Warder: Online Insider Threat Detection System Using Multi-Feature Modeling and Graph-Based Correlation
MILCOM 2019 - 2019 IEEE Military Communications Conference (MILCOM)(2019)
摘要
Existing insider threat detection models and frameworks generally focus on characterizing and detecting malicious insiders, for example by fusing behavioral analysis, machine learning, psychological characters, management measures, etc. However, it remains challenging to design a practical insider threat detection scheme that can be efficiently implemented and deployed in a real-world system. For example, existing approaches focus on extracting features from user behavioral activities but they lack in-depth correlation and decision making for suspected alerts; thus, resulting in high false positives and low detection accuracy. In this work, we propose a novel online insider threat detection system, Warder, which leverages diverse feature dimensions (using neural language processing) and fuses content and behavior features to create a user's daily profile to facilitate threat detection. Besides, hypergraph-based threat scenario feature tree is designed to correlate suspicious users' activities with threat scenarios to further screen the users. In practice, Warder can also be constantly updated using newly discovered features and threat scenarios. We evaluate the performance of Warder using the public CMU CERT dataset, as well as that of approaches from the Oxford group and CMU group. Findings from the evaluation demonstrate that Warder outperforms the other two competing approaches.
更多查看译文
关键词
anomaly detection,insider threat detection,hypergraph,online activity
AI 理解论文
溯源树
样例
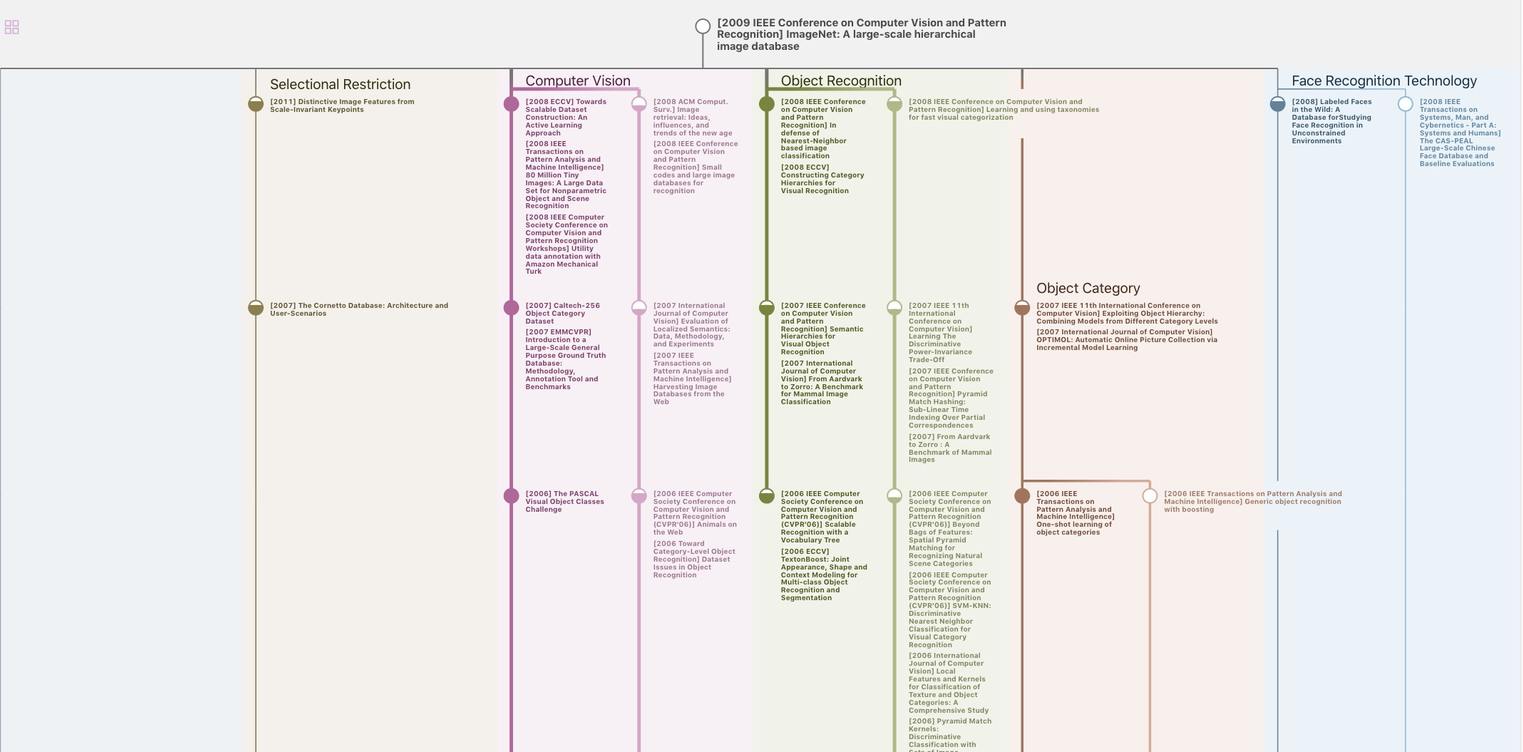
生成溯源树,研究论文发展脉络
Chat Paper
正在生成论文摘要