Federated Visual Classification with Real-World Data Distribution
European Conference on Computer Vision(2020)
摘要
Federated Learning enables visual models to be trained on-device, bringing advantages for user privacy (data need never leave the device), but challenges in terms of data diversity and quality. Whilst typical models in the datacenter are trained using data that are independent and identically distributed (IID), data at source are typically far from IID. Furthermore, differing quantities of data are typically available at each device (imbalance). In this work, we characterize the effect these real-world data distributions have on distributed learning, using as a benchmark the standard Federated Averaging (FedAvg) algorithm. To do so, we introduce two new large-scale datasets for species and landmark classification, with realistic per-user data splits that simulate real-world edge learning scenarios. We also develop two new algorithms (FedVC, FedIR) that intelligently resample and reweight over the client pool, bringing large improvements in accuracy and stability in training. The datasets are made available online.
更多查看译文
关键词
federated visual classification,real-world real-world
AI 理解论文
溯源树
样例
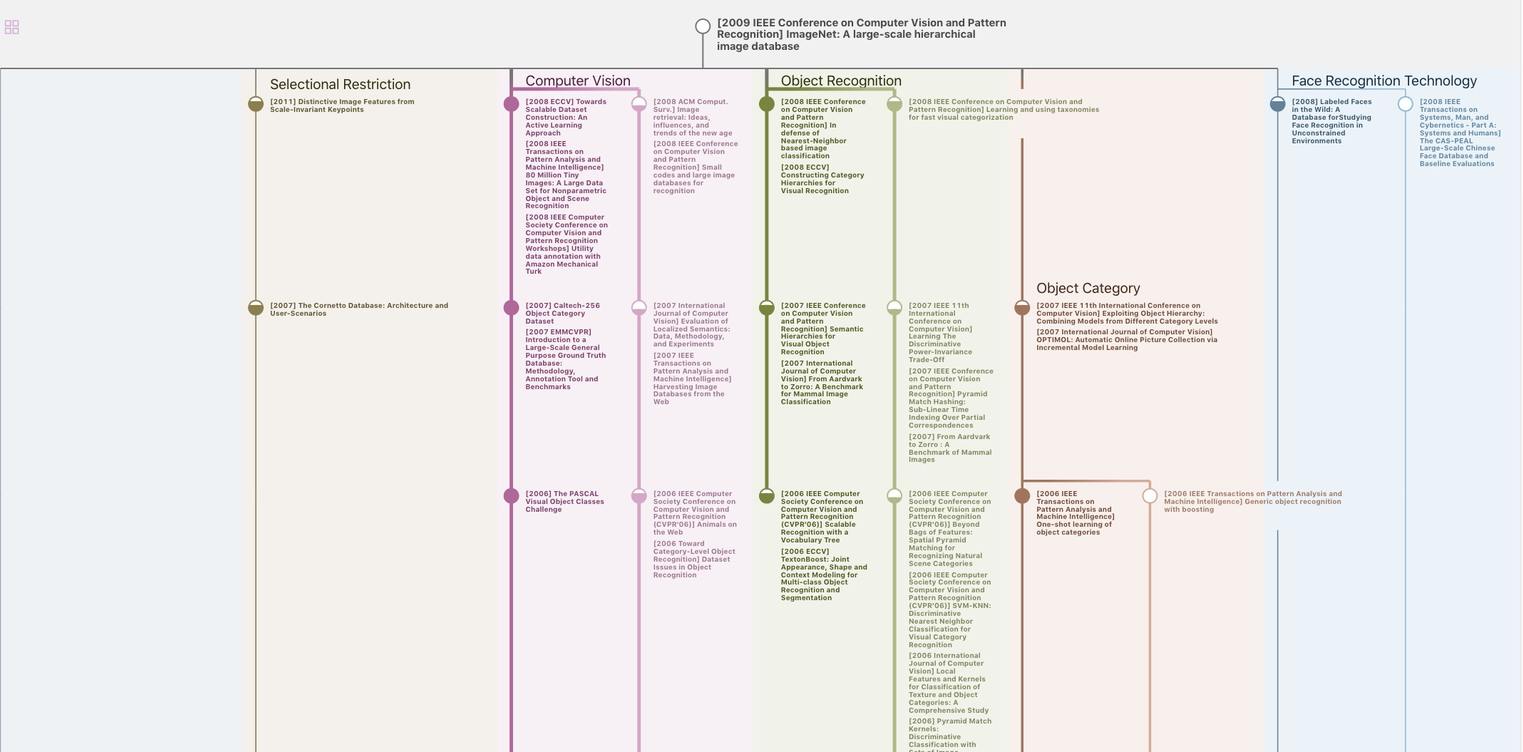
生成溯源树,研究论文发展脉络
Chat Paper
正在生成论文摘要