Aspect level sentiment classification with unbiased attention and target enhanced representations
SAC '20: The 35th ACM/SIGAPP Symposium on Applied Computing Brno Czech Republic March, 2020(2020)
摘要
Aspect-level sentiment classification aims at inferring the sentiment polarities of opinion targets for a given sentence. As a sentence might contain multiple sentiment-target pairs, extracting relevant information concerning the given target entity is the main challenge of this task. We try to overcome the challenge from two aspects. First, the attention mechanism is able to focus on the relevant part of the given entity and is well suited for this task. However, previous attention-based models still suffer from the problem of paying too much attention to some sentiment words that are irrelevant to the target. We call this as attention bias problem. To alleviate the biases, in this work, we introduce an adversarial training method to get unbiased attention. Second, we try to enhance the impact of the target from the perspective of word representations. Thus we propose an Embedding-Preserving Gating (EPGating) Mechanism. The mechanism dynamically incorporates target-related features into word representations as well as retains original word information. The experimental results on SemEval datasets demonstrate the effectiveness of our model.
更多查看译文
关键词
Aspect-based sentiment classification, Attention bias, Adversarial training, Gating mechanism
AI 理解论文
溯源树
样例
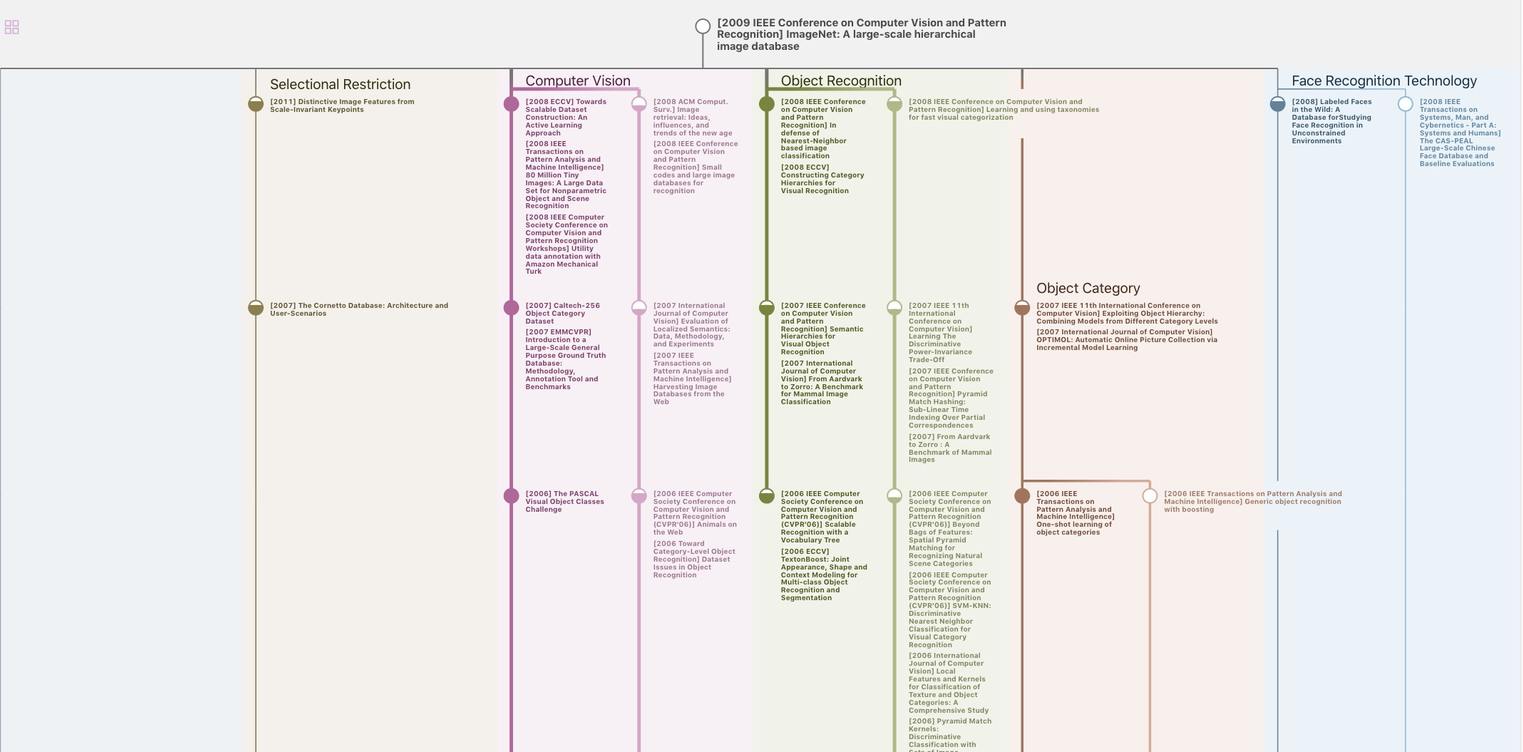
生成溯源树,研究论文发展脉络
Chat Paper
正在生成论文摘要