Filtering enhanced tomographic PIV reconstruction based on deep neural networks
IET Cyber-systems and Robotics(2020)
摘要
Tomographic particle image velocimetry (Tomo-PIV) has been successfully applied in measuring three-dimensional (3D) flow field in recent years. Such technology highly relies on the reconstruction technique which provides the spatial particle distribution by using images from multiple cameras at different viewing angles. As the most popular reconstruction method, the multiplicative algebraic reconstruction technique (MART) has advantages in high computational speed and high accuracy for low particle seeding reconstruction. However, the accuracy is not satisfactory in the case of dense particle distributions to be reconstructed. To overcome this problem, a symmetric encode–decoder fully convolutional network is proposed in this paper to improve the reconstruction quality of MART. The input of the neural network is the particle field reconstructed by the MART approach, while the output is the regenerated image with the same resolution. Numerical evaluations indicate that those blurred or irregular particles can be significantly refined by the trained neural network. Most of the ghost particles can also be removed by this filtering method. The reconstruction accuracy can be improved by more than 10% without increasing the computational cost. Experimental evaluations indicate that the trained neural network can also provide similar satisfactory reconstruction and improved velocity fields.
更多查看译文
关键词
image reconstruction,tomography,biomimetics,velocimeters,velocity measurement,neural nets,cameras,flow visualisation
AI 理解论文
溯源树
样例
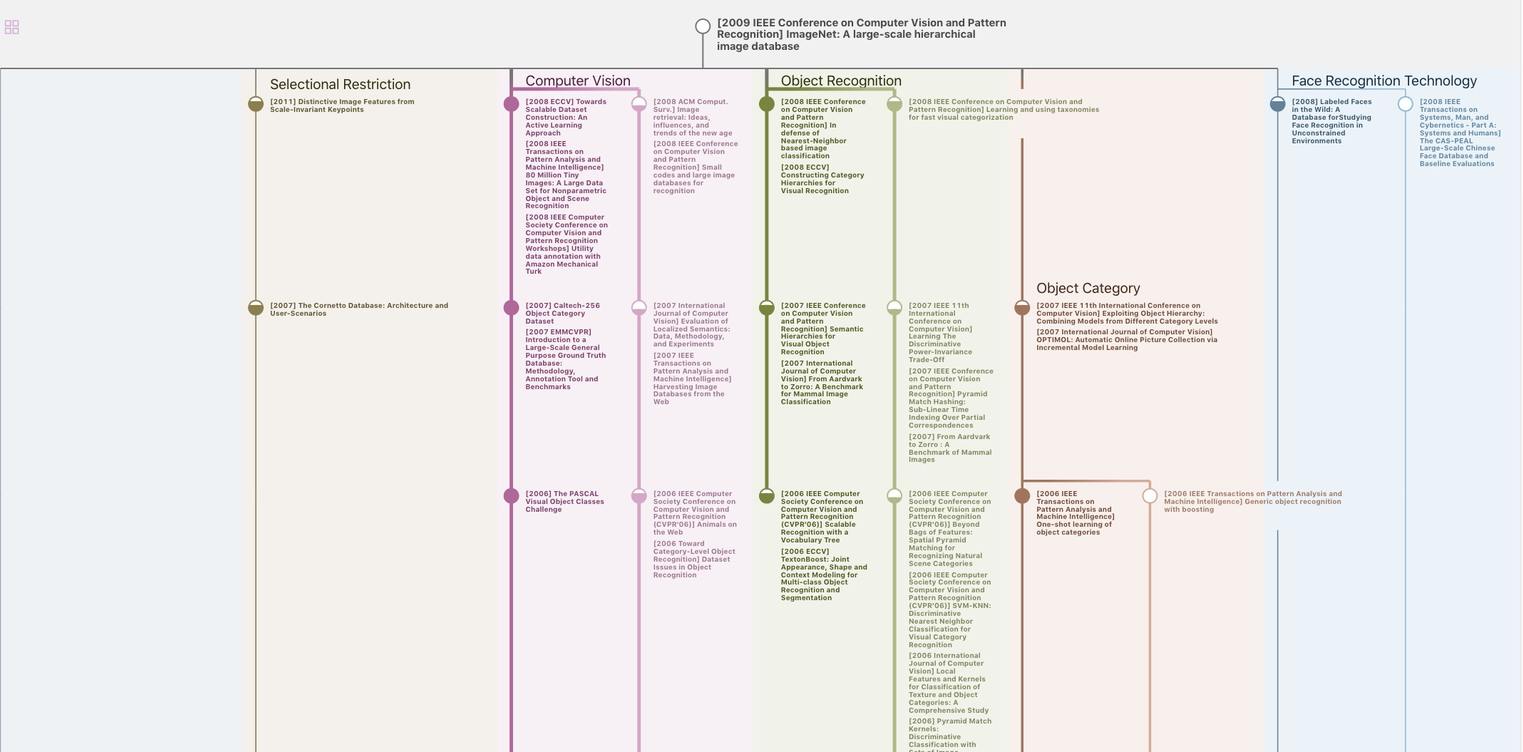
生成溯源树,研究论文发展脉络
Chat Paper
正在生成论文摘要