A Novel Stratification Framework for Predicting Outcome in Patients with Prostate Cancer
British journal of cancer(2020)
摘要
Background Unsupervised learning methods, such as Hierarchical Cluster Analysis, are commonly used for the analysis of genomic platform data. Unfortunately, such approaches ignore the well-documented heterogeneous composition of prostate cancer samples. Our aim is to use more sophisticated analytical approaches to deconvolute the structure of prostate cancer transcriptome data, providing novel clinically actionable information for this disease. Methods We apply an unsupervised model called Latent Process Decomposition (LPD), which can handle heterogeneity within individual cancer samples, to genome-wide expression data from eight prostate cancer clinical series, including 1,785 malignant samples with the clinical endpoints of PSA failure and metastasis. Results We show that PSA failure is correlated with the level of an expression signature called DESNT (HR = 1.52, 95% CI = [1.36, 1.7], P = 9.0 × 10 −14 , Cox model), and that patients with a majority DESNT signature have an increased metastatic risk ( X 2 test, P = 0.0017, and P = 0.0019). In addition, we develop a stratification framework that incorporates DESNT and identifies three novel molecular subtypes of prostate cancer. Conclusions These results highlight the importance of using more complex approaches for the analysis of genomic data, may assist drug targeting, and have allowed the construction of a nomogram combining DESNT with other clinical factors for use in clinical management.
更多查看译文
关键词
Computer science,Molecular medicine,Prostate cancer,Biomedicine,general,Cancer Research,Epidemiology,Molecular Medicine,Oncology,Drug Resistance
AI 理解论文
溯源树
样例
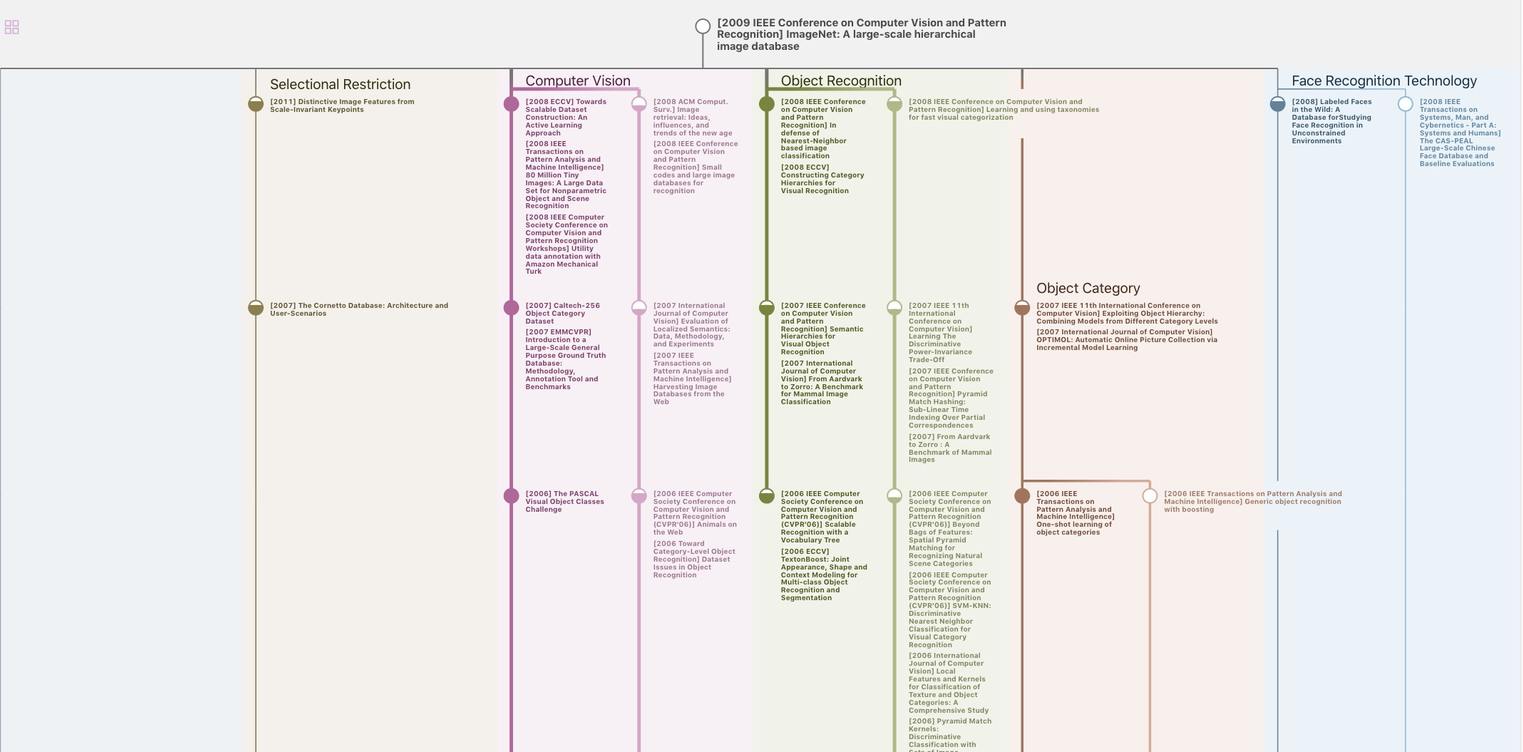
生成溯源树,研究论文发展脉络
Chat Paper
正在生成论文摘要