Joint image deblurring and matching with feature-based sparse representation prior
Pattern Recognition(2020)
摘要
Image matching aims to find a similar area of the small image in the large image, which is one of the key steps in image fusion and vision-based navigation; however, most matching methods perform poorly when the images to be matched are blurred. Traditional approaches for blurred image matching usually follow a two-stage framework - first resorting to image deblurring and then performing image matching with the recovered image. However, the matching accuracy of these methods often suffers greatly from the deficiency of image deblurring. Recently, a joint image deblurring and matching method that utilizes the sparse representation prior to exploit the correlation between deblurring and matching was proposed to address this problem and found to obtain a higher matching accuracy. Yet, that technique is not efficient when the image is seriously blurred, and the method’s time complexity is excessive. In this paper, we propose a joint image deblurring and matching approach with a feature-based sparse representation prior. Our approach utilizes two-directional two-dimensional (2D)2PCA to extract feature vectors from images and obtains a sparse representation prior in a robust feature space rather than the original pixel space, thus mitigating the influence of image blur. Moreover, the reduction in the feature dimension can also increase the computational efficiency. Extensive experiments show that our approach significantly outperforms state-of-the-art approaches in terms of both accuracy and speed.
更多查看译文
关键词
Blurred image matching,Joint image deblurring and matching,Sparse representation priorsparse,(2D)2PCA feature
AI 理解论文
溯源树
样例
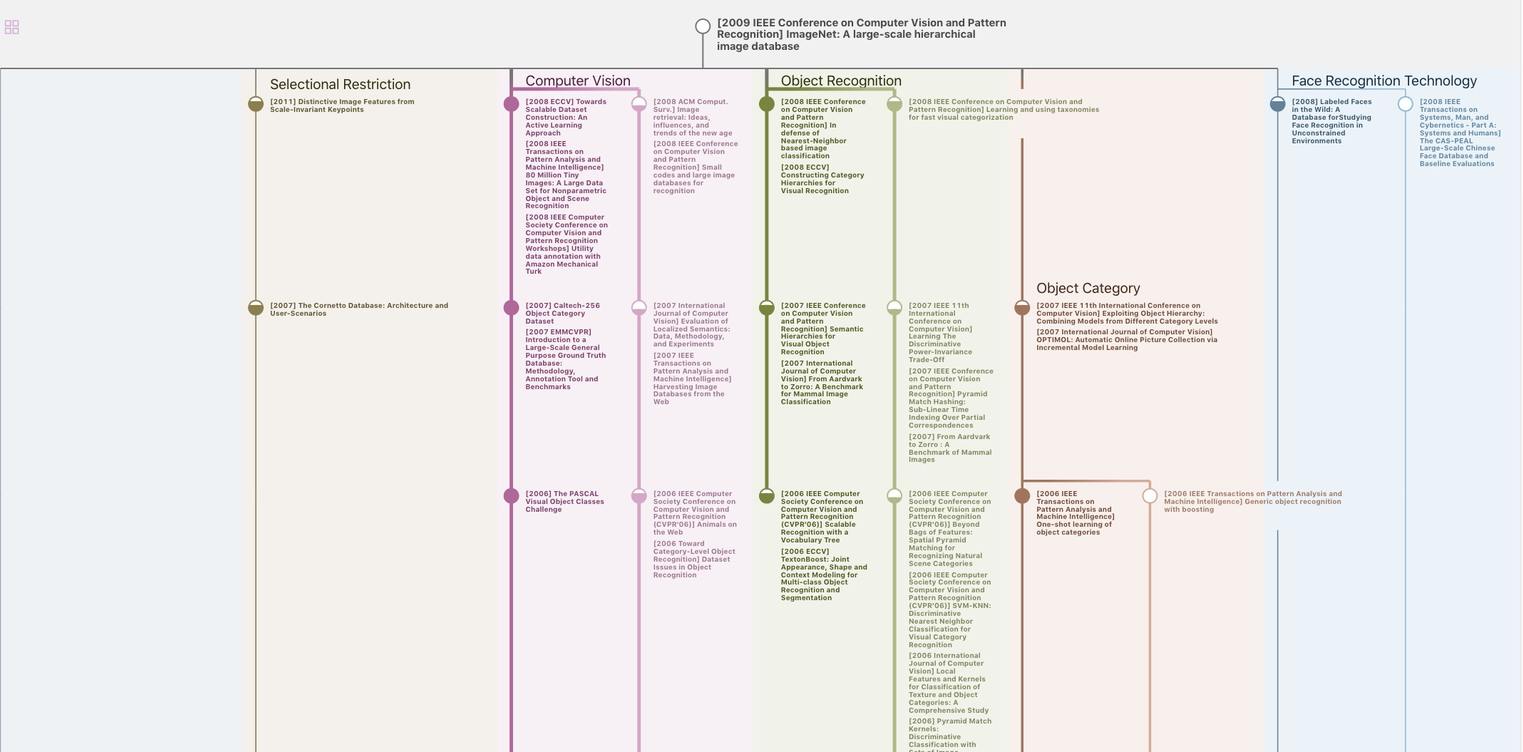
生成溯源树,研究论文发展脉络
Chat Paper
正在生成论文摘要