Weakly Supervised Easy-to-hard Learning for Object Detection in Image Sequences
Neurocomputing(2020)
摘要
Object detection is an important research problem in computer vision. Convolutional Neural Networks (CNN) based deep learning models could be used for this problem, but it would require a large number of manual annotated objects for training or fine-tuning. Unfortunately, fine-grained manually annotated objects are not available in many cases. Usually, it is possible to obtain imperfect initialized detections by some weak object detectors using some weak supervisions like the prior knowledge of shape, size or motion. In some real-world applications, objects have little inter-occlusions and split/merge difficulties, so the spatio-temporal consistency in object tracking are well preserved in the image sequences/videos. Starting from the imperfect initialization, this paper proposes a new easy-to-hard learning method to incrementally improve the object detection in image sequences/videos by an unsupervised spatio-temporal analysis which involves more complex examples that are hard for object detection for next-iteration training. The proposed method does not require manual annotations, but uses weak supervisions and spatio-temporal consistency in tracking to simulate the supervisions in the CNN training. Experimental results on three different tasks show significant improvements over the initialized detections by the weak object detectors.
更多查看译文
关键词
Weakly supervised,Easy-to-hard learning,Spatio-temporal consistency
AI 理解论文
溯源树
样例
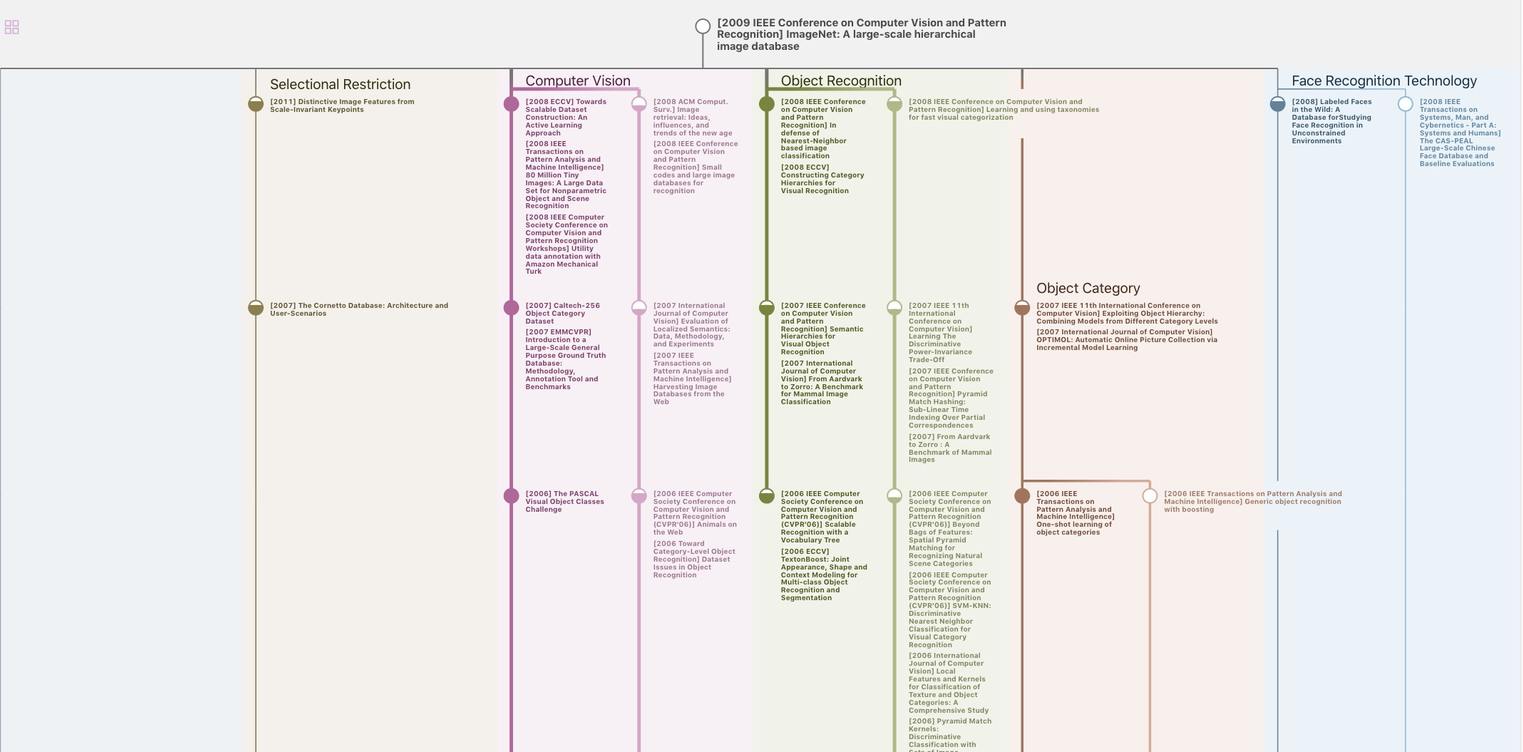
生成溯源树,研究论文发展脉络
Chat Paper
正在生成论文摘要