On the Feasibility of Automatic Malware Family Signature Generation
ASIA CCS '18: ACM Asia Conference on Computer and Communications Security Incheon Republic of Korea June, 2018(2018)
摘要
Malware detection has witnessed a rapid transition from manual signature release to fully automation in recent years. In particular, with the accumulation of huge malware sample sets, machine learning (ML) and deep learning (DL) have been proposed for verdict predicting and family attribution. Despite the high accuracy and efficiency, existing proposals fall short in providing explanation for their detection results. To fill in the gap between classification decisions and reasoning behind, we propose Galaxy, a generic approach for automatic malware family signature generation. Briefly, Galaxy selects meaningful metadata fields from static and dynamic analysis reports of the given samples. Based on the selected fields, all input samples will be clustered into groups according to similarity measurement. The observed similarities will then be converted into patterns and validated against multiple intelligence sources to decide whether it is suitable for malware detection. In the end, Galaxy launches a refine process to improve the grouping results and increase sample coverage. We have applied the Galaxy framework on daily incoming Android samples to our WildFire production since September 2016. Up to know, Galaxy has generated more than 12,500 unique family signatures covering a total of 1.75 million Android malwares. Those family signatures have provided valuable insights for the discovery of undocumented malicious domains and identification of Communication & Control (C&C) servers. Because of our rigid quality requirement, all released signatures have been proven to cause no false positives in production.
更多查看译文
关键词
Galaxy, Malware Categorization, Malware Detection, Clustering, Machine Learning, Intelligence, Android
AI 理解论文
溯源树
样例
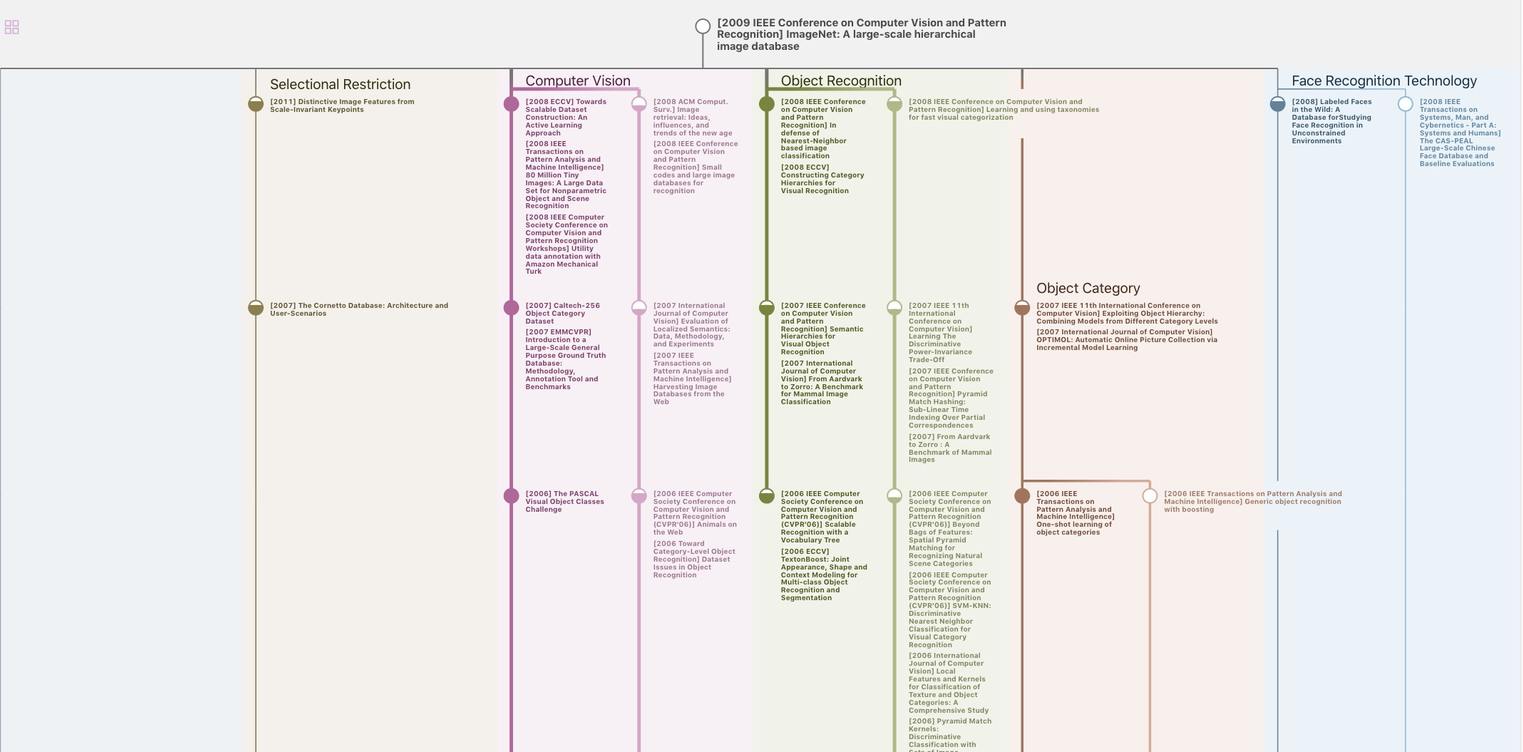
生成溯源树,研究论文发展脉络
Chat Paper
正在生成论文摘要