A Time Series Approach To Player Churn And Conversion In Videogames
INTELLIGENT DATA ANALYSIS(2021)
摘要
Players of a free-to-play game are divided into three main groups: non-paying active users, paying active users and inactive users. A State Space time series approach is then used to model the daily conversion rates between the different groups, i.e., the probability of transitioning from one group to another. This allows, not only for predictions on how these rates are to evolve, but also for a deeper understanding of the impact that in-game planning and calendar effects have. It is also used in this work for the detection of marketing and promotion campaigns about which no information is available. In particular, two different State Space formulations are considered and compared: an Autoregressive Integrated Moving Average process and an Unobserved Components approach, in both cases with a linear regression to explanatory variables. Both yield very close estimations for covariate parameters, producing forecasts with similar performances for most transition rates. While the Unobserved Components approach is more robust and needs less human intervention in regards to model definition, it produces significantly worse forecasts for non-paying user abandonment probability. More critically, it also fails to detect a plausible marketing and promotion campaign scenario.
更多查看译文
关键词
Time series, state space models, videogames, ARIMA, structural time series
AI 理解论文
溯源树
样例
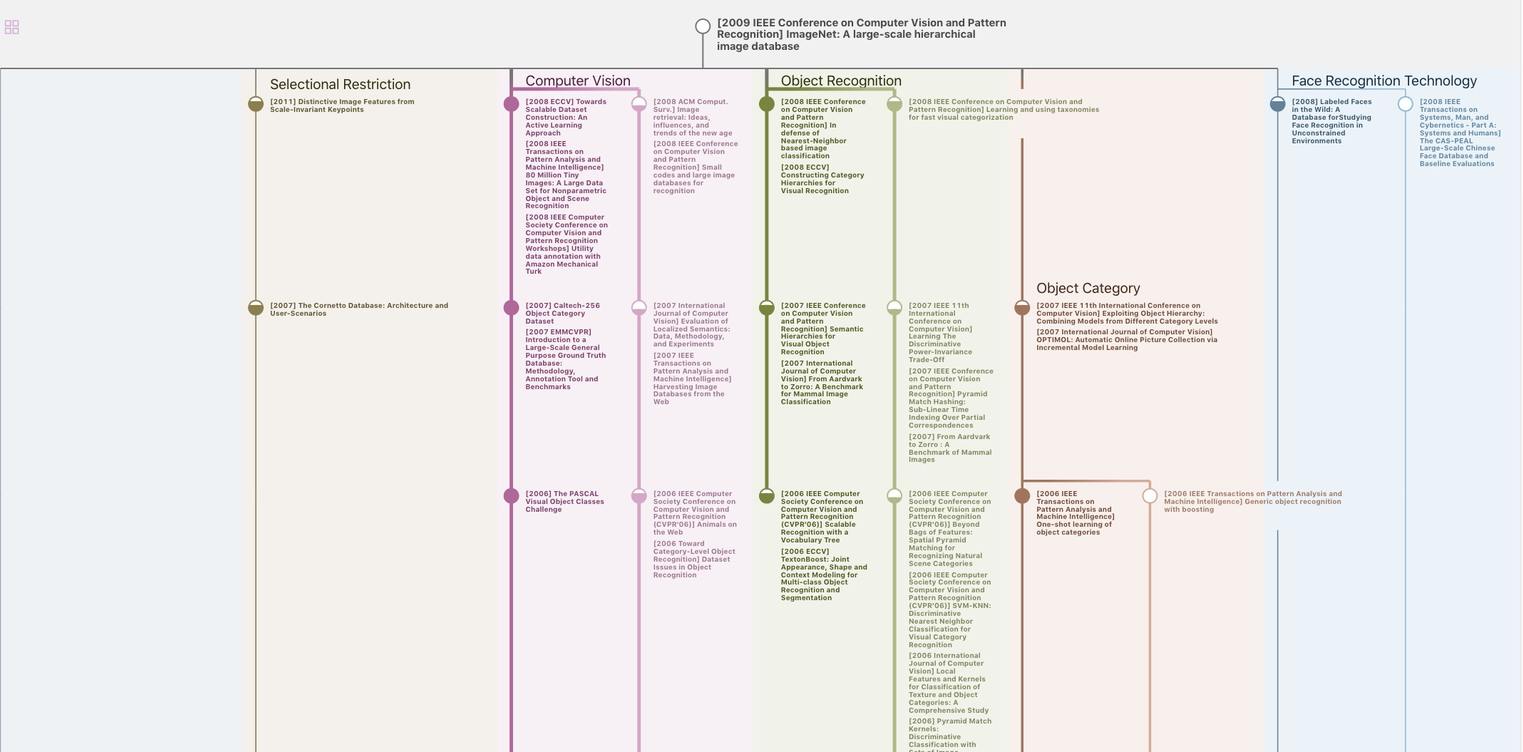
生成溯源树,研究论文发展脉络
Chat Paper
正在生成论文摘要