Predicting Biker Density at Bikeshare Station Intersections in San Francisco
2019 IEEE Global Humanitarian Technology Conference (GHTC)(2019)
摘要
Bike sharing platforms are becoming increasingly common alternatives to public transportation in cities, improving accessibility to areas not reachable by bus, train, or tram. While this can be beneficial for improving city connectivity, it also increases the likelihood of biker related accidents and vehicle collisions, especially in areas where protected bike lanes and safety infrastructure are not already in place. We compare machine learning models to predict biker density at road intersections in the city of San Francisco, using publicly available trip data from the city's most widely used bikeshare service, Ford GoBike, evaluating our model performance by monitoring mean squared error. Alongside our predictive models we develop a heatmap visualization application to display our predictions, providing an additional mode of interaction for users to access the forecasted information. The intended usage of our work is to predict areas of highest biker density at different times so that drivers and bikers can experience improved shared road safety. The deployment of our models can also inform city planning and alternative public transportation development.
更多查看译文
关键词
machine learning,prediction methods,neural networks,smart transportation,public transportation,bicycles,user interfaces,visualization
AI 理解论文
溯源树
样例
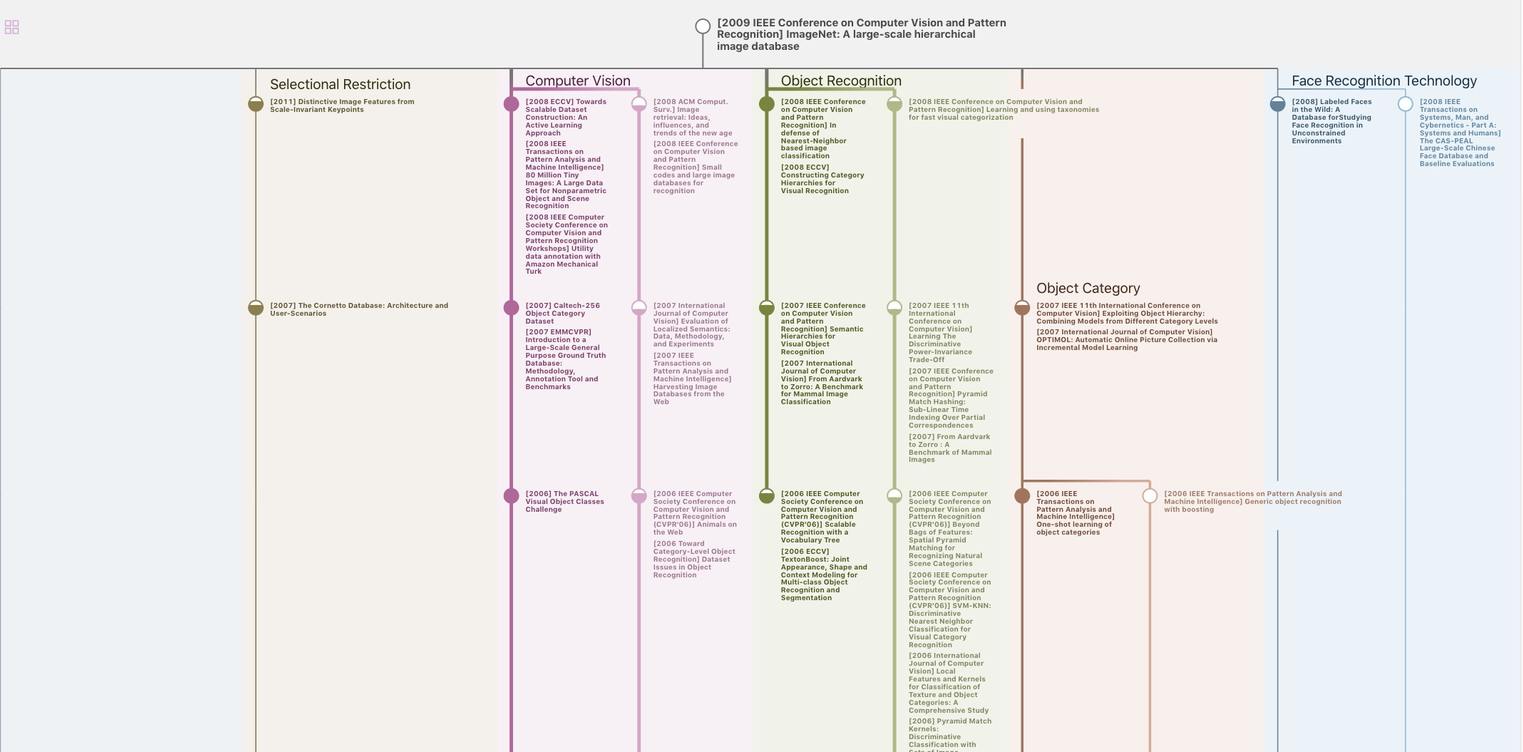
生成溯源树,研究论文发展脉络
Chat Paper
正在生成论文摘要