A Hybrid FeMFET-CMOS Analog Synapse Circuit for Neural Network Training and Inference
ISCAS(2020)
摘要
An analog synapse circuit based on ferroelectric-metal field-effect transistors is proposed, that offers 6-bit weight precision. The circuit is comprised of volatile least significant bits (LSBs) used solely during training, and non-volatile most significant bits (MSBs) used for both training and inference. The design works at a 1.8V logic-compatible voltage, provides 10^10 endurance cycles, and requires only 250ps update pulses. A variant of LeNet trained with the proposed synapse achieves 98.2% accuracy on MNIST, which is only 0.4% lower than an ideal implementation of the same network with the same bit precision. Furthermore, the proposed synapse offers improvements of up to 26% in area, 44.8% in leakage power, 16.7% in LSB update pulse duration, and two orders of magnitude in endurance cycles, when compared to state-of-the-art hybrid synaptic circuits. Our proposed synapse can be extended to an 8-bit design, enabling a VGG-like network to achieve 88.8% accuracy on CIFAR-10 (only 0.8% lower than an ideal implementation of the same network).
更多查看译文
关键词
LSB update pulse duration,CIFAR-10,hybrid FeMFET-CMOS analog synapse circuit,neural network training,ferroelectric-metal field-effect transistors,volatile least significant bits,nonvolatile most significant bits,hybrid synaptic circuits,voltage 1.8 V
AI 理解论文
溯源树
样例
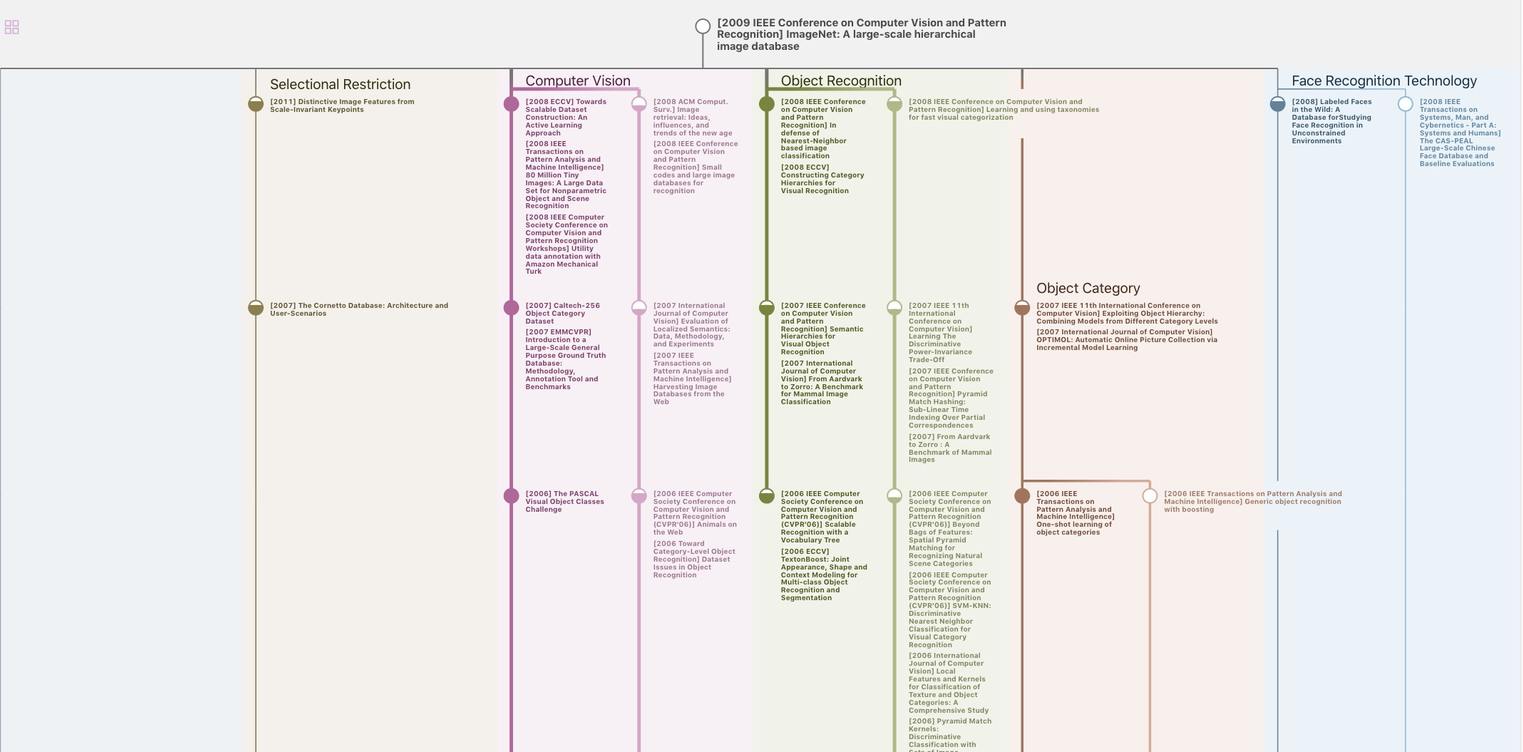
生成溯源树,研究论文发展脉络
Chat Paper
正在生成论文摘要