Arcing Fault Detection with Interpretable Learning Model Under the Integration of Renewable Energy
2019 North American Power Symposium (NAPS)(2019)
摘要
Under the trend of deeper renewable energy integration, active distribution networks are facing increasing uncertainty and security issues, among which the arcing fault detection (AFD) has baffled researchers for years. Existing machine learning based AFD methods are deficient in feature extraction and model interpretability. To overcome these limitations in learning algorithms, we have designed a way to translate the non-transparent machine learning prediction model into an implementable logic for AFD. Moreover, the AFD logic is tested under different fault scenarios and realistic renewable generation data, with the help of our self-developed AFD software. The performance from various tests shows that the interpretable prediction model has high accuracy, dependability, security and speed under the integration of renewable energy.
更多查看译文
关键词
Arcing Fault Detection,Distribution Networks,Power System Protection,Renewable Energy
AI 理解论文
溯源树
样例
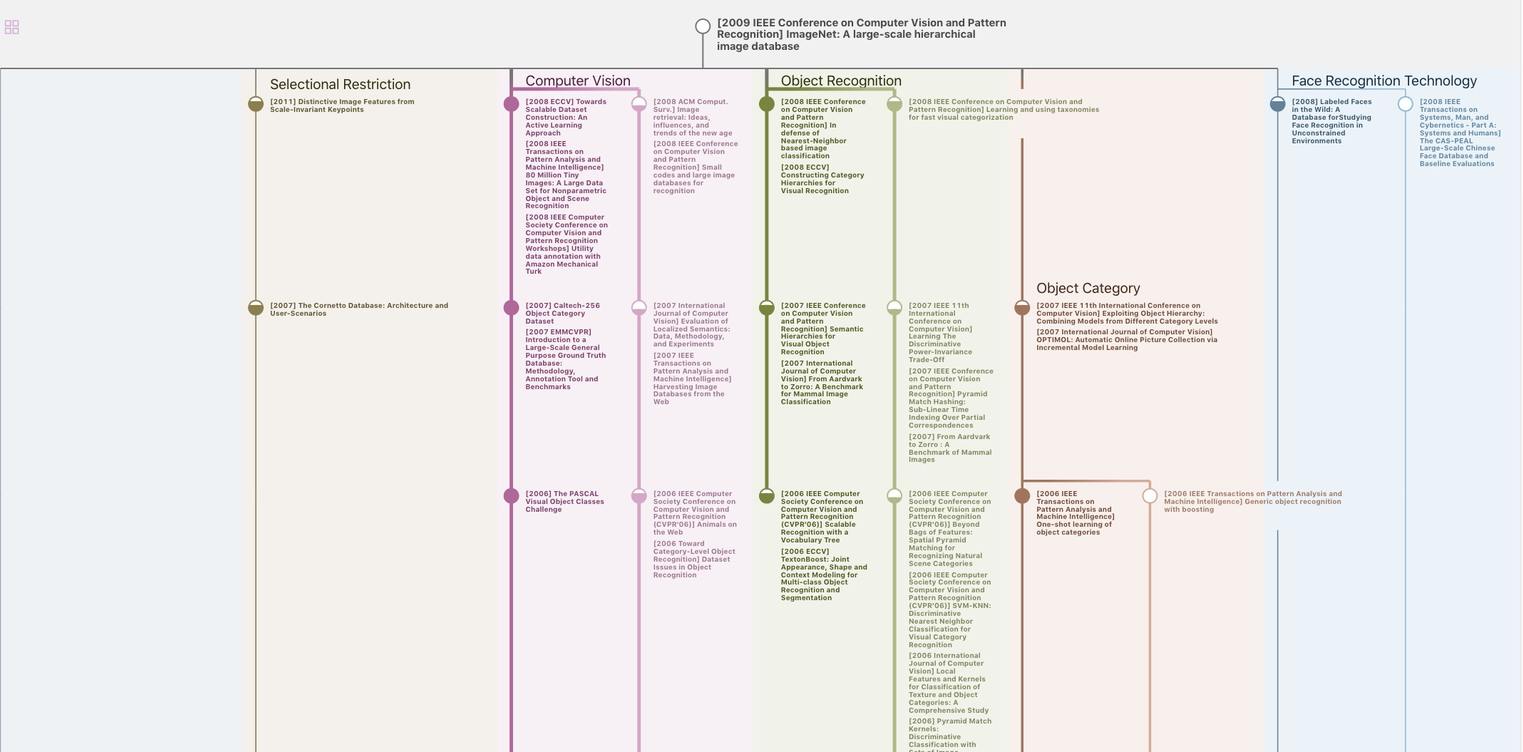
生成溯源树,研究论文发展脉络
Chat Paper
正在生成论文摘要