Interpreting deep convolutional neural network classification results indirectly through the preprocessing feature fusion method in ship image classification
JOURNAL OF APPLIED REMOTE SENSING(2020)
摘要
In spite of widespread adoption, deep convolutional neural networks (DCNNs) have a reputation for remaining "black boxes." Due to the feature extraction ability of DCNN itself, good predicted results can be achieved without any processing of the raw input images, but it is hard to explain what features of the raw images the results are based on. We propose a preprocessing feature fusion method, based on the Sobel and Canny edge operators, which we treat as the interpretation of the raw images. This method obtains the interpretable edge feature by fusing features in raw ship images, which helps to explain that the DCNN ship classification result is based on the ship edge feature. That is to say, through the interpretation of the raw ship images, we indirectly interpret the classification results of the DCNN to some degree. In subsequent experiments, the highest average accuracy of 85.31% was achieved from the fusion-1 dataset, whereas the highest average accuracy of 74.4% was achieved from the balanced fusion-1 dataset, and the best mean average accuracy of 74.3% is achieved from the cross-validated experiments, which helps to verify the validity of our preprocessing method. (C) 2020 Society of Photo-Optical Instrumentation Engineers (SPIE).
更多查看译文
关键词
deep convolutional neural network,preprocessing method,feature fusion,Sobel Canny
AI 理解论文
溯源树
样例
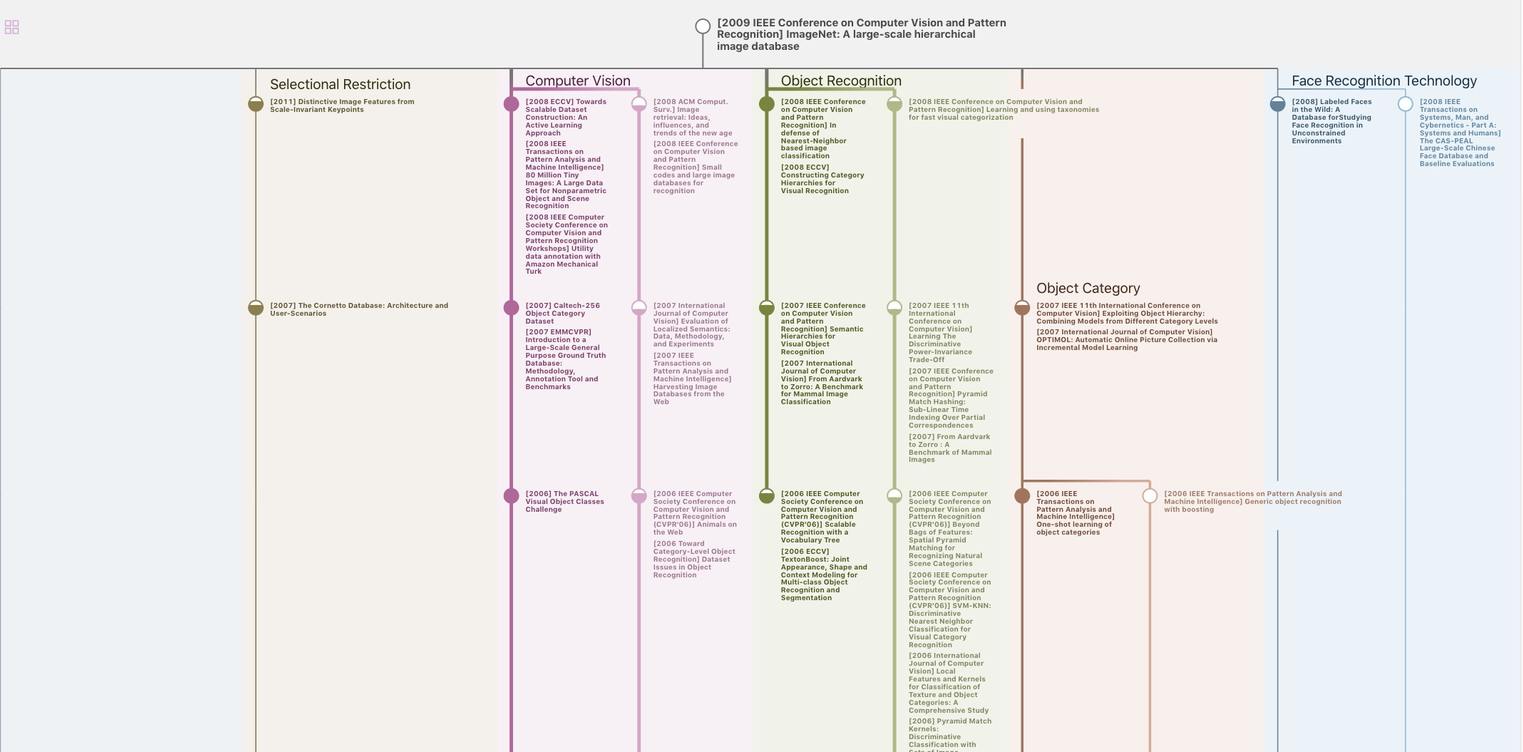
生成溯源树,研究论文发展脉络
Chat Paper
正在生成论文摘要