Can In-Memory/Analog Accelerators Be A Silver Bullet For Energy-Efficient Inference?
2019 IEEE INTERNATIONAL ELECTRON DEVICES MEETING (IEDM)(2019)
摘要
A lot of energy-efficient accelerators for inference has been reported. Our investigation result on dependence of energy efficiency on bit precision of accelerators shows that energy efficiency of in-memory/analog accelerators looks better than that of digital accelerators. However, accuracy of in-memory/analog accelerators is usually deteriorated. In order to practically reduce average bit precision for weights while maintaining accuracy, we introduce our proposed filter-wise quantization technique and a specific HW architecture with variable bit width MAC units used in a bit-parallel manner. Finally, we discuss whether in-memory/analog accelerators can actually be a silver bullet for energy-efficient inference.
更多查看译文
关键词
in-memory/analog accelerators,energy-efficient
AI 理解论文
溯源树
样例
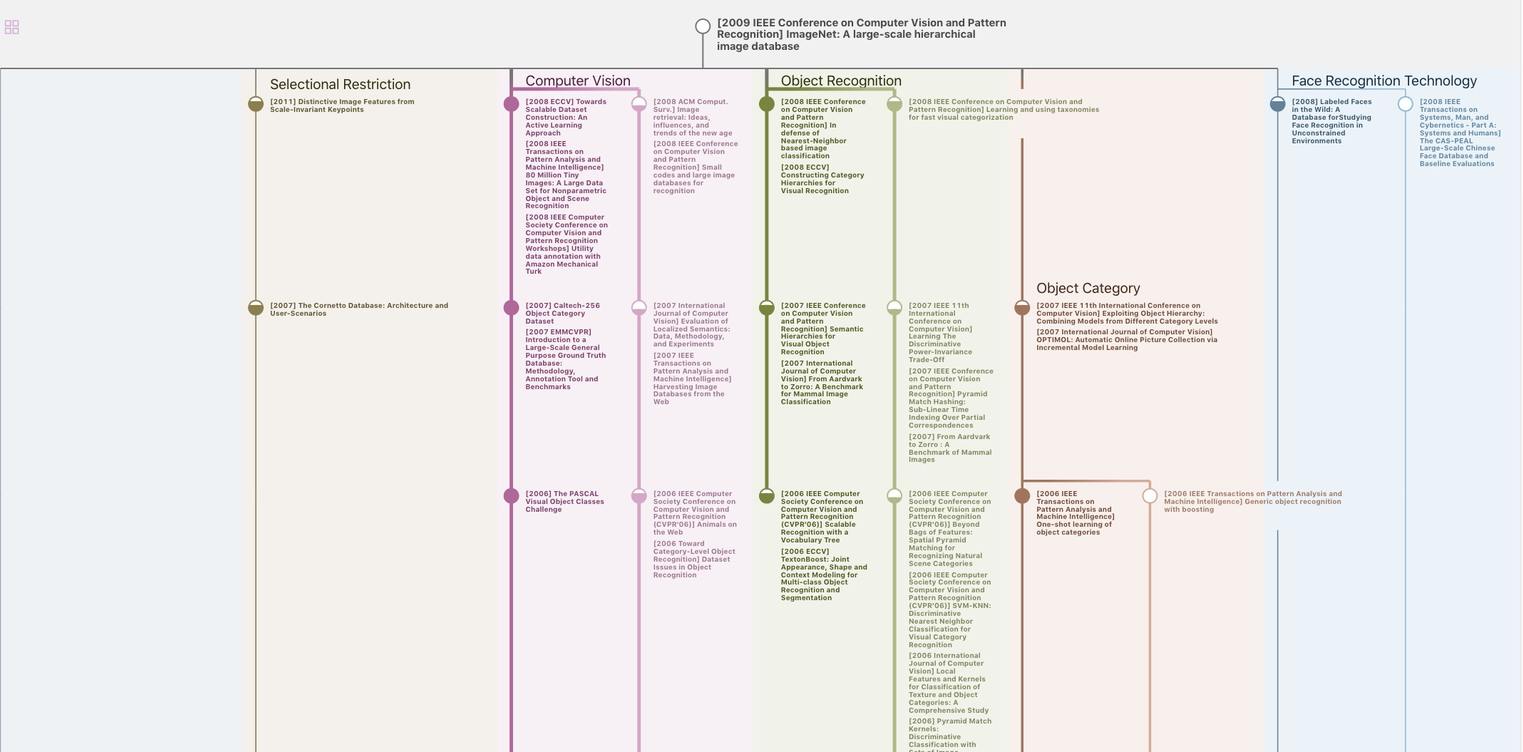
生成溯源树,研究论文发展脉络
Chat Paper
正在生成论文摘要