Empowering personalized feedback on hot water usage: a field study with shower meters
SAC '20: The 35th ACM/SIGAPP Symposium on Applied Computing Brno Czech Republic March, 2020(2020)
摘要
Digitalization enables an ever-increasing opportunity to promote resource conservation by providing timely consumption feedback to individuals. Yet, in multi-person households, appliances and fixtures are often shared, which makes it difficult to deliver person-specific feedback for each user. In this paper, we tackle this problem for household's most energy-intensive day-to-day activity - showering - by leveraging granular water consumption data. To this end, we collected labeled time series data of 691 shower events from 28 individuals, defined features, implemented and adjusted several classifiers, and analyzed the feasibility of our identification approach. Across all locations, the results - which were evaluated with stratified five-fold cross validations - provide robust evidence that the presented approach can indeed identify users reliably immediately after the end of a shower event. More specifically, the classifier with the best overall performance (Random Forest) achieved an average accuracy of 83.2% even for the most challenging environment in our field test (differentiating between five individuals in a company shower) and reached an average accuracy of 98.8% for a two-person household. Moreover, the approach requires only little training data for a satisfactory performance.
更多查看译文
关键词
User Identification, Personalized Feedback, Hot Water Consumption, Machine Learning
AI 理解论文
溯源树
样例
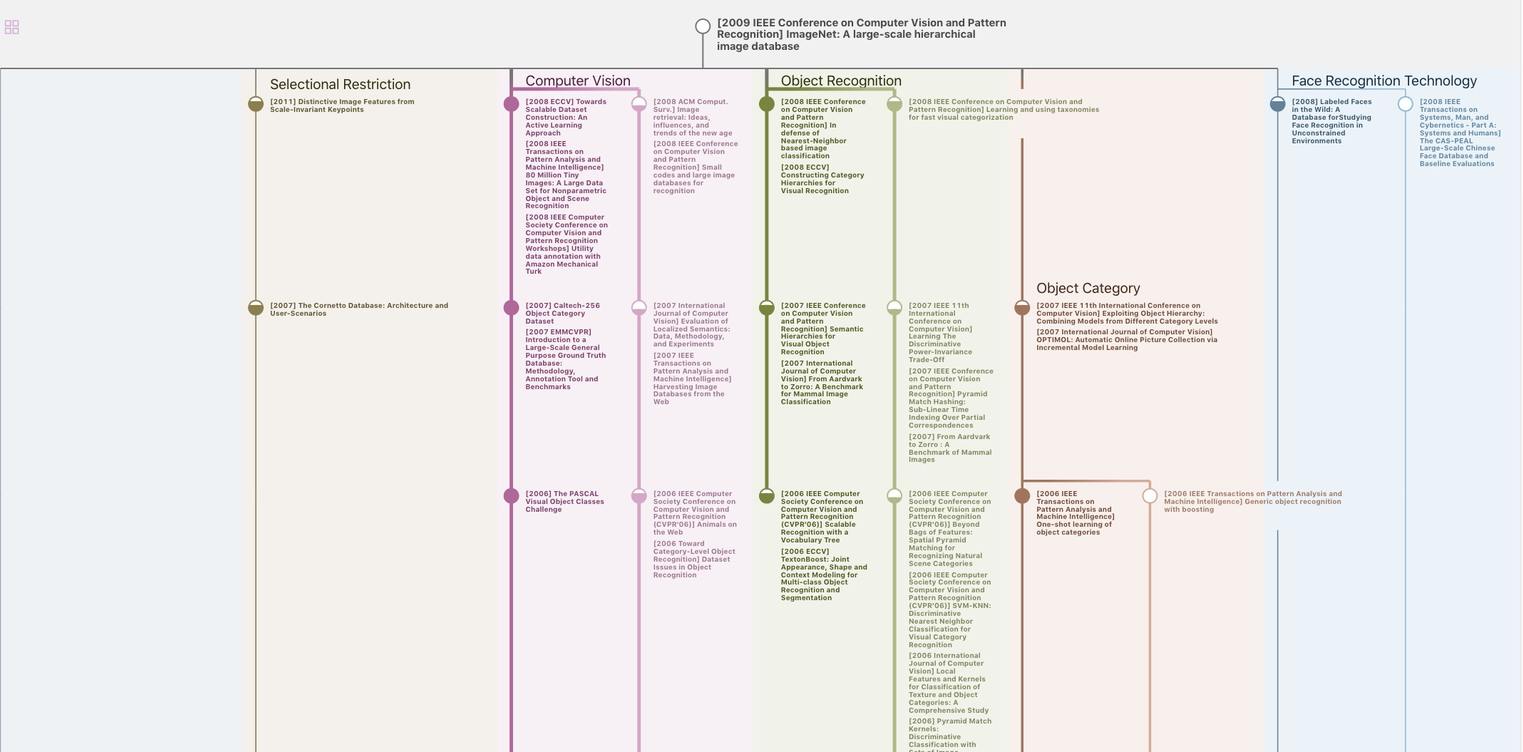
生成溯源树,研究论文发展脉络
Chat Paper
正在生成论文摘要