U-Net cascaded with dilated convolution for medical image registration
chinese automation congress(2019)
摘要
Registration is a critical step in many biomedical image analysis tasks. In this work, we propose a novel cascaded 3D U-Net with dilated convolution for volumetric image registration. We implement a coarse-to-fine registration strategy that commonly used in traditional registration methods by cascading two 3D U-Net based networks. In the first registration stage, a 3D U-Net network is employed to predict the large and coarse displacements. In order to capture more precise pixel-level displacement field, in the second stage, we propose to substitute the down-sampling operations in the encoding stage of 3D U-Net by dilated convolutions. As result, the resolution of feature map can be maintained as well as its field-of-view and semantic information. In addition, to obtain a more parameter-efficient network that is essential for volumetric image analysis, we replace the 3D convolution kernel by 3D dense separated convolution (3DDSC) module. We evaluated the proposed method on two commonly used datasets, and the results demonstrated that our method could obtain better performance than state-of-the-art image registration algorithms with short execution time.
更多查看译文
关键词
image registration,U-Net,dilated convolution
AI 理解论文
溯源树
样例
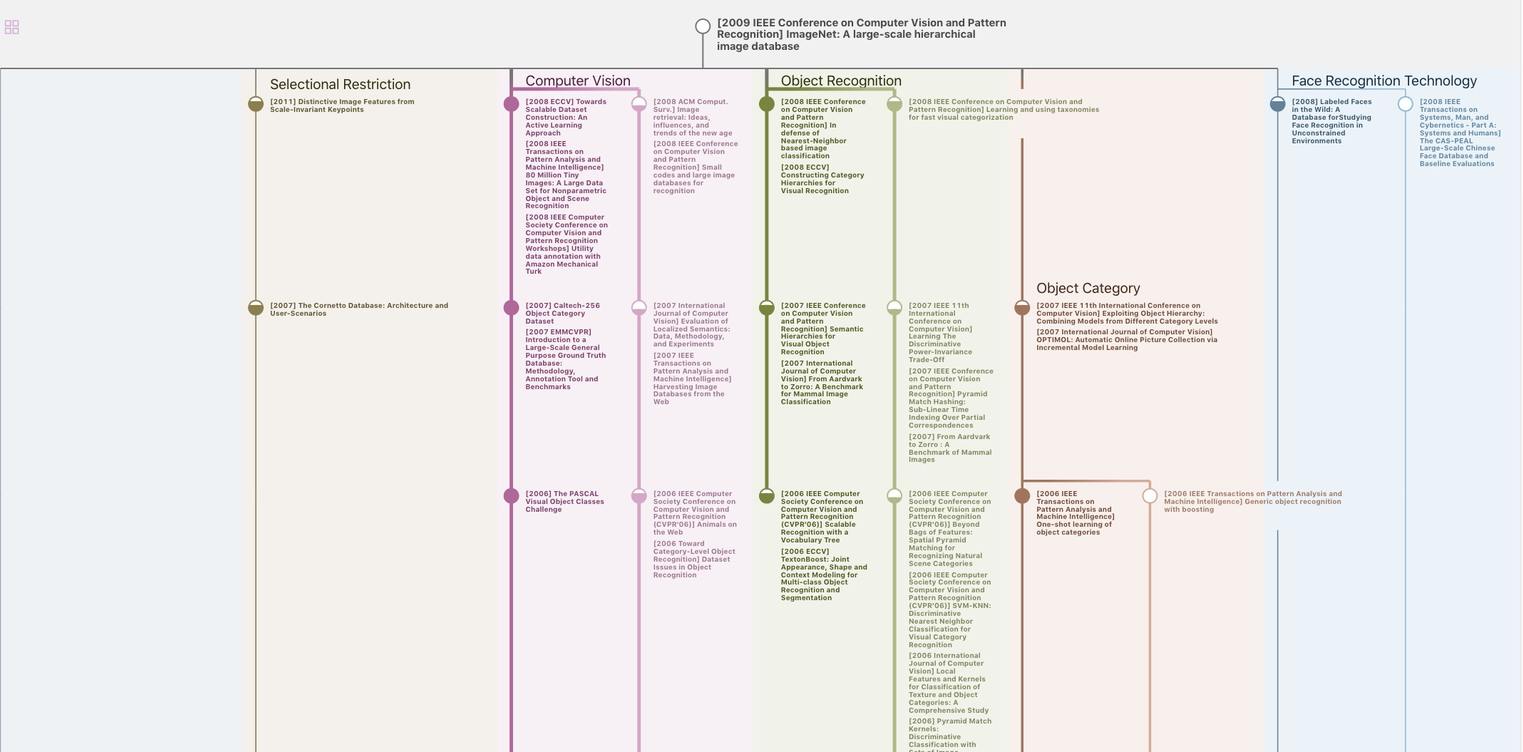
生成溯源树,研究论文发展脉络
Chat Paper
正在生成论文摘要