Fusion of Five Satellite-Derived Products Using Extremely Randomized Trees to Estimate Terrestrial Latent Heat Flux over Europe
REMOTE SENSING(2020)
摘要
An accurate estimation of spatially and temporally continuous latent heat flux (LE) is essential in the assessment of surface water and energy balance. Various satellite-derived LE products have been generated to enhance the simulation of terrestrial LE, yet each individual LE product shows large discrepancies and uncertainties. Our study used Extremely Randomized Trees (ETR) to fuse five satellite-derived terrestrial LE products to reduce uncertainties from the individual products and improve terrestrial LE estimations over Europe. The validation results demonstrated that the estimation using the ETR fusion method increased the R-2 of five individual LE products (ranging from 0.53 to 0.61) to 0.97 and decreased the RMSE (ranging from 26.37 to 33.17 W/m(2)) to 5.85 W/m(2). Compared with three other machine learning fusion models, Gradient Boosting Regression Tree (GBRT), Random Forest (RF), and Gaussian Process Regression (GPR), ETR exhibited the best performance in terms of both training and validation accuracy. We also applied the ETR fusion method to implement the mapping of average annual terrestrial LE over Europe at a resolution of 0.05 ? in the period from 2002 to 2005. When compared with global LE products such as the Global Land Surface Satellite (GLASS) and the Moderate Resolution Imaging Spectroradiometer (MODIS), the fusion LE using ETR exhibited a relatively small gap, which confirmed that it is reasonable and reliable for the estimation of the terrestrial LE over Europe.
更多查看译文
关键词
terrestrial latent heat flux,machine learning method,Europe
AI 理解论文
溯源树
样例
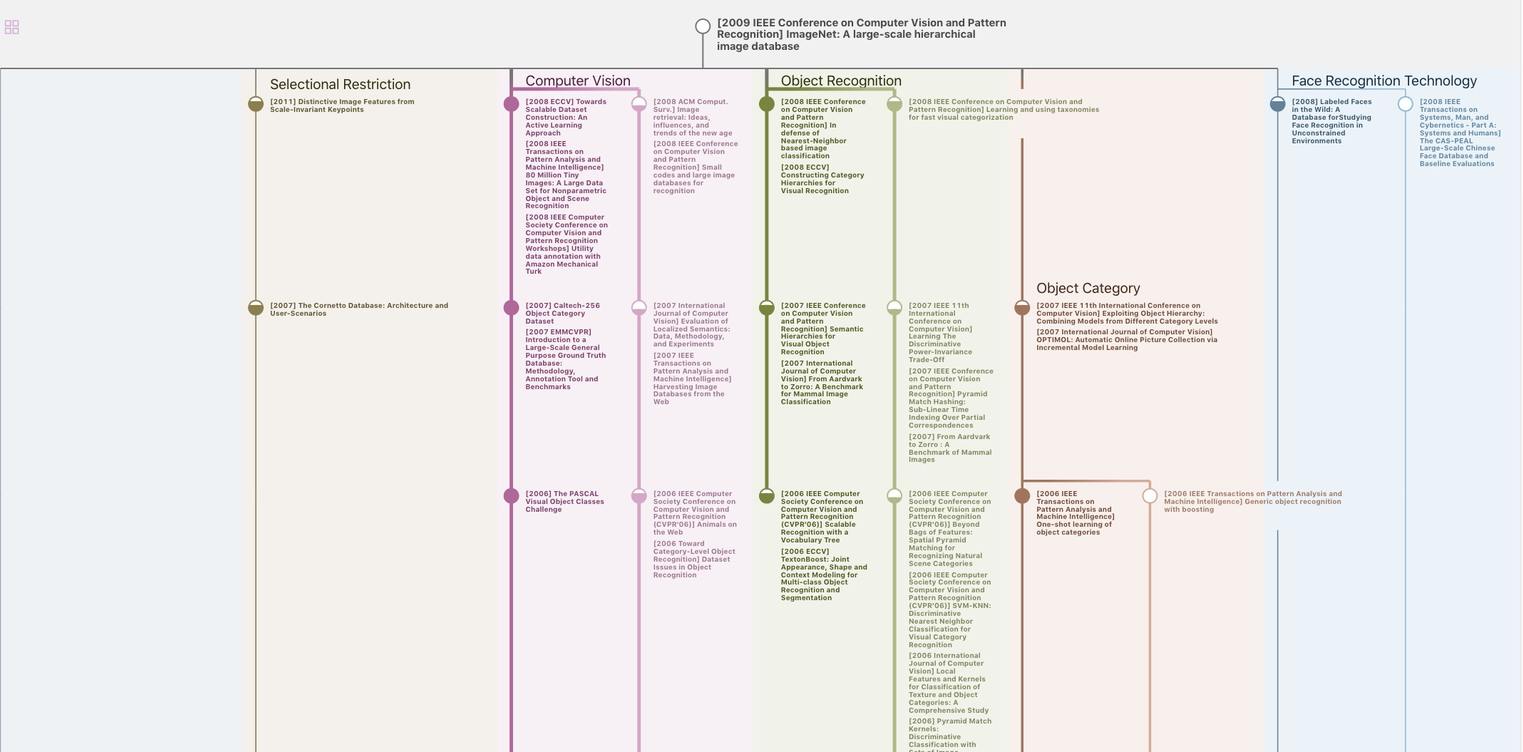
生成溯源树,研究论文发展脉络
Chat Paper
正在生成论文摘要