Annual Streamflow Time Series Prediction Using Extreme Learning Machine Based on Gravitational Search Algorithm and Variational Mode Decomposition
JOURNAL OF HYDROLOGIC ENGINEERING(2020)
摘要
Accurate annual runoff prediction plays an important role in modern water resources planning and management. Here, a hybrid model using evolutionary extreme learning machine and variational mode decomposition (VMD) is developed to forecast annual runoff time series. In the proposed method, the VMD method is first used to decompose the original streamflow into a series of disjoint subcomponents; second, each subcomponent is forecasted by constructing an appropriate extreme learning machine model while the gravitational search algorithm is adopted to tune the model parameters; finally, the aggregated output generated by the forecasting results of all the models is treated as the final simulated output. The annual runoff data series of three huge hydropower reservoirs in China are chosen to testify the performance of the proposed forecasting model. The results show that the developed model can outperform several traditional methods with respect to the employed statistical indexes. Thus, the decomposition-ensemble idea is helpful to yield accurate and stable forecasting results, while the proposed forecasting method can provide strong technical support for operators in water resources and power systems.
更多查看译文
关键词
Hydrological time series forecasting,Variational mode decomposition,Evolutionary extreme learning machine,Gravitational search algorithm,Decomposition and ensemble
AI 理解论文
溯源树
样例
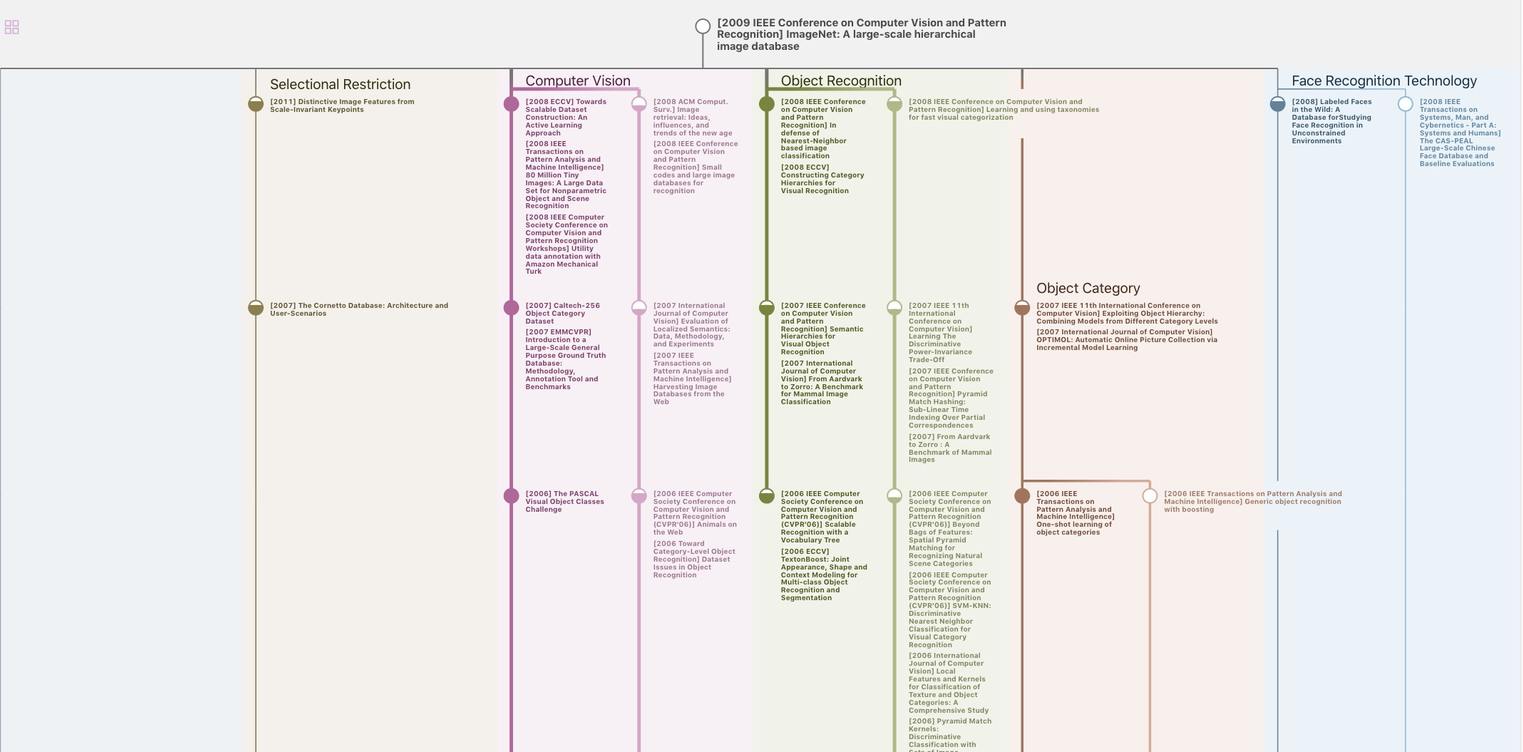
生成溯源树,研究论文发展脉络
Chat Paper
正在生成论文摘要