Passenger Flow Prediction in Traffic System Based on Deep Neural Networks and Transfer Learning Method
2019 4th International Conference on Intelligent Transportation Engineering (ICITE)(2019)
摘要
Nowadays, many problems have arisen during the rapid development of our traffic systems, such as crowd congestion, traffic accident, imbalance use of infrastructures, etc. By predicting passenger behaviors in advance, we can solve these problems to a large extent. However, the task is not easy. Because modern traffic is a large, high-dimensional and mutual-correlation system, we face many difficulties such as complexity, nonlinearity, data insufficiency, etc. In this paper, we proposed a method called DTLTP (Deep Transfer Learning for Traffic Prediction) which can deal with the encountered challenges effectively. The model captures latent characters and relations of space and time by using embedding learning method. Also, we adopt hybrid deep neural networks which have flexible structure and strong learning ability to deal with nonlinearity of the task. Furthermore, the model applies transfer learning technique which can take full use of related data to help the training of target task. Finally, we evaluate the performance of our model from multiple perspectives, showing that DTLTP is a promising and excellent approach for traffic flow prediction.
更多查看译文
关键词
traffic prediction,deep learning,transfer learning,machine learning,intelligent transportation system
AI 理解论文
溯源树
样例
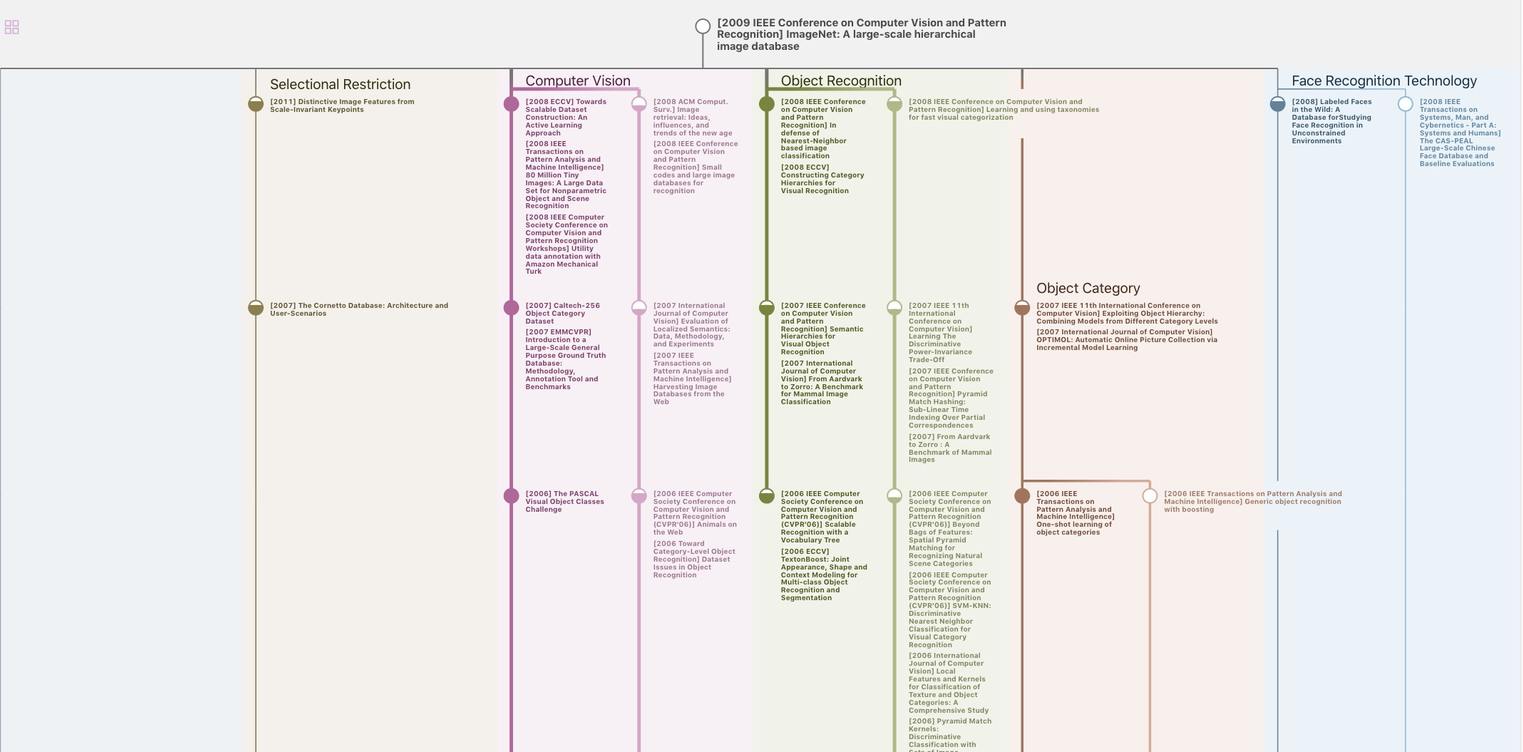
生成溯源树,研究论文发展脉络
Chat Paper
正在生成论文摘要