Flynet 2.0: Drosophila Heart 3d (2d+Time) Segmentation In Optical Coherence Microscopy Images Using A Convolutional Long Short-Term Memory Neural Network
BIOMEDICAL OPTICS EXPRESS(2020)
摘要
A custom convolutional neural network (CNN) integrated with convolutional long short-term memory (LSTM) achieves accurate 3D (2D + time) segmentation in cross-sectional videos of the Drosophila heart acquired by an optical coherence microscopy (OCM) system. While our previous FlyNet 1.0 model utilized regular CNNs to extract 2D spatial information from individual video frames, convolutional LSTM, FlyNet 2.0, utilizes both spatial and temporal information to improve segmentation performance further. To train and test FlyNet 2.0, we used 100 datasets including 500,000 fly heart OCM images. OCM videos in three developmental stages and two heartbeat situations were segmented achieving an intersection over union (IOU) accuracy of 92%. This increased segmentation accuracy allows morphological and dynamic cardiac parameters to be better quantified. (C) 2020 Optical Society of America under the terms of the OSA Open Access Publishing Agreement
更多查看译文
AI 理解论文
溯源树
样例
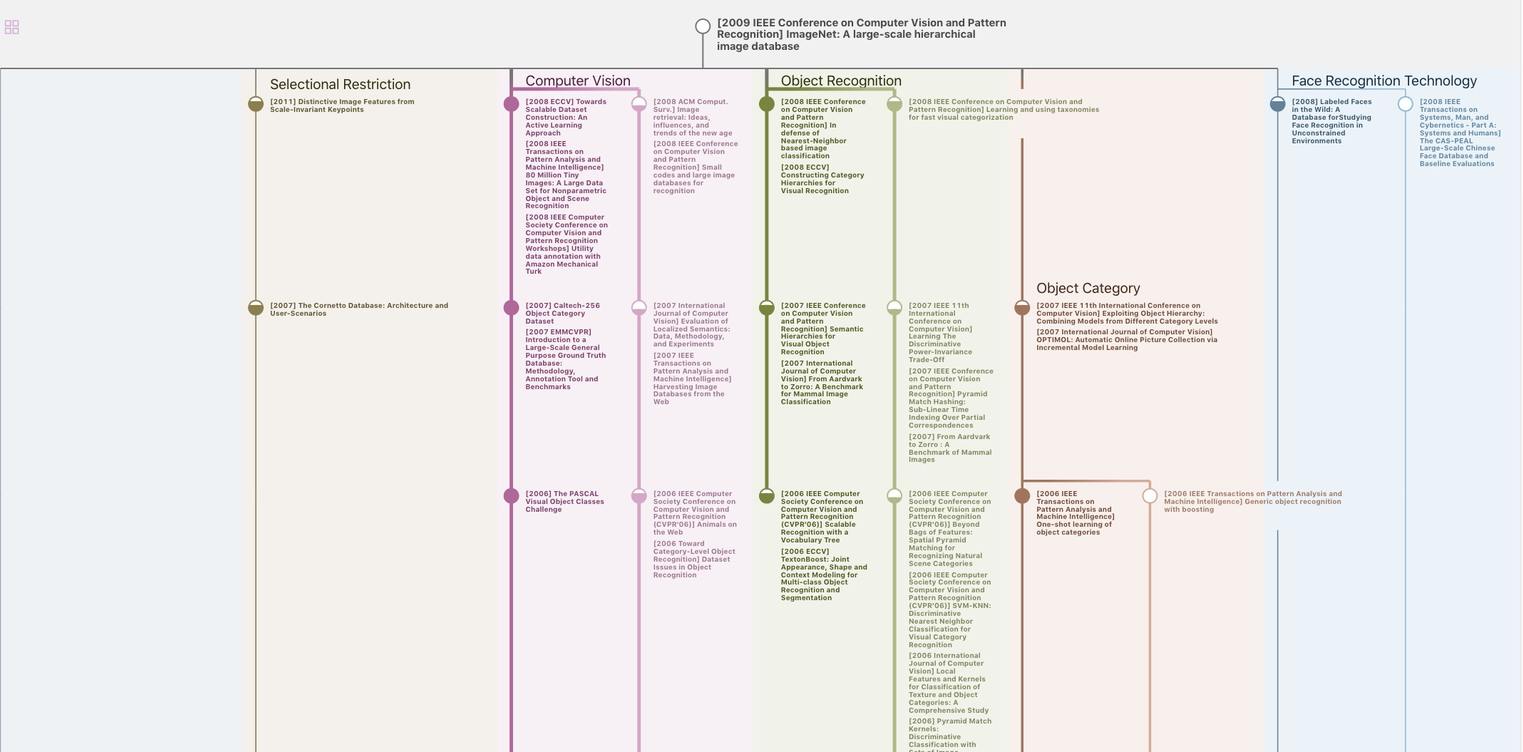
生成溯源树,研究论文发展脉络
Chat Paper
正在生成论文摘要