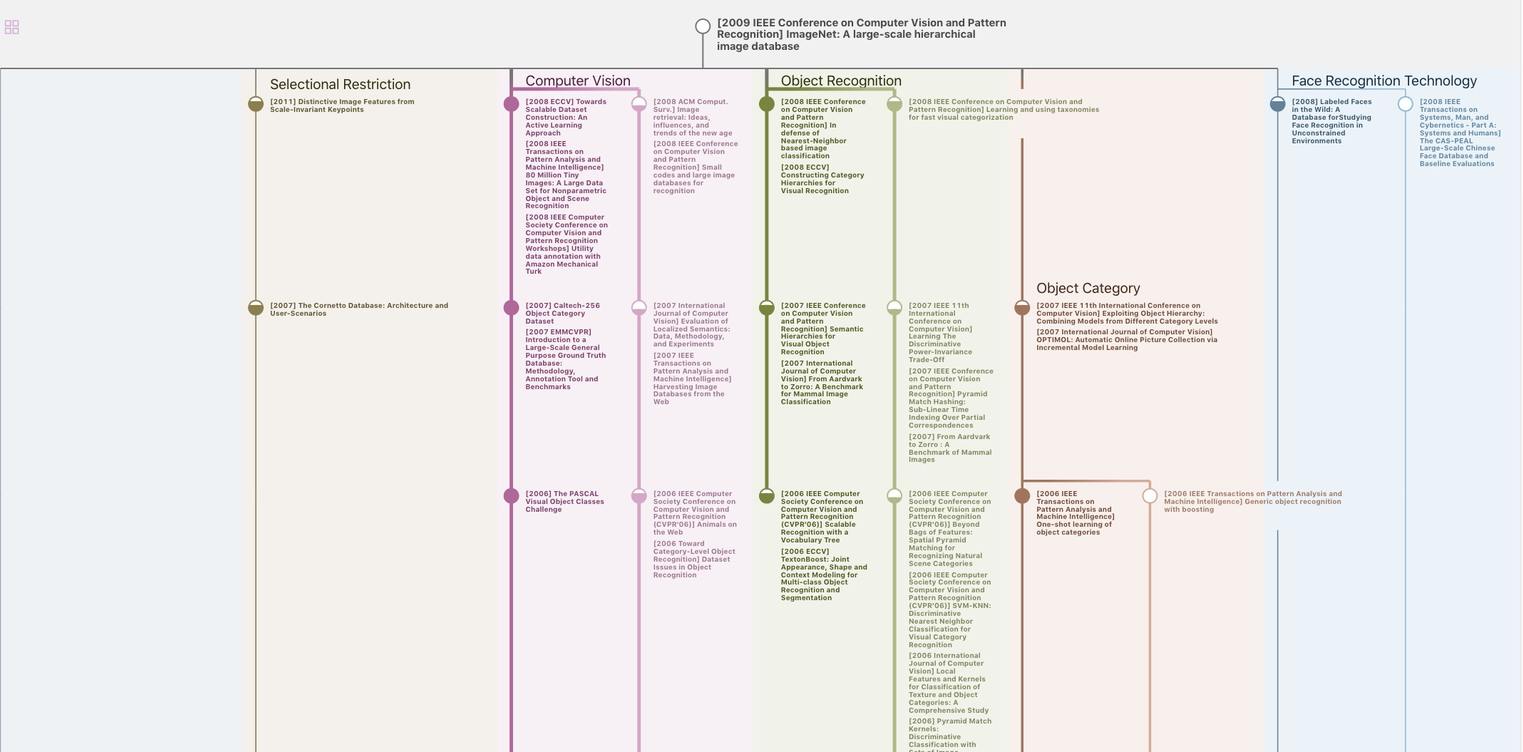
生成溯源树,研究论文发展脉络
Chat Paper
正在生成论文摘要