Adversarial Attribute-Text Embedding for Person Search with Natural Language Query
IEEE Transactions on Multimedia(2020)
摘要
The newly emerging task of person search with natural language query aims at retrieving the target pedestrian by a text description of the pedestrian. It is more applicable compared to person search with image/video query, i.e., person re-identification. In this paper, we propose a novel Adversarial Attribute-Text Embedding (AATE) network for person search with text query. In particular, a cross-modal adversarial learning module is proposed to learn discriminative and modality-invariant visual-textual features. It consists of a cross-modal learner and a modality discriminator, playing a min-max game in an adversarial learning way. The former is to improve intra-modality discrimination and inter-modality invariance towards confusing the modality discriminator. The latter is to distinguish the features from different modalities and boost the learning of modality-invariant features. Moreover, a visual attribute graph convolutional network is proposed to learn visual attributes of pedestrians, which possess better descriptiveness, interpretability and robustness compared to pedestrian appearance features. A hierarchical text embedding network, consisting of multi-stacked bidirectional LSTMs and a textual attention block, is developed to extract effective textual features from text descriptions of pedestrians. Extensive experimental results on two challenging benchmarks, have demonstrated the effectiveness of the proposed approach.
更多查看译文
关键词
Visualization,Natural languages,Feature extraction,Cameras,Semantics,Task analysis,Robustness
AI 理解论文
溯源树
样例
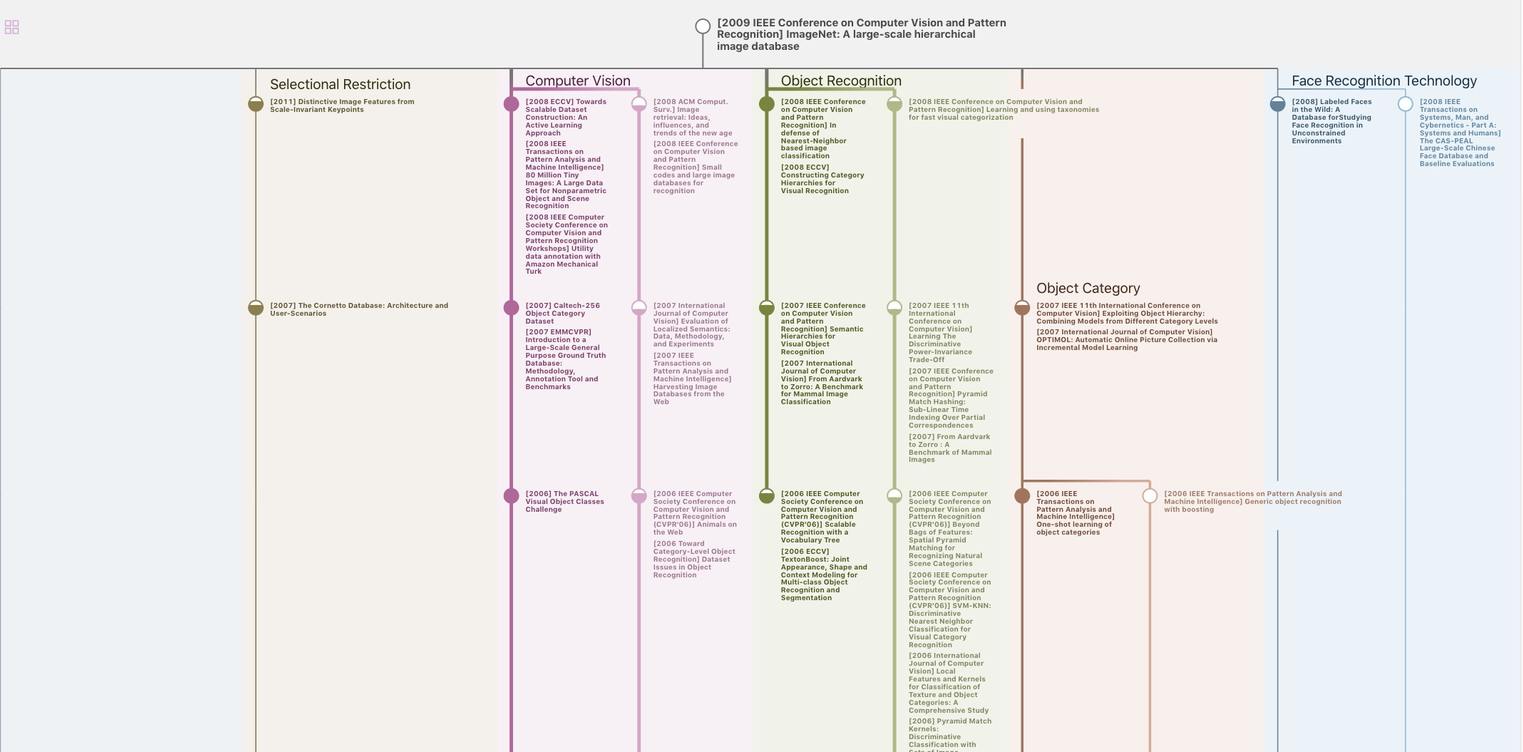
生成溯源树,研究论文发展脉络
Chat Paper
正在生成论文摘要