A novel unsupervised domain adaptation based on deep neural network and manifold regularization for mechanical fault diagnosis
MEASUREMENT SCIENCE AND TECHNOLOGY(2020)
摘要
Fault diagnosis plays an important role in modern rotating machinery. Recently, some deep neural network-based domain adaptation methods have been successfully applied in cross-domain fault diagnosis problems. However, most of these methods only reduce the distribution discrepancy between different domains, without considering the geometry differences and misalignments across different domains. To this end, a new domain adaptation approach based on a deep neural network is proposed for mechanical fault diagnosis. There are three main contributions in total for the proposed approach. (i) l(2,1)-norm based weight regularization is applied to reinforce the representative features of the original data. (ii) Manifold regularization is employed to further exploit the knowledge of marginal distributions and reduce the geometry differences between different domains. (iii) Subspace alignment is induced to reduce the misalignments of different domains. A gear dataset and a bearing dataset are selected to demonstrate the validity and robustness of the proposed approach. According to the experimental results, the proposed approach significantly outperforms the comparison approaches in mechanical fault diagnosis.
更多查看译文
关键词
unsupervised domain adaptation,deep neural network,manifold regularization,subspace alignment,fault detection
AI 理解论文
溯源树
样例
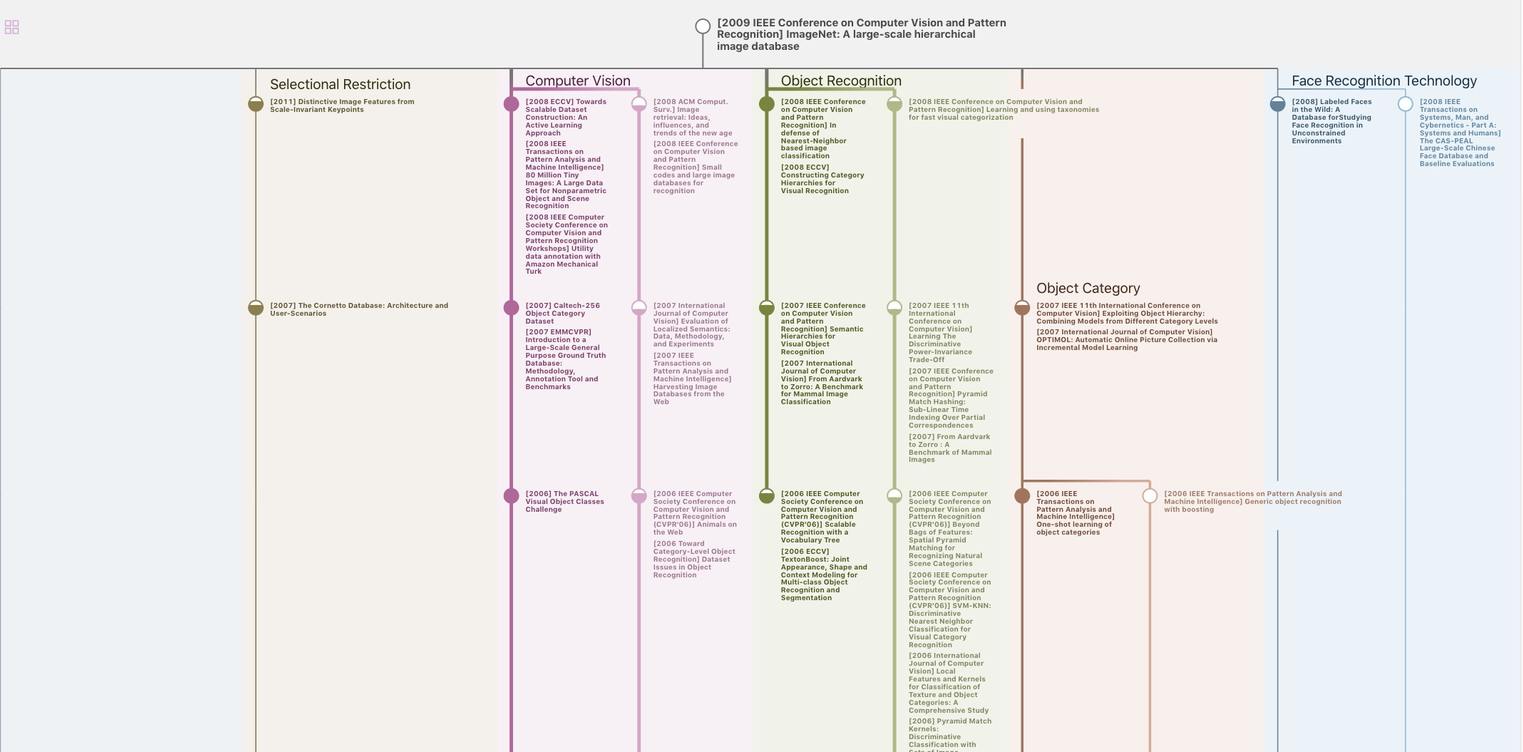
生成溯源树,研究论文发展脉络
Chat Paper
正在生成论文摘要