Towards Discriminability And Diversity: Batch Nuclear-Norm Maximization Under Label Insufficient Situations
2020 IEEE/CVF CONFERENCE ON COMPUTER VISION AND PATTERN RECOGNITION (CVPR)(2020)
摘要
The learning of the deep networks largely relies on the data with human-annotated labels. In some label insufficient situations, the performance degrades on the decision boundary with high data density. A common solution is to directly minimize the Shannon Entropy, but the side effect caused by entropy minimization, i.e., reduction of the prediction diversity, is mostly ignored. To address this issue, we reinvestigate the structure of classification output matrix of a randomly selected data batch. We find by theoretical analysis that the prediction discriminability and diversity could be separately measured by the Frobeniusnorm and rank of the batch output matrix. Besides, the nuclear-norm is an upperbound of the Frobenius-norm, and a convex approximation of the matrix rank. Accordingly, to improve both discriminability and diversity, we propose Batch Nuclear-norm Maximization (BNM) on the output matrix. BNM could boost the learning under typical label insufficient learning scenarios, such as semi-supervised learning, domain adaptation and open domain recognition. On these tasks, extensive experimental results show that BNM outperforms competitors and works well with existing well-known methods. The code is available at https://github.com/cuishuhao/BNM.
更多查看译文
关键词
batch nuclear-norm maximization,Shannon entropy minimisation,semisupervised learning,BNM,matrix rank,batch output matrix,Frobenius-norm,prediction discriminability,randomly selected data batch,classification output matrix,prediction diversity,entropy minimization,data density,decision boundary,human-annotated labels,deep network learning,label insufficient situations
AI 理解论文
溯源树
样例
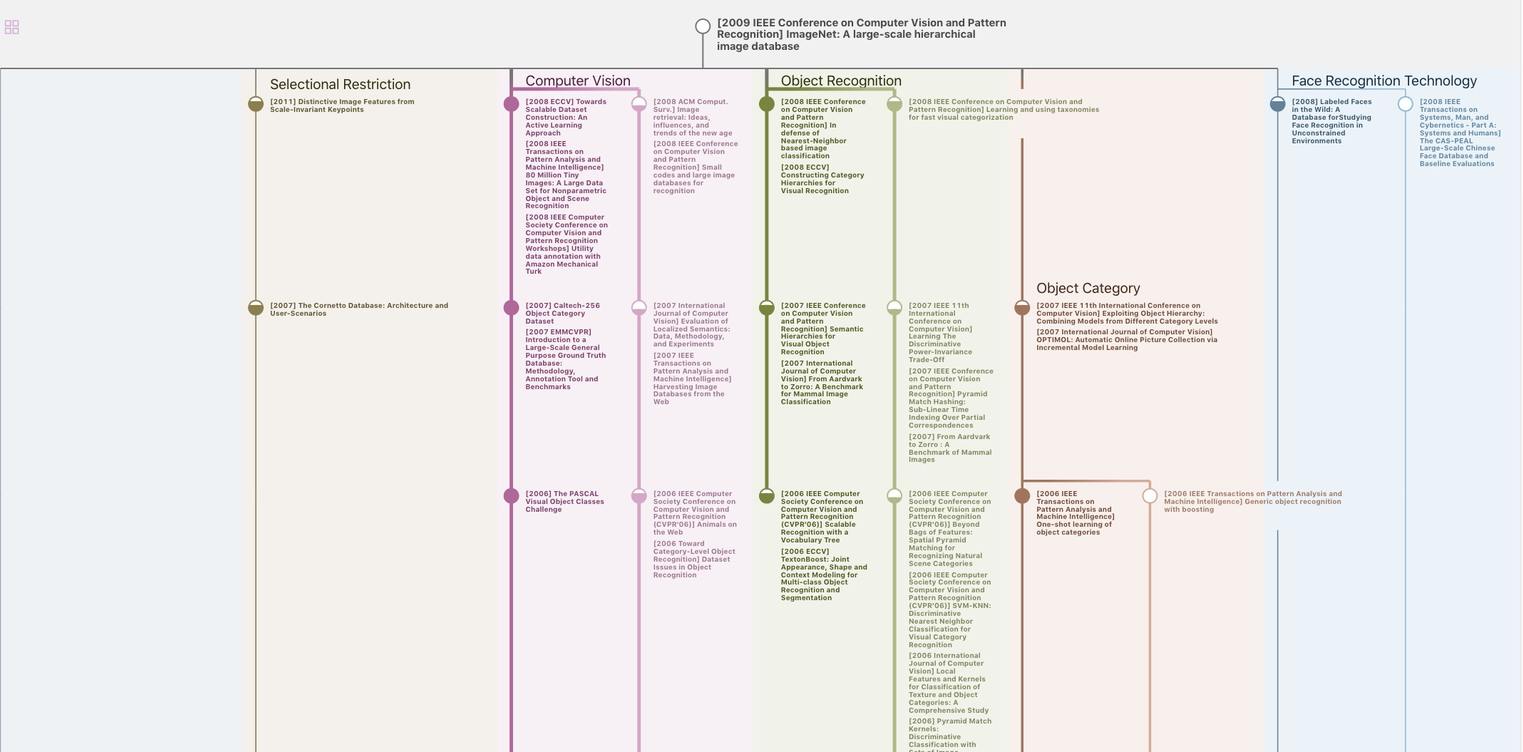
生成溯源树,研究论文发展脉络
Chat Paper
正在生成论文摘要