FairCharge: A Data-Driven Fairness-Aware Charging Recommendation System for Large-Scale Electric Taxi Fleets
Proceedings of the ACM on Interactive, Mobile, Wearable and Ubiquitous Technologies(2020)
摘要
Our society is witnessing a rapid taxi electrification process. Compared to conventional gas taxis, a key drawback of electric taxis is their prolonged charging time, which potentially reduces drivers' daily operation time and income. In addition, insufficient charging stations, intensive charging peaks, and heuristic-based charging station choice of drivers also significantly decrease the charging efficiency of electric taxi charging networks. To improve the charging efficiency (e.g., reduce queuing time in stations) of electric taxi charging networks, in this paper, we design a fairness-aware Pareto efficient charging recommendation system called FairCharge, which aims to minimize the total charging idle time (traveling time + queuing time) in a fleet-oriented fashion combined with fairness constraints. Different from existing works, FairCharge considers fairness as a constraint to potentially achieve long-term social benefits. In addition, our FairCharge considers not only current charging requests, but also possible charging requests of other nearby electric taxis in a near-future duration. More importantly, we simulate and evaluate FairCharge with real-world streaming data from the Chinese city Shenzhen, including GPS data and transaction data from more than 16,400 electric taxis, coupled with the data of 117 charging stations, which constitute, to our knowledge, the largest electric taxi network in the world. The extensive experimental results show that our fairness-aware FairCharge effectively reduces queuing time and idle time of the Shenzhen electric taxi fleet by 80.2% and 67.7%, simultaneously.
更多查看译文
关键词
Electric taxi,Pareto efficiency,charging recommendation,fairness-aware,recommendation system
AI 理解论文
溯源树
样例
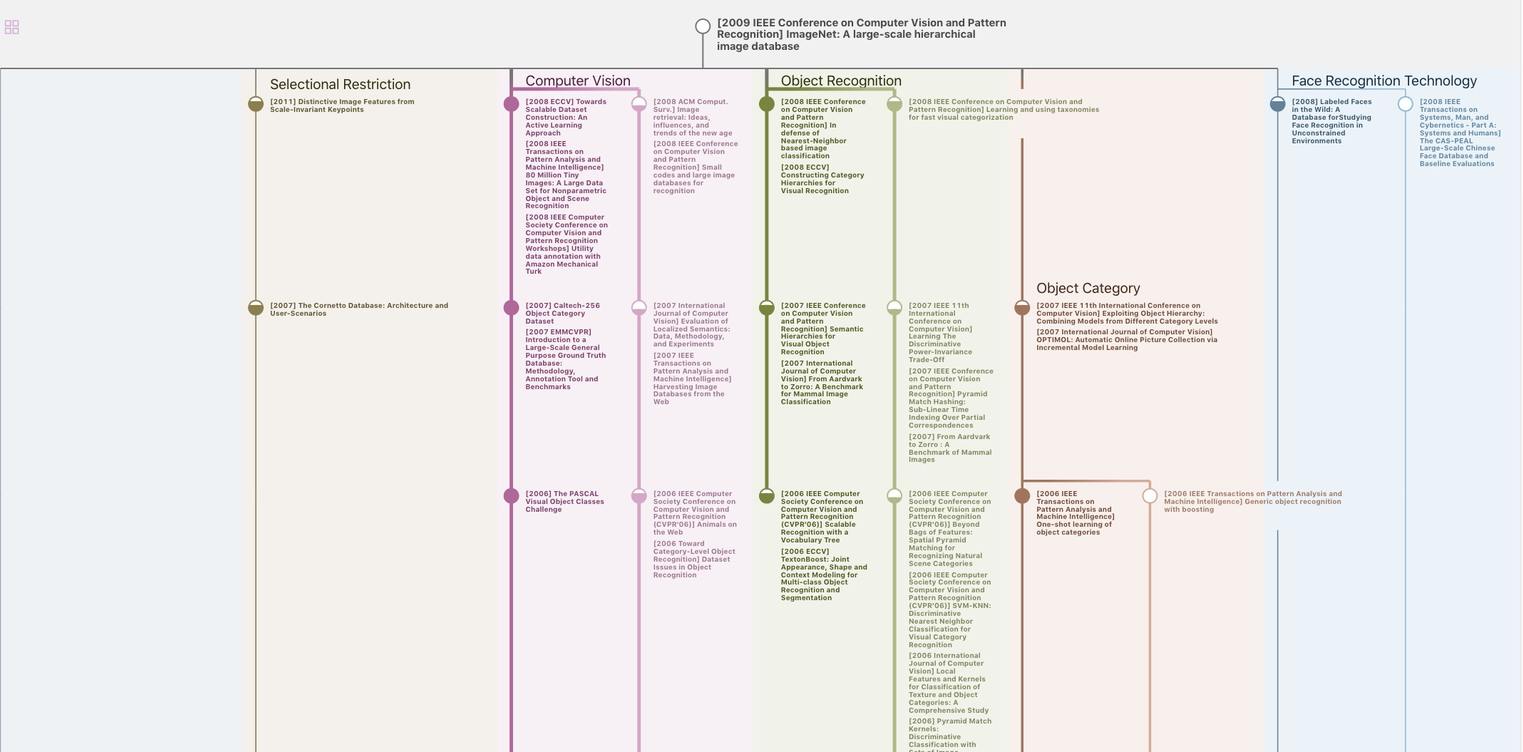
生成溯源树,研究论文发展脉络
Chat Paper
正在生成论文摘要