Enhancing Top-Down Proteomics Data Analysis by Combining Deconvolution Results through a Machine Learning Strategy.
Journal of the American Society for Mass Spectrometry(2020)
摘要
Top-down mass spectrometry (MS) is a powerful tool for the identification and comprehensive characterization of proteoforms arising from alternative splicing, sequence variation, and post-translational modifications. However, the complex data set generated from top-down MS experiments requires multiple sequential data processing steps to successfully interpret the data for identifying and characterizing proteoforms. One critical step is the deconvolution of the complex isotopic distribution that arises from naturally occurring isotopes. Multiple algorithms are currently available to deconvolute top-down mass spectra, resulting in different deconvoluted peak lists with varied accuracy compared to true positive annotations. In this study, we have designed a machine learning strategy that can process and combine the peak lists from different deconvolution results. By optimizing clustering results, deconvolution results from THRASH, TopFD, MS-Deconv, and SNAP algorithms were combined into consensus peak lists at various thresholds using either a simple voting ensemble method or a random forest machine learning algorithm. For the random forest algorithm, which had better predictive performance, the consensus peak lists on average could achieve a recall value (true positive rate) of 0.60 and a precision value (positive predictive value) of 0.78. It outperforms the single best algorithm, which achieved a recall value of only 0.47 and a precision value of 0.58. This machine learning strategy enhanced the accuracy and confidence in protein identification during database searches by accelerating the detection of true positive peaks while filtering out false positive peaks. Thus, this method shows promise in enhancing proteoform identification and characterization for high-throughput data analysis in top-down proteomics.
更多查看译文
AI 理解论文
溯源树
样例
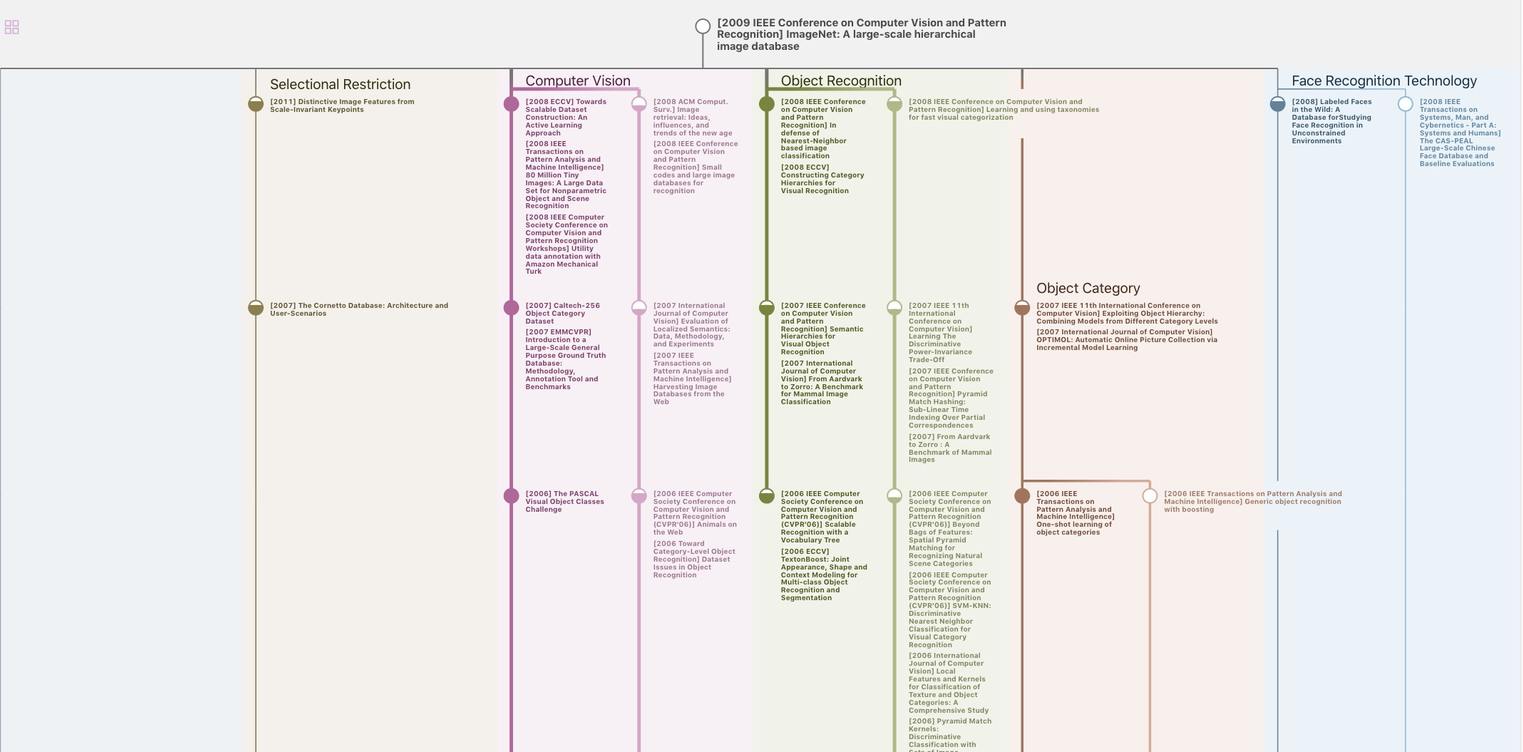
生成溯源树,研究论文发展脉络
Chat Paper
正在生成论文摘要