Robust Least Squares for Quantized Data
SIGNAL PROCESSING(2020)
摘要
In this paper we formulate and solve a robust least squares problem for a system of linear equations subject to quantization error. Ordinary least squares fails to consider uncertainty in the data matrices, modeling all noise in the observed signal. Total least squares accounts for uncertainty in the data matrix, but necessarily increases the condition number of the system compared to ordinary least squares. Tikhonov regularization or ridge regression, is frequently employed to combat ill-conditioning, but requires heuristic parameter tuning which presents a host of challenges and places strong assumptions on parameter prior distributions. The proposed method also requires selection of a parameter, but it can be chosen in a natural way, e.g., a matrix rounded to the 4th digit uses an uncertainty bounding parameter of 0.5e-4. We show here that our robust method is theoretically appropriate, tractable, and performs favorably against ordinary and total least squares for both residual and absolute error reduction.
更多查看译文
关键词
Least squares approximation,Quantization error,Ridge regression,Robust optimization,Subgradient methods,Tikhonov regularization,Total least squares
AI 理解论文
溯源树
样例
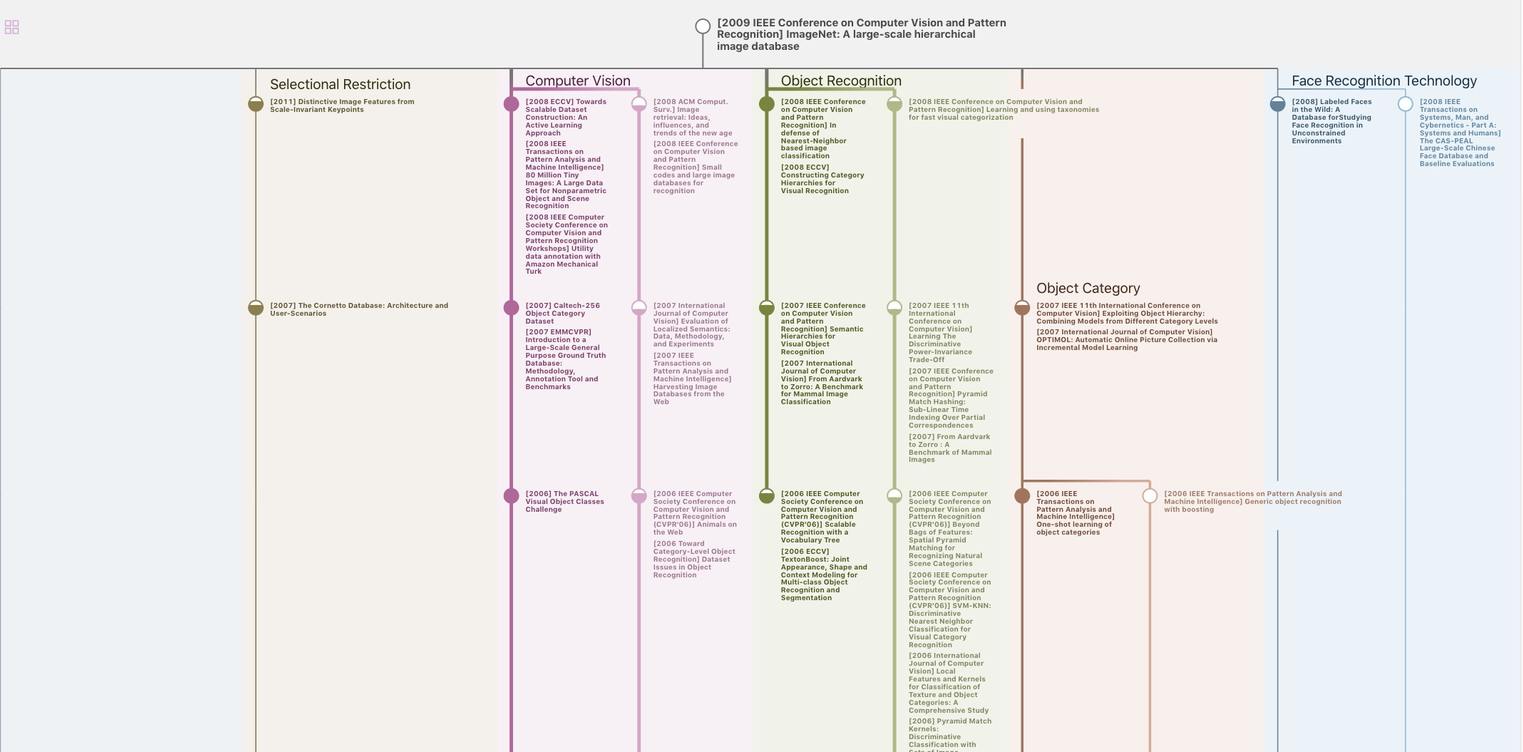
生成溯源树,研究论文发展脉络
Chat Paper
正在生成论文摘要