Cross-Modal Deep Face Normals With Deactivable Skip Connections
2020 IEEE/CVF CONFERENCE ON COMPUTER VISION AND PATTERN RECOGNITION (CVPR)(2020)
摘要
We present an approach for estimating surface normals from in-the-wild color images of faces. While data-driven strategies have been proposed for single face images, limited available ground truth data makes this problem difficult. To alleviate this issue, we propose a method that can leverage all available image and normal data, whether paired or not, thanks to a novel cross-modal learning architecture. In particular, we enable additional training with single modality data, either color or normal, by using two encoder-decoder networks with a shared latent space. The proposed architecture also enables face details to be transferred between the image and normal domains, given paired data, through skip connections between the image encoder and normal decoder. Core to our approach is a novel module that we call deactivable skip connections, which allows integrating both the auto-encoded and image-to-normal branches within the same architecture that can be trained end-to-end. This allows learning of a rich latent space that can accurately capture the normal information. We compare against state-of-the-art methods and show that our approach can achieve significant improvements, both quantitative and qualitative, with natural face images.
更多查看译文
关键词
in-the-wild color images,cross-modal learning architecture,latent space,auto-encoded branches,encoder-decoder networks,single modality data,normal data,ground truth data,single face images,data-driven strategies,surface normals,cross-modal deep face normals,natural face images,normal information,image-to-normal branches,deactivable skip connections,normal decoder,image encoder
AI 理解论文
溯源树
样例
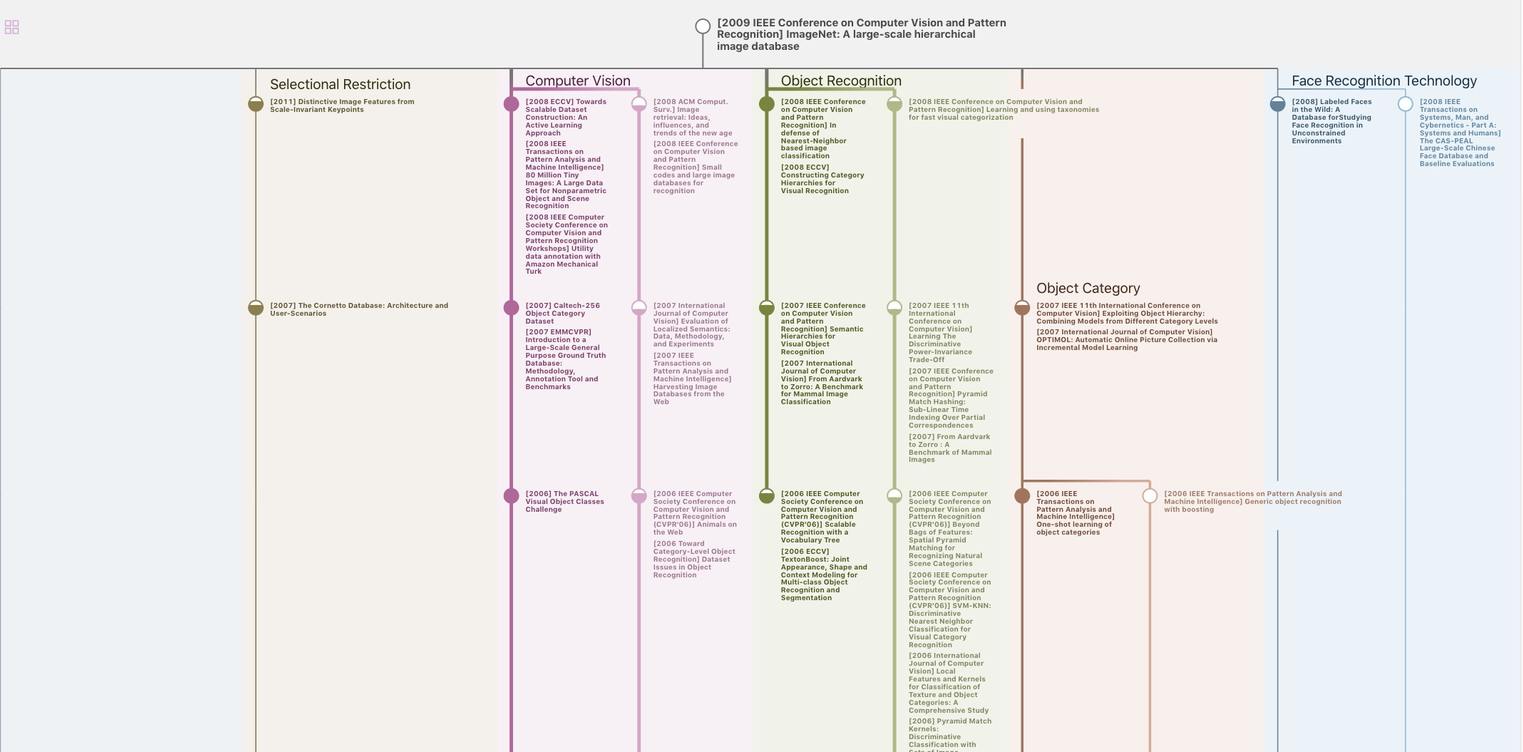
生成溯源树,研究论文发展脉络
Chat Paper
正在生成论文摘要