A multi-quantile regression time series model with interquantile lipschitz regularization for wind power probabilistic forecasting
ELECTRIC POWER SYSTEMS RESEARCH(2022)
摘要
Modern decision-making processes require uncertainty-aware models, especially those relying on non-symmetric costs and risk-averse profiles. The objective of this work is to propose a dynamic model for the conditional nonparametric distribution function (CDF) to generate probabilistic forecasts for a renewable generation time series. To do that, we propose an adaptive non-parametric time-series model driven by a regularized multiple-quantileregression (MQR) framework. In our approach, all regression models are jointly estimated through a single linear optimization problem that finds the global-optimal parameters in polynomial time. An innovative feature of our work is the consideration of a Lipschitz regularization of the first derivative of coefficients in the quantile space, which imposes coefficient smoothness. The proposed regularization induces a coupling effect among quantiles creating a single non-parametric CDF model with improved out-of-sample performance. A case study with realistic wind-power generation data from the Brazilian system shows: 1) the regularization model is capable to improve the performance of MQR probabilistic forecasts, and 2) our MQR model outperforms five relevant benchmarks: two based on the MQR framework, and three based on parametric models, namely, SARIMA, and GAS with Beta and Weibull CDF.
更多查看译文
关键词
Least absolute shrinkage and selection operator, Lipschitz regularization, Multi-quantile regression, Non-parametric time series, Probabilistic forecast, Renewable generation forecast
AI 理解论文
溯源树
样例
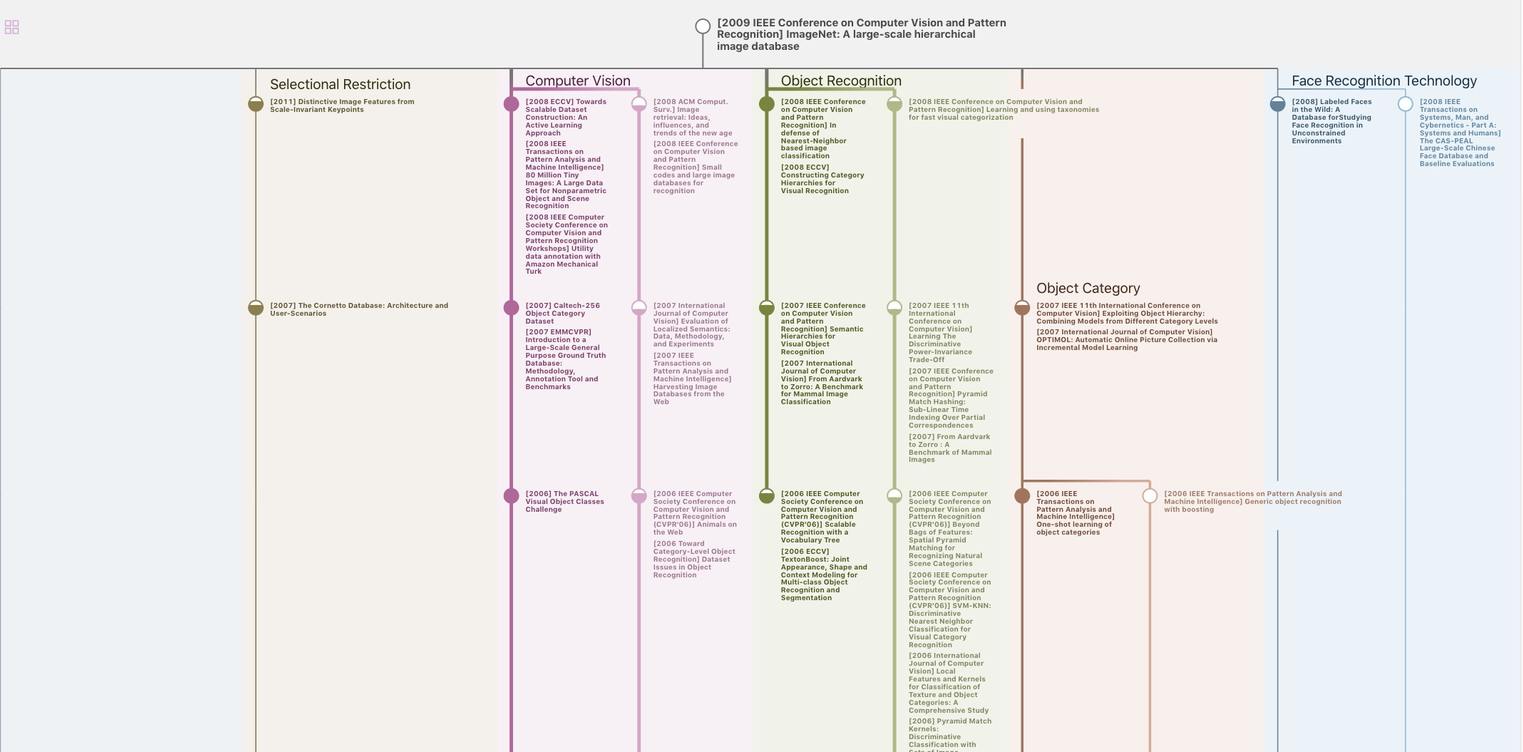
生成溯源树,研究论文发展脉络
Chat Paper
正在生成论文摘要