HL-Pow - A Learning-Based Power Modeling Framework for High-Level Synthesis.
ASP-DAC(2020)
摘要
High-level synthesis (HLS) enables designers to customize hardware designs efficiently. However, it is still challenging to foresee the correlation between power consumption and HLS-based applications at an early design stage. To overcome this problem, we introduce HL-Pow, a power modeling framework for FPGA HLS based on state-of-the-art machine learning techniques. HL-Pow incorporates an automated feature construction flow to efficiently identify and extract features that exert a major influence on power consumption, simply based upon HLS results, and a modeling flow that can build an accurate and generic power model applicable to a variety of designs with HLS. By using HL-Pow, the power evaluation process for FPGA designs can be significantly expedited because the power inference of HL-Pow is established on HLS instead of the time-consuming register-transfer level (RTL) implementation flow. Experimental results demonstrate that HL-Pow can achieve accurate power modeling that is only 4.67% (24.02 mW) away from onboard power measurement. To further facilitate power-oriented optimizations, we describe a novel design space exploration (DSE) algorithm built on top of HL-Pow to trade off between latency and power consumption. This algorithm can reach a close approximation of the real Pareto frontier while only requiring running HLS flow for 20% of design points in the entire design space.
更多查看译文
关键词
time-consuming register-transfer level implementation flow,HL-Pow,onboard power measurement,power-oriented optimizations,power consumption,running HLS flow,learning-based power modeling framework,high-level synthesis,HLS-based applications,accurate power model,generic power model,power evaluation process,power inference,FPGA HLS,machine learning,automated feature construction flow,feature extraction,design space exploration,Pareto frontier,power 24.02 mW
AI 理解论文
溯源树
样例
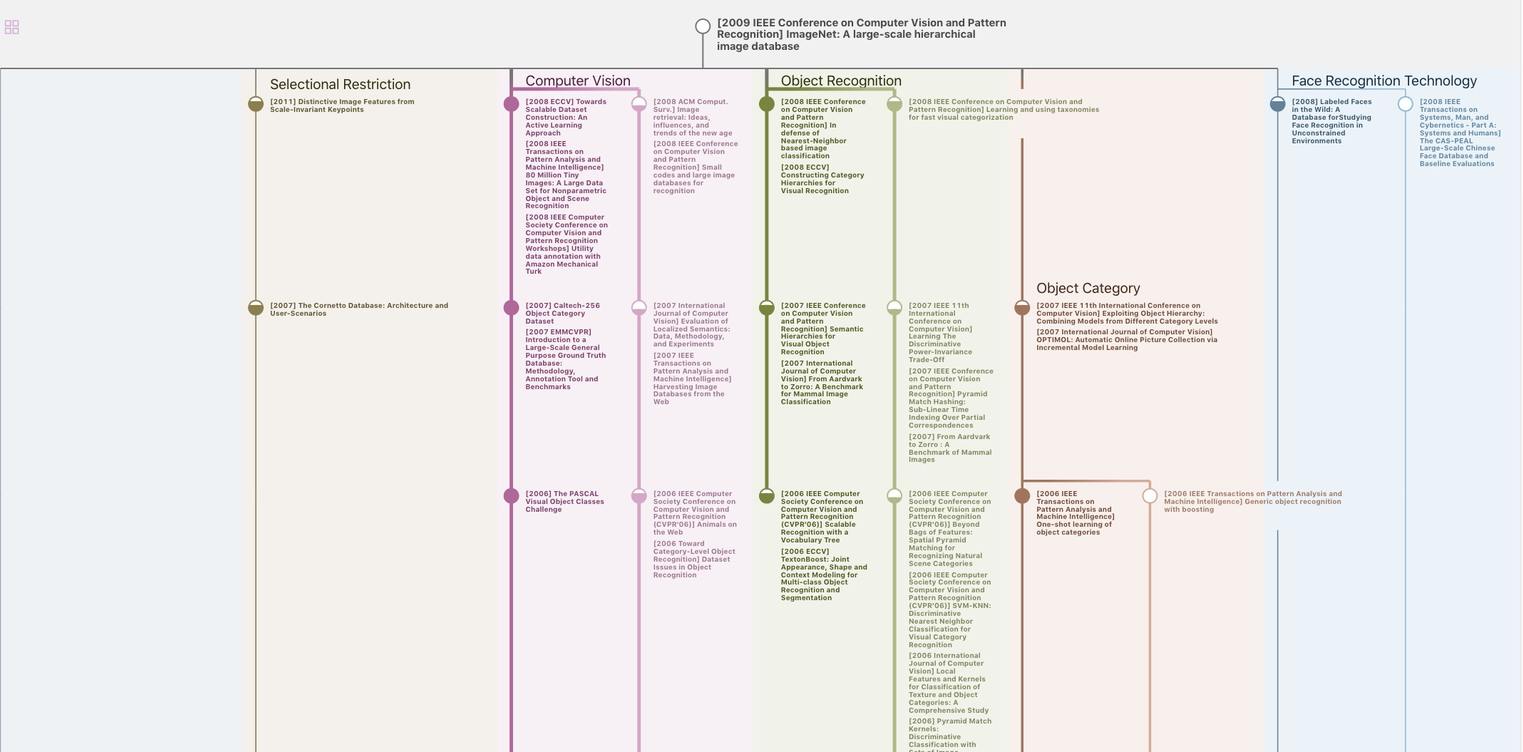
生成溯源树,研究论文发展脉络
Chat Paper
正在生成论文摘要