Too many cooks: Coordinating multi-agent collaboration through inverse planning
AAMAS '19: International Conference on Autonomous Agents and Multiagent Systems Auckland New Zealand May, 2020(2020)
摘要
Humans collaborate in dynamic and flexible ways. Collaboration requires agents to coordinate their behavior on the fly, sometimes jointly solving a single task together and other times dividing it up into sub-tasks to work on in parallel. We develop Bayesian Delegation, a learning mechanism for decentralized multi-agent coordination that enables agents to rapidly infer the sub-tasks that other agents are working on by inverse planning. These inferences enable agents to determine, in the absence of communication, whether to plan jointly with others or work on complementary sub-tasks. We test this model in a suite of decentralized multi-agent environments inspired by cooking problems. To succeed, agents must coordinate both their high-level plans (sub-task) and their low-level actions (avoiding collisions). Including joint sub-tasks in the prior of Bayesian delegation enables agents to carry out sub-tasks that neither agent can finish independently. The full system outperforms lesioned systems that are missing one or more of these capabilities.
更多查看译文
AI 理解论文
溯源树
样例
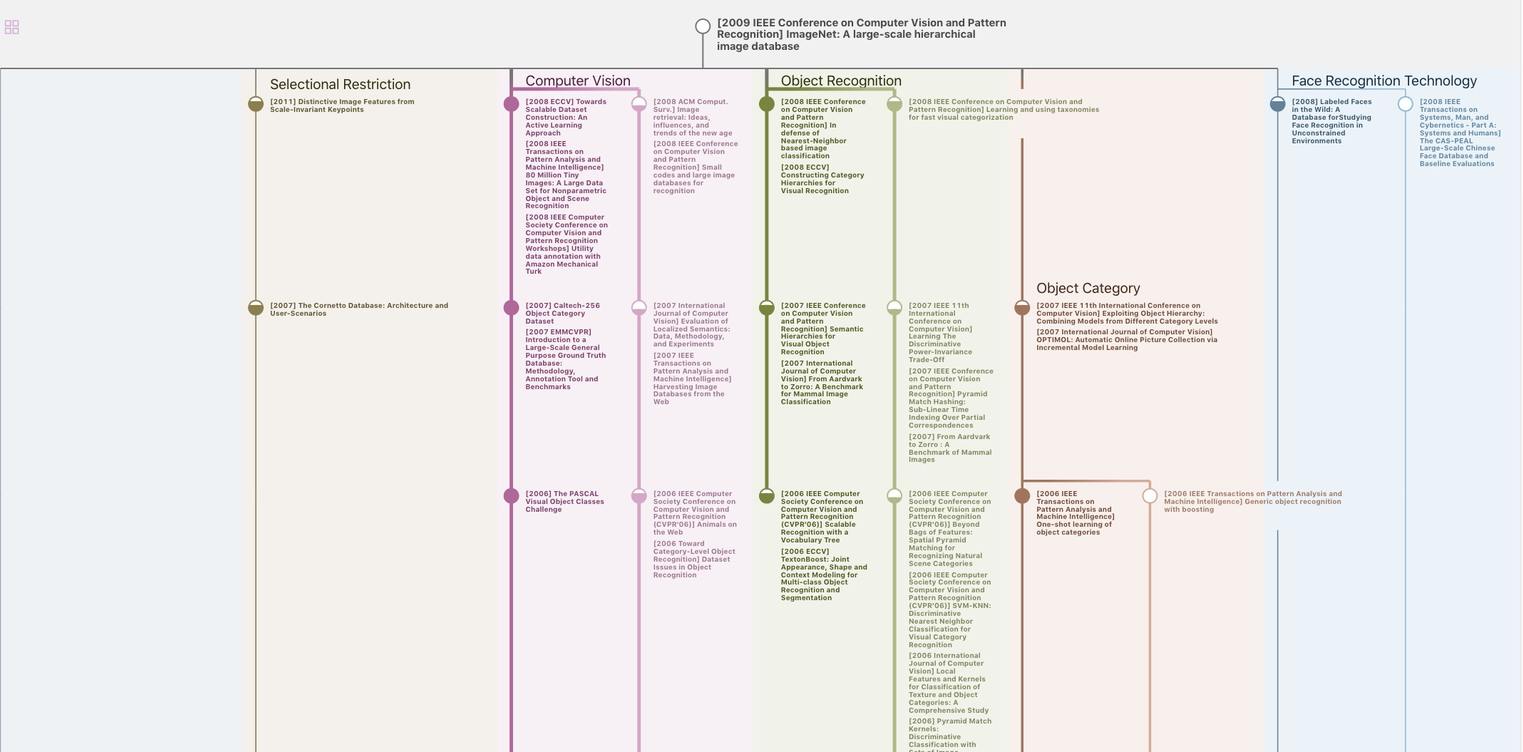
生成溯源树,研究论文发展脉络
Chat Paper
正在生成论文摘要