A Version Space Perspective on Differentially Private Pool-Based Active Learning
2019 IEEE International Workshop on Information Forensics and Security (WIFS)(2019)
摘要
We analyze pool-based active learning under a differential privacy guarantee. At every active learning step, some samples are selected to be labeled by an oracle, and the new labels are used to update the classifier. We want to preserve differential privacy during both the sample selection step and the classifier update step. To study the evolution of the active learner, we use the concept of a version space of possible hypotheses (classifiers). This concept helps establish a principled notion of the informativeness of a pool sample: When informative samples are labeled and used for training, the version space shrinks, yielding classifiers consistent with the labeled samples. To provide privacy, we query the oracle with both informative and non-informative samples using a simple randomized sampling scheme. We prove the privacy guarantee, and characterize the increase in label complexity resulting from our randomized sampling strategy. To examine how our theoretical analysis manifests in practice, we built an SVM-based active learner, and measured the accuracy and label complexity achieved with and without privacy.
更多查看译文
关键词
version space perspective,oracle,informative samples,noninformative samples,label complexity,randomized sampling strategy,SVM-based active learner,differential private pool-based active learning
AI 理解论文
溯源树
样例
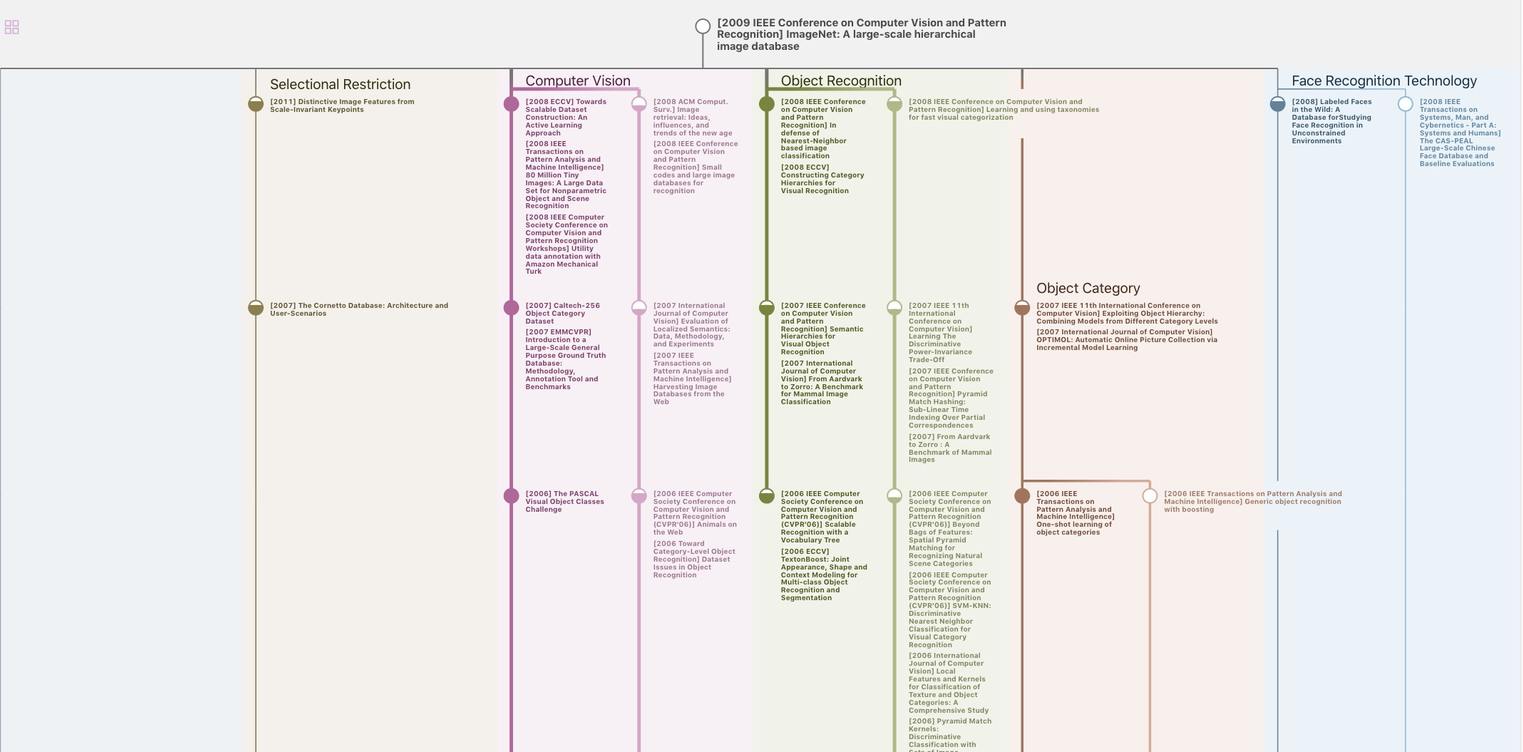
生成溯源树,研究论文发展脉络
Chat Paper
正在生成论文摘要